Beyond Cortana: What artificial intelligence means for the future of Microsoft

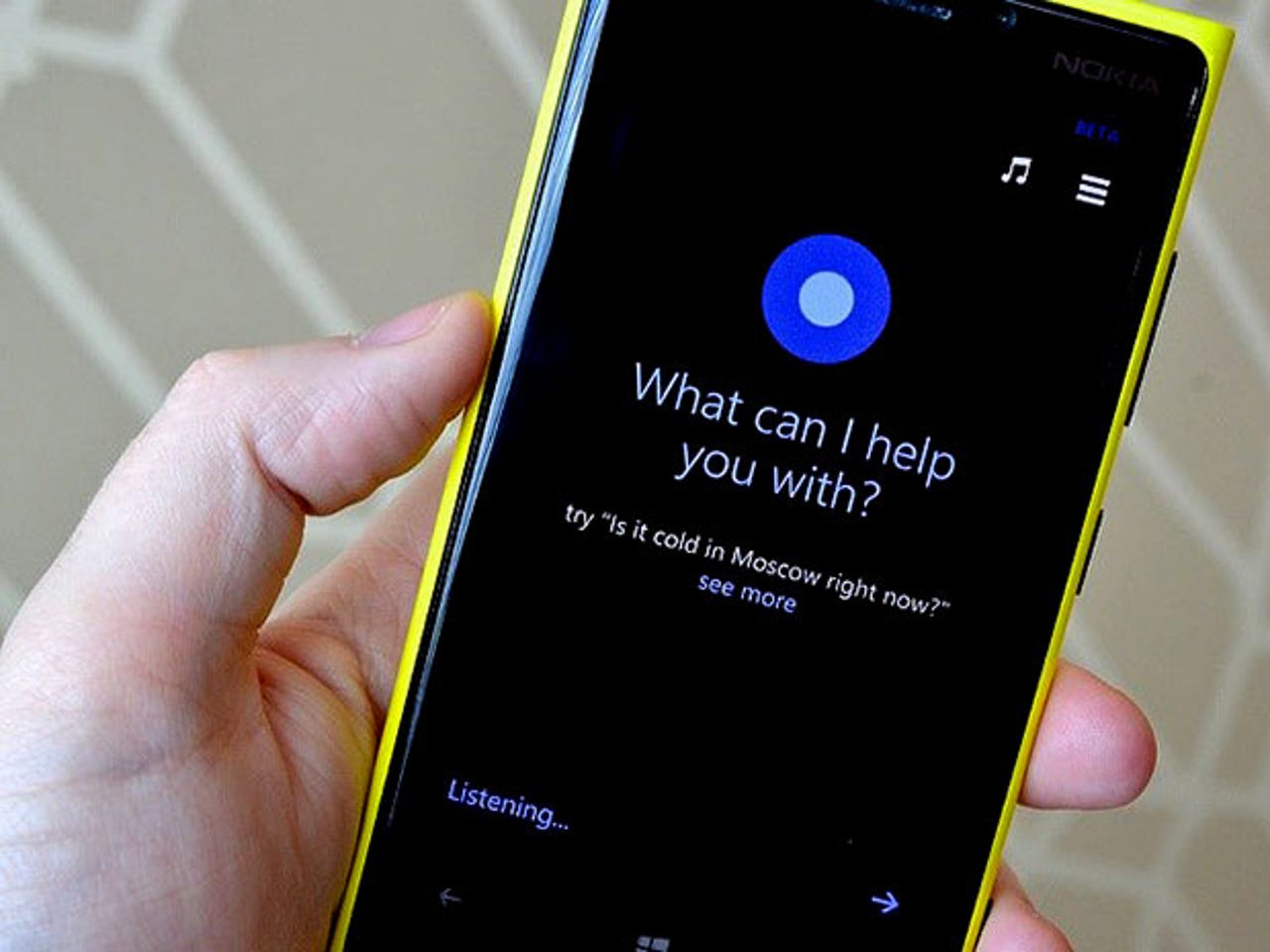
While Satya Nadella might claim to not know how Microsoft's new Skype translator technology works, the AI research teams at Microsoft Research have a pretty good idea: the neural nets they use have come close to showing that the deep grammar theories of linguists like Noam Chomsky are the basis of how we communicate. But that's only part of the story of how MSR's AI research is powering the new Microsoft.
At the recent Future in Review conference, the head of Microsoft Research (MSR), Peter Lee, discussed the future of personal assistant technologies and the current state of practical artificial intelligence. His thoughts provide some interesting insight into the importance of AI to Microsoft, and why Bing is such a core technology for its future products.
Tech Pro Research: Security
The AI work is the basis of the contextual ambient intelligence that's at the heart of Nadella's mobile and cloud vision for Microsoft — and it's through tools like the Skype translator and Windows Phone's Cortana we're seeing just how MSR's research AIs are becoming products.
Lee's vision is one of AI helping humans, so it's perhaps not surprising that the face of Microsoft's AI is Cortana, a virtual personal assistant. It's not the endpoint, it's as Lee says: "[A] part of the evolution of AI, showing what it can be."
That's why MSR is deeply involved in the development of what's at heart a consumer product. While much of MSR's work is blue sky, looking at the future of computing, it's also part of many of Microsoft's cloud scale projects, such as Bing and Cortana.
Cortana is, at heart, a user experience for an artificial intelligence, as Lee says. "What the user sees is a UI that's intended to be like a personal assistant, built using the basic building blocks for natural interaction," he says.
It's the AI behind it that's handling much of what it does — or rather, a series of different neural networks and rules engines that are the closest to AI we have today. They're designed to make inferences from your personal data, to be prescient (not creepy). It's here that Nadella's ambient intelligence comes in to play, As Lee asks: "Can we mine all the data we have access to, and then extract intelligence?"
Cortana just one of many user experiences for this type of AI research, as Lee's researchers work on. "Knocking down 20 to 30 year old problems in AI, understanding intent," he says. The aim is to produce systems that can see, hear and understand, by focusing on correlations in large amounts of data.
Scale is important here, as the more data the better, but it's essential to be careful that the resulting rules and neural nets don't lead to incorrect correlations. For one thing, it's easy for neural nets to fall into the fallacy of correlation implying causation. We know that people on the streets with umbrellas don't cause rain, but it's hard for a neural net to make that distinction.
A big part of MSR's AI research is around understanding what causes what, the process that AI scientists call causal inference. It's a complex problem, and it's why despite its conversational Chit-Chat rules engine, understanding things about situations — situational inference — is still beyond Cortana. Though while commercialised AI may not be there yet, research projects are getting close. In MSR's Redmond offices, Building 99, there's a robot receptionist that aims to understand when two people are talking to each other.
Lee told his audience about another live experimental AI in Building 99. As you walk to the elevators, the doors open before you get there. The lifts are controlled by yet another neural net, this one trained on the behaviour of people in the hall near the lifts. Here cameras watched people in the building's atrium for months.
The neural net correlated the behaviours of people going to the elevator, a problem complicated by the fact that the elevators were directly on the route to the building's restaurant and that the open space of the atrium was used for impromptu meetings. Over the months of watching, the neural net learnt to understand the intent of people in the atrium, building a model of their actions and comparing the model to their actual behaviour. Once the system had enough confidence in its intent model, it automatically switched over to controlling the lift.
What had evolved in the AI's neural net was something new, something that couldn't be developed manually: there were too many variables, too many paths individuals could take through the atrium. The system had needed to develop positional awareness, and phase out undesired stimulus.
That's part of what's interesting about the neural nets that power Cortana's speech recognition, and the Bing translation tools — and now the Skype real-time translator. They're things we don't really understand, but that do exhibit behaviours that tell us something new about the way the world works.
Speech recognition and real-time transcription have been lab-grade technologies for some time. They work well in limited circumstances, but need engineering before they're released in the outside world — especially considering the wide range of contexts and environments they work in.
When Satya Nadella talks about not understanding just how the translation neural nets work, he's being accurate — but only in a limited way. MSR has been investigating what it calls "transfer learning" for some time now. With hyperscale systems and lots of English sources it's possible to get past what was known as the "over-fitting problem" where too much data made neural nets unreliable.
With MSR's current generation of neural nets you can just keep training with more data, and the results get better. Where things get interesting, is when the same neural net is trained with Chinese as well: not only does it learn Chinese, but its English performance improves. Training the same net with French means it learns French faster, and both English and Chinese recognition get better.
This where transfer learning comes in: the neural net for one language makes it easier to generate the net for another. The effect isn't just being found in MSR's AIs; other researchers are seeing the same thing. Lee notes that this consistent effect is a matter of the net's lower layers "discovering the structures of human language". It's a fascinating set of discoveries, and as Lee says: "This could have big implications for understanding human discourse. I can't overstate the excitement in the field."
Has AI unraveled one of the longest running debates in modern linguistics? In the 1950s, when Noam Chomsky suggested that all languages were based on common deep structures it sparked a series of arguments that have run for much of the last half-century. But now with neural nets like those that power Skype's translator, we're starting to see a deep statistical linkage between related terms, terms that might only be linked by gender relationships.
The more data we have, the better those translations get. We're seeing that with Bing's automated translations of Twitter. As Lee says, some things, like humour, are hard. But the result is a treasure trove of data that's making our phones better assistants, and that's starting to break down the barriers of language.
This then is the value of MSR's blue-sky, large-scale research, and of the massive collection of data at the heart of Bing. It might take years to get results, but when it does it can do some rather world-changing things with the devices on our desks, and in our pockets. It's the engine that drives Nadella's ambient intelligence, and the future of Microsoft.
Further reading