Can a database of radiation therapy dosages help cancer patients?
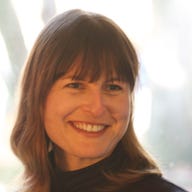
Radiation therapy involves precisely targeted x-ray beams used to destroy cancerous tumors. But it’s far from a precise science. Dr. Todd McNutt, assistant professor of radiation oncology at Johns Hopkins University, explained that different physicians will approach radiation differently, based on what they are willing to compromise in radiating the target to spare nearby organs and tissues (called critical structures). “That’s part of the art and how different physicians see things,” he said.
But now, oncologists at Hopkins have a new, data-driven tool to make radiation treatment more exact. McNutt, director of clinical informatics for the school’s Department of Radiation Oncology and Molecular Radiation Sciences, has worked with his team to build an analytical database that pulls together therapy data and allows providers to look at the best and the worst outcomes. This enables them to create an optimal radiation dosage treatment plan.
Called the Frankenstein method, since it’s combining data from many different former patients, McNutt said the system will improve clinical care faster than traditional clinical trials. We spoke last week.
OK, I’ve to got ask: What’s behind this name, the Frankenstein method? And how do patients feel about it?
The whole story revolves around how we look at information about prior patients and use the data from their treatments to influence both the quality and efficiency of taking care of new patients. So how we make the best use of past experiences.
Today we do that mostly based on the physician’s experience. How do you take a computer system and store that prior experience so you can retrieve it and improve medicine?
In radiotherapy, we have linear accelerators that deliver the radiation. Where the beams intersect we have a very high dose of radiation, but it’s impossible to bring the beam in and not treat everything in its path. So our goal is to identify target areas and critical structures –kidney, bladder, muscle tissue--anywhere we want to limit the amount of radiation.
As you can image, every patient is different, so they have different shapes of the structures. So the best example is head and neck. We would target the primary tumor and prophylactically treat the lymphatic system around it where there is a high likelihood of metastatic disease. At the same time, we have 13 critical structures that we want to avoid--including the parotid glands (responsible for salivary function), mandible, spinal cord, brain stem, larynx etc. But how close is the parotid gland to the target? If they get too much radiation dose, [the patient] might spend the rest of their life with xerostomia, or dry mouth.
So we have a database of prior patient shapes of target volumes and critical structures and the relationships between the two. For new patients we can look into the database and find all parotid glands from all prior patients. Of all those, we look at what was the best dose distribution, and that gives us guidance on how good of sparing we should be able to do for the patient.
So the Frankenstein comes in in that we might find the parotid gland from Patient 22 and the spinal cord from Patient 71. So we’re getting the best dose from all the structures from many different patients, and in the end, we’re finding the dose distribution we should expect to be able to achieve.
How long did it take you to create this?
We’ve been working on this for three years. A lot of it’s the data collection.
So the more data your collect the more accuracy you can achieve with the dosage.
Our ability to predict how well we can do is only as good as the data in our database. We want to improve our database because we want it to represent what we really can achieve.
Does this work best for certain types of cancer?
It’s helpful for radiation therapy, which is targeted. So it applies to cancers like prostate, pancreas, breast. We haven’t tried it on brain. We started to work on the thoracic region (lung cancer). We did a lot on the head and neck.
The [treatment plan] also depends on the critical structure. Something like the spinal cord is a serial structure—if I radiate one small part of it I kill it and I can paralyze the person from that point down. So I don’t want any part to receive too much radiation. On the other hand, the parotid gland or lung, I can radiate one-third of it and kill the tissue and it can still function, but maybe at less capacity. So it’s important to know if it’s a serial structure or a parallel structure, like a kidney or a lung.
Tell me about the technology you are using to collect the data.
We characterize the complex relationship between the critical structures and the target in a overlap volume histogram. It basically tells us how far of a percentage the critical structure is away from the target. Knowing this allows us to find all cases in the database where that portion of the parotid is closer to the target, and find the lowest dose delivered as our prediction of best achievable dose. Thus helping us know how good a plan we should be able to generate.
What’s the system you use to do this?
It’s a treatment planning system for intensity modulated radiation therapy called Pinnacle3, which is now a product of Phillips. I wrote a good portion of that software when I was at Phillips.
How much have you used this with patients?
We have used it for a blind study and a few patients over the last year and a half. My new post-doc’s job is to deploy it more in the clinical process.
Do you explain to patients how you’re using this data?
At this point we haven’t. For now we view it as a means to insure quality in plans.
It seems like once the data is collected, it could be used by doctors at any institution in the same way that you’re using it.
To put it at other institutions, we’d need them to build their own databases. One of the things we want to do is compare the planning at different institutions. You’ll see there are differences in the positions and how much they are willing to compromise to spare a critical structure. Right now that’s part of the art and how different physicians see things.
We’re starting to share data with MD Anderson [Cancer Center, at the University of Texas], and it’s clear they’re willing to compromise more of the target to spare the parotid gland. And that becomes clinical judgment. So that’s something we have to address and figure out. In the same database, we also have toxicity scores and do follow-ups on the patients. So we’ll also align different institutions with toxicities showing, for example, if you go down that route you have a higher rate of toxicity like voice change or something like that.
So you’ve got something you’re calling an “art” and the level of compromise is a somewhat subjective thing. Yet now numbers are being attached to it, and some day there may be standards using this data to make the procedures more uniform?
The only way we’ll ever find out is getting the data organized so you can run true comparisons. It’s the only way to solve it. It’ll take a lot more data collection and data sharing. I have a 20-year career left, and someday maybe there will be more funding for it. We’re still working on trying to get some federal funding. I don’t know that they don’t like the idea; I just think they don’t have a lot of funding. For me, it’s a question of whether I want to spend more time writing grants or doing the interesting work.
This is part of your bigger program called OncoSpace. What’s the idea behind the overall program?
Oncospace is our database and website access to it. The idea is to allow for the storage of a lot of radiation therapy data for analysis and use that for decision support. That’s sort of the theme of it. The treatment plan quality is one nice example with very complex data of how we can take advantage of that.
We do this now for clinical trials, and that’s how we learn from prior patients. Then, eventually, someone changes their practice because of what someone is finding. But it’s very inefficient, and it’s myopic in that it’s very controlled because patients fall off those trials and it’s just those two groups.
So that’s not to say the clinical trials shouldn’t happen, but we should also be capturing our clinical data from regular patients and learning from that, just to see if we’re doing as well as we should be. The everyday patients are not followed as closely and rigorously as they could be. Only about 3 percent of radiation therapy patients are on a clinical trial. So we’re throwing away 97 percent of data.
The cool thing is that now we have a real example that can improve therapy. We’re showing we can get a better dose distribution and improve the plans significantly.
This post was originally published on Smartplanet.com