China pumps up the hype about A.I. with oddball computer chip
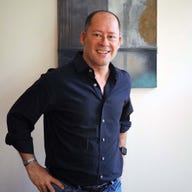
The term "artificial general intelligence," or AGI, doesn't actually refer to anything, at this point, it is merely a placeholder, a kind of Rorschach Test for people to fill the void with whatever notions they have of what it would mean for a machine to "think" like a person.
Despite that fact, or perhaps because of it, AGI is an ideal marketing term to attach to a lot of efforts in machine learning. Case in point, a research paper featured on the cover of this week's Nature magazine about a new kind of computer chip developed by researchers at China's Tsinghua University that could "accelerate the development of AGI," they claim.
The chip is a strange hybrid of approaches, and is intriguing, but the work leaves unanswered many questions about how it's made, and how it achieves what researchers claim of it. And some longtime chip observers doubt the impact will be as great as suggested.
"This paper is an example of the good work that China is doing in AI," says Linley Gwennap, longtime chip-industry observer and principal analyst with chip analysis firm The Linley Group. "But this particular idea isn't going to take over the world."
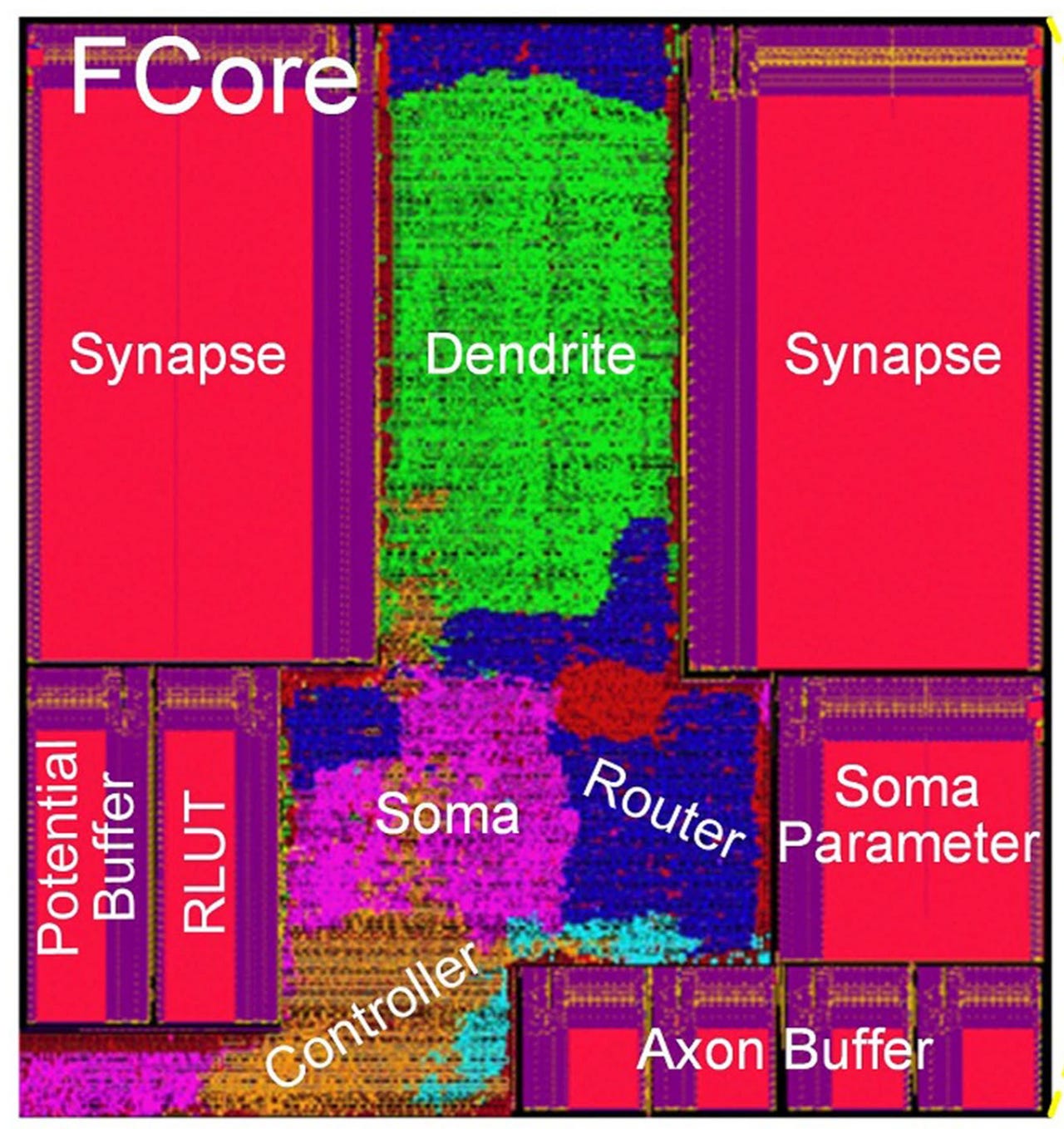
The layout of an "FCore" computing core in the Tianjic chip by China's Tsinghua University scholars.
The premise of the paper, "Towards artificial general intelligence with hybrid Tianjic chip architecture," is that to achieve AGI, computer chips need to change. That's an idea supported by fervent activity these days in the land of computer chips, with lots of new chip designs being proposed specifically for machine learning.
The Tsinghua authors specifically propose that the mainstream machine learning of today needs to be merged in the same chip with what's called "neuromorphic computing." Neuromorphic computing, first conceived by Caltech professor Carver Mead in the early '80s, has been an obsession for firms including IBM for years, with little practical result.
Neuromorphic chips transform incoming data by "spiking" in electrical potential, whereas today's deep learning performs a "matrix multiplication" operation that transforms incoming data via a set of "weights" or parameters, and then passes them through a non-linear operation called an "activation." The two different paths of the data are incommensurable, which is why they have been pursued as separate technologies to date.
Comparison of data flows for traditional deep learning and the neuromophic approach to computing, which the authors have sought to fuse into one chip.
The Chinese researchers, led by Jing Pei of Tsinghua's Center for Brain-Inspired Computing Research, claim their "Tianjic" chip has successfully "fused" the two approaches. Back in 2015, Pei and colleagues developed a strictly neuromorphic computer. This time, they've combined some of the aspects of that chip with the deep learning matrix multiplication. The Tianjic chip has numerous "cores" that operate in parallel, and each one can perform either the spikes of the neuromorphic approach or the matrix operations of the deep learning approach.
The crux is that Pei and colleagues have "aligned the model data flow," meaning, they organized which operations of the deep learning calculation correspond to which in the neurmorphic ones, so that the cores can flip back and forth between the two.
The paper has provocative terms for the various areas of the chip cores, borrowed from the biology of the brain, such as "axon," "soma," "dendrite" and "synapse."
A self-driving bicycle design, with the Tianjic chip attached to its rear.
To demonstrate what they can do, they programmed the chip to perform a number of real-world tasks such as identifying objects and determining a course to move through a terrain of objects. They have demonstrated it by attaching the chip to a bicycle with no rider that is able to move on its own through its environment, responding to voice cues from its master. You can see the demo in a video accompanying the article, and posted on YouTube.
The bicycle moves by computing a number of things with the different neuromorphic or deep learning networks, "including a CNN for image processing and object detection, a CANN [continuous attractor neural network] for human target tracking, an SNN [spiking neural network] for voice-command recognition, and an MLP [multi-layer perceptron] for attitude balance and direction control."
Also: Chip world tries to come to grips with promise and peril of AI
The stunt was picked up this week by the media, big time, with various headlines playing upon the feat with quips such as, "look, no hands," regarding the autonomous bike. The New York Times ran with "And now a bicycle built for no one."
The problem is, there are a number of strange omissions here. Start with the notion of AGI: nowhere is a really sharp definition provided, much less a theoretical suggestion that combining functions is somehow an approach to AGI. The implication is that having one chip do a bunch of things is somehow a more "general" kind of chip. As the authors write, "An AGI system […] requires a general platform" that supports lots of processing "in a unified architecture." That sounds like consolidation and integration, not necessarily like intelligence.
For another thing, there is very little information about how the two types of networks, neural and neuromorphic, are trained, which is an important issue for either one separately, and even more important when they're combined. Pei and colleagues write, in the "Methods" section of the paper, that they trained the deep learning part in the normal way, and that for the neuromorphic part, they relied on a method introduced last year by some of the researchers, called "Spatio-temporal backpropagation," a version of the backpropogation approach common in deep learning.
There are also some missing details about the chip's fabrication. For example, the part is said to have "reconfigurable" circuits, but how the circuits are to be reconfigured is never specified. It could be so-called "field programmable gate array," or FPGA, technology or something else. Code for the project is not provided by the authors as it often is for such research; the authors offer to provide the code "on reasonable request."
More important is the fact the chip may have a hard time stacking up to a lot of competing chips out there, says analyst Gwennap. The specs seem underwhelming, in his view. "Tianjic's reported 1.28 TOPS/watt [trillions of operations per watt, a common measure of performance] is similar to today's GPUs," he notes, referring to graphics processing unit chips made by Nvidia and Advanced Micro Devices. However, the performance is "well behind more advanced architectures" of newer chips, he notes.
Also: AI is changing the entire nature of compute
Gwennap's colleague, Mike Demler, concurs. He notes some inaccuracies in the paper, such as the contention that spiking neurons require "extra high-precision memory" circuits for some functions. Demler's review of a neuromorphic chip by chip giant Intel, called "Loihi," shows that such is not the case. A chip developed by startup Brainchip, Inc., also proves the claim false, he says. Moreover, since the Loihi chip has already shown that conventional neural networks, such as a convolutional neural network, or CNN, can be implemented as a spiking neuron, there's no need for the kind of "unified" chip that the Tsinghua authors claim.
To Gwennap, it is a bit of an odd goal to try and combine two things in one. "What the paper calls ANN and SNN are two very different means of solving similar problems, kind of like rotating (helicopter) and fixed wing (airplane) are for aviation," says Gwennap. "Ultimately, I expect ANN and SNN to serve different end applications, but I don't see a need to combine them in a single chip; you just end up with a chip that is OK for two things but not great for anything."
But you also end up generating a lot of buzz, and given the tension between the U.S. and China over all things tech, and especially A.I., the notion China is stealing a march on the U.S. in artificial general intelligence — whatever that may be — is a summer sizzler of a headline.