Cloud apps, big data and the wisdom of swarms
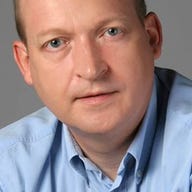
It was only after my wife acquired an iPhone 4S this week that I fully understood the importance of big data for SaaS vendors. Did you need to train Siri to recognise your voice, I wondered? What I found from a brief Internet search was a revelation. I'm old enough to remember the PC-based voice recognition systems of the late 1990s from the likes of IBM and Dragon Software. Those systems had to be trained over a period of a week or more to recognise the sound of the user's voice. Siri doesn't do that. Instead, it matches the voice it hears to a library of voice patterns and uses the closest match to interpret what you say.
What's happening behind the scenes is that Siri has analyzed tens of thousands of voices and identified patterns that run across all of those voices. As a cloud-sourced app, it can continue to refine and hone that central library of voice patterns based on what it encounters in the field. This is where the cloud approach really wins. The old, PC-based systems could learn a person's voice, but they couldn't use that learning to improve their ability to learn the next user's. A cloud app like Siri can continuously evolve its core capability with every new user.
So instead of having to perfectly predict every anticipated type of voice (and inevitably fail on the unexpected edge cases), the cloud-based app can simply react to what it finds. As my wife started using the phone on a family car journey, Siri dealt effortlessly with the mixed voices of my wife and two excited children. If you'd set out to develop a voice-recognition system, would you have thought to include that use case in the spec? Siri doesn't have to make that judgement — in fact, Siri doesn't judge at all, it just works with what it finds. That, along with the broad base of data that it gets to work with, is what makes it so powerful.
This is what makes big data so important for SaaS vendors. It's not simply the ability to analyse huge pools of data. What really matters is the broad base of that data, gathered from a large mix of users within which patterns of behavior can be analysed and then applied elsewhere. Think of it as swarm data — lots of individual, autonomous behaviors that collectively add up to reusable patterns.
A few weeks ago, I wrote about cloud collaboration vendor Huddle's new file synchronization capability. This uses analysis of prior behavior across its user base to decide which shared files to download to a user's local device, and then continues learning from behavior patterns among the user and their colleagues to make its predictive downloading more and more accurate. Like Siri, the historic analysis of its existing broad base of user behavior gives it a head start in delivering accurate results from the get-go.
SaaS vendors are in a unique position because of the collective behaviorial data they're able to amass. For the past year, email provider Mailchimp has employed a data scientist on what it calls its Email Genome Project, looking for patterns in the millions of emails and campaigns its customers generate. It's been instrumental in finding and shutting down malicious email accounts, as well as generating benchmark stats that customers can use to evaluate their performance. These are useful advances, but what I'd really like to see next is to have those benchmarks brought into the app to evaluate mailings while they're being created.
I know that many SaaS vendors think of big data as a mine of potentially useful information but are uncertain where they are most likely to unlock significant value. To my mind, it's the behavioral data that holds the most promise because, in analyzing how their swarms of users behave, they can discover new ways to automate common patterns of behavior. Those reusable patterns that can shortcut a learning process and deliver faster results are going to be like gold dust for those who are first to surface them.