Cloudera's Hilary Mason: To make AI useful, make it more "boring"
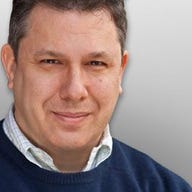
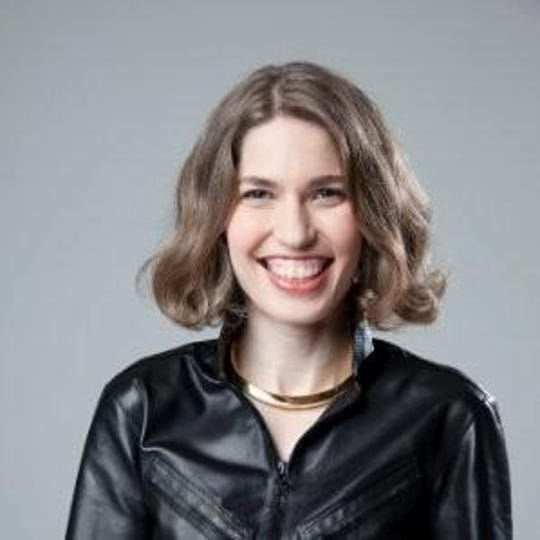
Cloudera's GM of Machine Learning, Hilary Mason
When it comes to Artificial Intelligence, the industry is at a crossroads of fascination versus function. We're awed by the technology, but a number of forces are conspiring to minimize the progress we're making, especially in the Enterprise. There are a few people out there who are adamantly trying to address this. One of them is Hilary Mason, Cloudera's GM of Machine Learning.
Mason was previously Chief Data Scientist at Bitly, then founder and CEO of Fast Forward Labs, which Cloudera acquired in 2017. While Fast Forward still exists as a unit of Cloudera and is doing very important work, Mason's role puts her at the head of AI at Cloudera overall. That's a powerful place to be, for a couple of reasons. One is that Cloudera's recent merger with Hortonworks makes it an undisputed leader in the data lake/Hadoop/Spark/analytics world. Perhaps more important, though, is that AI is one of the company's three core focuses (the other two being the Edge and Data Warehousing).
Executive opinion
With that seat of power and the experience that comes from her current and previous roles, Mason's brain is one of the best to pick, in order to get a sense of how applied AI is going, as a commercial effort. I had the chance recently to talk to Mason and do just that. And what I found out is, while Mason is genuinely optimistic and excited about AI, her view is that, as an industry, we could be doing better.
Mason says people are paying attention to the wrong things. She believes strongly that we are focusing almost exclusively on the technology of AI and, as a result, we're failing to focus on what AI can actually give us. In general, Mason says we need to focus on "boring" business problems, like coupons and anti-money laundering, and less on "cool" things, like robots that talk to us.
Two camps
More specifically, Mason describes a somewhat alarming segregation in the field.
On the one hand, the AI world has its researchers, who are doing groundbreaking, innovative work, but often in the vacuum of academia. Researchers often aren't collaborating with industry enough, and often work with a stable of pedagogically common data sets that don't always translate well to business problems. As such, much AI research work is cloistered away. This deprives Enterprise organizations of researchers' innovative advances, and the lack of a broad variety of data coming from real-world industrial problems also hampers the progress researchers can make, even in that cloistered domain.
Industry, on the other hand, has its own problems. So enamored of AI, Enterprise organizations often take a spend-first-plan-later approach. And, unable to bootstrap the AI work on their own, they may also engage startups to do the work for them, many of which aren't especially stable or well-funded. The combination leads to unacceptably high project failure rates and, even for projects deemed successful, results that often fall short of potential.
Minding the gap
FastForward Lab's (FF) mission is to help bridge this academic-industrial gap and the performance deficit of each side. One way FF does this is by acting as consultants who advise business customers on whether their data is of sufficient quality to go forward and build ML or deep learning models. If the data does offer sufficient integrity and efficacy, then FF can help apply cutting edge AI from academia, to industry. FF also publishes academic-caliber reports that result from conversations with researchers and the building of prototypes that apply the researchers' work to bona fide business contexts.
Customers can subscribe to these reports and engage FF to help them build out a data science organization. This includes helping customers recruit data scientist talent, and also to determine priorities and initiatives. FF will mentor customers through early initiatives that can demonstrate quick results and strong ROI, and may then progress into more sophisticated work, with higher risk but also higher potential value. FF will even do machine learning development work for customers -- but all with an eye toward helping the customer get its in-house data science group going -- and not acting as that group on an outsourced basis.
AI for the rest of us?
For those unable to engage FF, what are some ways to make AI more actionable? There's been lots of talk lately about automated machine learning (AutoML) as a "democratizing" technology. And, of course, there are lots of data science platforms available today, from various companies, including Cloudera.
Where does Mason come down on such technologies? To begin with, she predicts AutoML will become commoditized within a few years. She sees it less as a tool for business users to do AI, and more as a productivity tool for data scientists whose skills we will still need. Of course, Mason is a data scientist and may have some vested interest in that point of view; AutoML probably benefits both groups, and could benefit developers as well.
Mason says today's tooling is geared towards technical data scientists and that that's not enough - since data engineers and admins need to be part of the equation, too. She says Cloudera's ambitions go well past its current Data Science Workbench offering. So maybe we'll see Cloudera support these other AI workloads, and perhaps that commoditized AutoML technology will be brought to bear as well.
On-board with "boring"
One thing is pretty clear though: if one of the industry's foremost data scientists thinks we have to calm down about the technology of AI, then vendors and customers should focus more on its prolific and careful application, and less on its gloss and prestige.
Early startups typically have a tough time doing that, though, so it may be up to the Clouderas, Microsofts, Amazons and Googles of the world to push us further along.