Comparative thinking: Are analogies the engine of innovation?
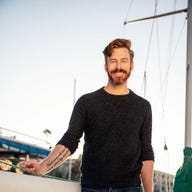
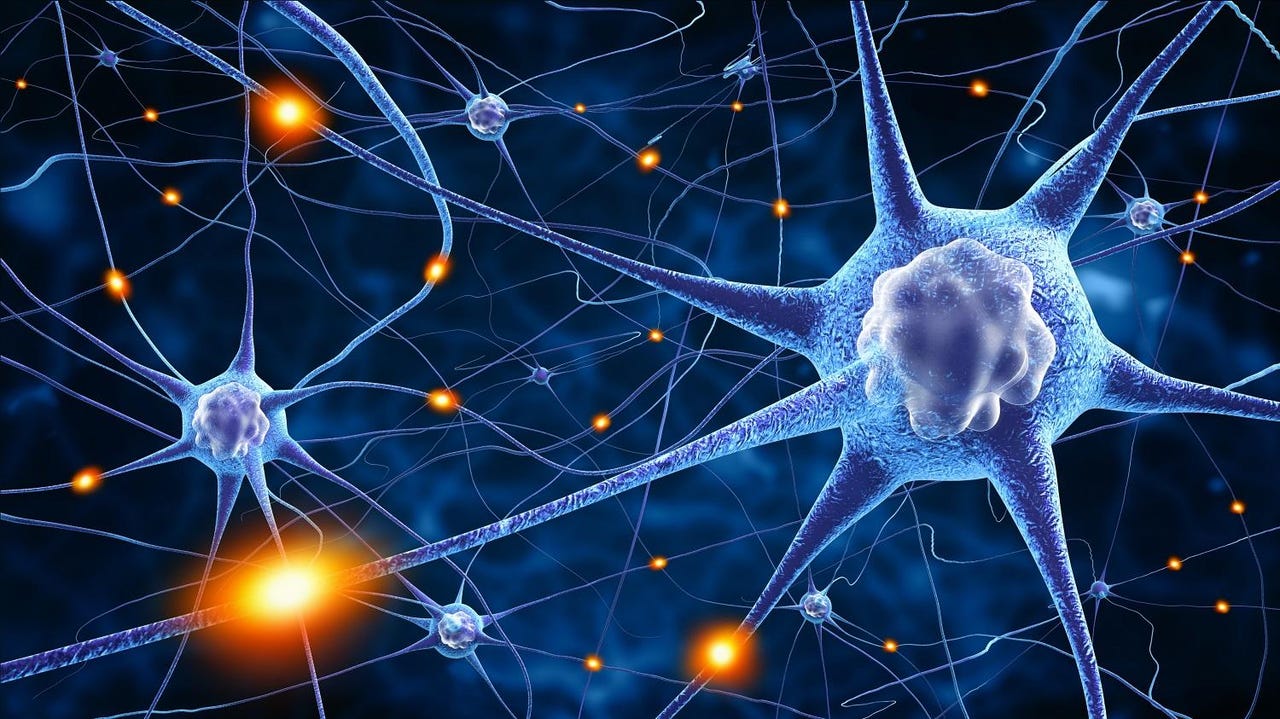
A couple years ago I wrote about an effort to emulate analogous thinking in order to tackle difficult problems and drive innovation. The research, conducted by computer scientists at Carnegie Mellon University and Hebrew University in Jerusalem, sought to harness the power of a deceptively simple intellectual trick: Solving a complex problem by making unlikely connections to known problem-solution sets that might seem unrelated on the surface.
If you could train AI to "think" analogously, the premise holds, you'll drive an explosion of innovation.
The researchers are back, abetted by scientists from the Bosch Research and Technology Center in Pittsburgh, the University of Maryland, and New York University Stern School of Business, with a new report published online this week by the Proceedings of the National Academy of Sciences. The report describes a process for utilizing remote workers and AI in concert to identify analogies. The possible upshot is a machine learning toolkit that could accelerate innovation and lift us past what some describe as an innovation plateau.
"People are really interested in how we start generating breakthroughs again," explains Dafna Shahaf, assistant professor of computer science at Hebrew University of Jerusalem. "The pace of discovery is high, but does not scale with the amount of resources invested in research."
Breakthroughs have long been driven by the perfect analogy. A CMU spokesperson offered me the example of Wilbur Wright, who got his idea for wing warping, a crucial prinicple for steering an airplane, while twisting a cardboard box. But after decades of frenzied innovation and technology development, much of the low hanging fruit of innovation has been plucked. Facing increasingly complex challenges, it's less likely that a lone genius will spark to a perfect analogy to solve a problem in its entirety.
Analogous problem-solving has to scale to keep up. Instead of an individual solving a problem in isolation, the authors of the National Academy of Sciences report suggest that problems can be tackled using a mix of individuals, who can solve portions of problems in their area specialties, and AI, coordinating the efforts to come up with better analogies and yield more efficient scientific discovery.
The researchers have relied on crowd workers at Amazon Mechanical Turk to crowd source analogous thinking. In one example, the authors developed an AI tool that enables a designer to specify the focus of a product description and then abstract it. In a simple example, you can imagine a description of a screw top Nalgene water bottle. Abstracting the description of the water bottle, you'd be talking about a vessel to hold a quantity of liquid or other material that has a screw lid.
It doesn't take much of an imaginative leap to transfer the insights from the Nalgene product to a new product to hold, say, coffee. But that's precisely the intellectual maneuver that, scaled up, can lead to real solutions concerning massively complex issues like overpopulation, long distance space travel, or cures for devastating illnesses.
One way this could work, according to the researchers, is by teaching AI to recognize the purpose or research goal sections of academic papers and, using AI models, identify other papers that share common purposes, even if they're from different topic areas. This would help solve one of the most insidious problems with the current academic research ecosystem, where vast bodies are knowledge are siloed into different departments and specialties.
The authors of the repot point out that there are more than 9 million U.S. patents and 2 million product or solution ideas on platforms such as Kickstarter and InnoCentive, which form a robust database of problem-solution sets from which to extract analogous innovations.
According to the researchers, there are three primary challenges to tackle. One is the sheer volume of information, which necessitates a machine learning approach. Another is the tendency of humans to fixate on surface-level details rather than deep concepts, the latter of which, like fundamental laws of physics, tend to travel easily between fields. A third is the sheer complexity of real-world problems, which require solutions of several sub-problems, which means multiple analogies at multiple levels of abstraction.
It's fascinating research, as it seeks not only to identify the underpinnings of human problem-solving, but scale it in an applicable way.
Solving those challenges could usher in a new era of discovery, explains Niki Kittur, a professor in Carnegie Mellon University's Human-Computer Interaction Institute, providing people with the inspiration necessary to make breakthroughs now just beyond our reach.