Data scientist: The cult of pan-galactic data doesn't work for business
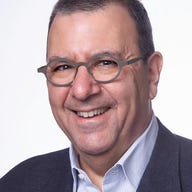
In the executive suite, they stuff data-rich dashboards with chart junk to create useless and misleading graphics. Meanwhile, many companies vacuum up as much customer data as they can find, to leave their analytics options open in the future.
Both of these examples reflect a cultish attitude toward data, based on the belief that collecting data is a useful, even virtuous, activity that creates its own reward. These notions rest on wrongheaded, yet popular, misconceptions about how to aggregate and use data to best advantage.
Getting to the root of this problem is difficult, so I invited two experts to join me on Episode 270 of the CXOTalk series of conversations with the world's top innovators:
- Anthony Scriffignano, Ph.D. is the Chief Data Scientist at Dun & Bradstreet (disclosure: CXOTalk underwriter) and a well-known figure in the world of data science.
- David Bray, Ph.D. is Executive In-Residence at Harvard University and Executive Director at People-Centered Internet. Previously, he was CIO at the Federal Communications Commission.
The two guests explode misconceptions on the value of aggregating data without a clear, underlying business strategy. The discussion addresses the problem from both a data science and business perspective.
Featured
These guys are smart, smart, smart so I urge you to watch the video embedded above and read the edited comments below. You can also see a complete transcript at the CXOTalk site.
And, yes, they even discuss the evils of viewing data in a pan-galactic manner. Read closely and remember, you heard it here first!
What's wrong with accumulating all the data you can get your hands on?
David Bray: Conventional wisdom says that more data equals better output. Anthony and I want to underscore it's about, one, the quality of the data, but also the diversity of the data. If you have a lot of data, but it's extremely biased, or it's missing what you really want to focus on as a business, then it's not going to be relevant to what you're trying to achieve.
To operationalize and think about data go back to first principles and say, "What is the problem we are trying to solve? What are the insights we're trying to gain?" Once you answer those questions, then you can say, "Are we looking in the right places? Are we analyzing the correct data from those streams? And, is it diverse enough to make sure we're not getting bias introduced into what we are seeing or we're actually missing things and having blind spots?"
Anthony Scriffignano: Yeah, if I can just add to that. The amount of data on earth is doubling at a rate that is arguably unmeasurable right now. There are lots of studies that they're all looking at different things. How much data is transferred on the Internet? How much data is stored on devices? All of those are proxies. We don't know anymore.
There's a sense of thinking, "Well, gee. That's a lot more fodder. We can learn a lot more from that data." It turns out that data begets data. So, an event happens, and there's a lot of data that is just duplicative. Just having more information doesn't mean you necessarily have more ground truth.
TechRepublic: 5 big data focal points for CDOs and CIOs in 2018
If you use things like machine learning, they see more evidence of something. They train themselves on that and believe those things are more important. Then we become focused on things that are probably less important, but more talked about or more occurring in the data.
David, you brought up the idea of veracity. That's a very big deal. You know I jokingly say, "Well, it's on the Internet. It must be true." Well, we know that's not true, right? AI tends to ingest things and then treat it as true. More and more, it's becoming important to have these skills such as knowing when I have enough data; the right data; understanding the bias; being able to defend stopping where I stopped; questioning how do I know it's true?
These are all critically important skills. It's not just, "Bring me more data." That's almost certainly going to make things worse in today's world.
What's the takeaway here for executives?
Anthony Scriffignano: You have to ask a good question. Don't start with the data. There's a big tendency to start with -- I get a message or a phone call a week from somebody. I call it the "Have I got a dataset for you" conversation.
It's great that you have a dataset. Congratulations. I wish you well. But, what problem am I trying to solve? I talk to you about how our customers' problems are changing.
How can executives formulate the right questions to ask?
David Bray: If I could wax poetically, briefly, just because you know we like to interject a combination of both left brain and right brain thinking here on CXOTalk, E.E. Cummings once said, "Always a more beautiful answer that asks a more beautiful question."
As Anthony pointed out, there are new requirements being placed on certain businesses regarding knowing who you're doing business with.
Okay, so that's the answer that I want to achieve. Then the question is, how do I know who I'm doing business with at a level that is sufficient and dependable, so in case that later someone says, "Well, you were working with this following company. Did you happen to know that they were doing money laundering?" or something like that. You can say, "Well, we did these following checks, and this is how we didn't find anything at the time," or, "it was apparent that they weren't doing at the time."
You need to think about, again, what is the beautiful answer that you want to achieve, and then what are the beautiful questions that you need to make sure that you're rigorous. I think the other thing that you can also do is you can also pull together almost different people from your business units and say, "What are the important questions that our customers are asking us, our stakeholders are asking us?" Almost collectively brainstorm [to uncover] interesting questions we're being posed that we cannot answer. Then, you can create a prioritized list, "If I had to answer these top three questions, these would be the things I'd want to achieve."
Anthony Scriffignano: There's something that we talk about a lot. We call it the dispositive threshold. It's not a term you can look up anywhere. I made it up. It's the point at which you can dispose of the question. You can answer the question with the data.
The tricky thing is that when you get to that point, you have enough data to answer the question, but it's not necessarily enough data to answer the question right. Now, how do we define right? How do we define good enough? How do we define how raw my analysis needs to be before it would make a different decision? These are very, very big questions, and they're not answerable necessarily with math.
Sometimes, as David said, you've got to go talk to the users and say, "Are you marketing to them? Are you trying to sue them? Is somebody going to die if we get this wrong?" Those are different levels of adjudication, I would hope. We need to understand the sensitivity, the decision elasticity in how we're using data to use it as practitioners and not just pushing buttons and producing reports.
David Bray: If I may build on what Anthony said, [and share] a practical example.
Back in 2001, 2002, I was with the bioterrorism preparedness response program at the U.S. Centers for Disease Control. We were working with the state public health facilities and public health labs to try and figure out when they saw an increase in flu, things such as that.
Suddenly, one day, one of my team members came running to me and said, "We've just seen a five-times increase in the amount of flu in the southwestern part of the United States." I was like, "Well, that's curious. What's going on here?" When we looked, what happened was they were only updating their record set once every month, and so you got the data volume all at the same time.
TechRepublic: How Apple plans to make patient data rule the future of medicine
Unfortunately, that made it all of a sudden look like there was a traumatic spike. And so, as Anthony mentioned, it's about making sure you ask the right question and then also understand the context in which the data is being received.
Also, a lot of our statistical methods were developed for an era in which I would say is not big data. The challenge is, as you grow the amount of data, you may find things that show up mathematically as appearing to be statically significant but, in the real world, might not be correlated whatsoever.
Anthony Scriffignano: There is a common assumption in math that when we develop a regression equation that explains what we're looking at well enough to stay within a certain tolerance, we can call it a day and move on. The rest of the data that varies from the equation that we're using to predict the behavior is considered to be random.
It turns out it's not random at all. It contains pockets of bad guys. It contains pockets of fascinating new opportunity. And so, we have to be able to use methods, and sometimes there are AI methods that can do things like this. Some methods are not supervised necessarily; methods based on observation, recursion, and learning.
Are we using modern methods, or are we using math from 1980? We need to ask that question. If the answer is we're using math from 1980, there's no reason to shoot yourself. It's just that you might want to get a little help.
Tell us about data velocity, context, and related issues?
Anthony Scriffignano: [For example,] we want to understand total risk and total opportunity. What are the things that move slowly regarding total risk? Well, how people pay their bills every month. That moves roughly on a monthly basis in different billing cycles, but we understand. You don't pay your bills every millisecond, right?
What moves more quickly? Well, fraud moves pretty quickly, right? Maybe I want to have a more agile process for detecting potential malfeasants than for detecting a change in propensity to pay bills. It's a question of not trying to paint everything with one broad brush and say, "This is the pan-galactic answer for everything we're going to do with data."
We wind up in this conundrum where I want to see something holistically as if all the data was in one place, but probably the worst thing to do is to try to put all the data in one place. Again, you don't have to give up and walk out of the room. That's an approachable problem. Hospitals deal with it all the time. There's biometric data that are being produced in real time, and there's billing data that gets produced once a month. We can fix this problem if we'd just back up from it and stop trying to conquer it with a simple assumption.
David Bray: Well, I think what he's mentioning is that context, context, context. Context matters.
If there's anything that we want people to take away in 2018 is understanding the context of how your data is produced, how it's used, and how you want it to answer your questions. That really matters.
Figure out the velocity of the data that you're dealing with. If it's at a millisecond update, you're almost looking for the meta-constructs. What are the trends in that data that are relevant to the question you're asking? Whereas, if it's something that happens once a month, that might be more easily analyzable in a different fashion.
Anthony Scriffignano: There's one other nuance to what David is talking about, which I think is worth bringing up here.
The science of understanding metadata is now coming through its adolescence. It used to be that the metadata was just this sort of dictionary that comes with the data that tells us how it's structured. Well, most data is unstructured these days, so good luck with that.
But, there is a lot of metadata, even with a video that's posted, [for example]. I know when it was posted. I know who posted it. I know how long it is. I know what format it's in. All of those things are part of the metadata.
Also: Mix and match analytics: data, metadata, and machine learning for the win
Imagine that I could look across the metadata that's available in a certain sphere that I'm looking at. [Then,] I could see that it suddenly spiked; or that the sentiment of the comments, the mean sentiment of the comments, has suddenly shifted negatively; or that a common phrase has emerged that was never common before. That might be just enough to say to me, "Pay attention to this."
Now I want to pull all that data in for the time being because I know that something interested has happened. It's the way your brain works. I like to say that nobody is paying attention to how their shoes feel until you say that. Then everybody starts to think about their shoes. Your brain does this all the time. We have algorithms that can do this as well.
CXOTalk brings together the most world's top business and government leaders for in-depth conversations on digital disruption, AI, innovation, and related topics. Be sure to watch our many episodes! Thumbnail image Creative Commons from Pixabay.