Deep learning godfathers Bengio, Hinton, and LeCun say the field can fix its flaws
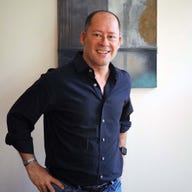
From left, Geoffrey Hinton, Yann LeCun, Yoshua Bengio.
Artificial intelligence has to go in new directions if it's to realize the machine equivalent of common sense, and three of its most prominent proponents are in violent agreement about exactly how to do that.
Primers
Yoshua Bengio of Canada's MILA institute, Geoffrey Hinton of the University of Toronto, and Yann LeCun of Facebook, who have called themselves co-conspirators in the revival of the once-moribund field of "deep learning," took the stage Sunday night at the Hilton hotel in midtown Manhattan for the 34th annual conference of the Association for the Advancement of Artificial Intelligence.
The three, who were dubbed the "godfathers" of deep learning by the conference, were being honored for having received last year's Turing Award for lifetime achievements in computing.
Each of the three scientists got a half-hour to talk, and each one acknowledged numerous shortcomings in deep learning, things such as "adversarial examples," where an object recognition system can be tricked into misidentifying an object just by adding noise to a picture.
"There's been a lot of talk of the negatives about deep learning," LeCun noted.
Each of the three men was confident that the tools of deep learning will fix deep learning and lead to more advanced capabilities.
The big idea shared by all three is that the solution is a form of machine learning called "self-supervised," where something in data is deliberately "masked," and the computer has to guess its identity.
For Hinton, it's something called "capsule networks," which are like convolutional neural networks widely used in AI, but with parts of the input data deliberately hidden. LeCun, for his part, said he borrowed from Hinton to create a new direction in self-supervised learning.
"Self-supervised is training a model to fill in the blanks," LeCun said.
"This is what is going to allow our AI systems to go to the next level," said LeCun. "Some kind of common sense will emerge."
And Bengio talked about how machines could generalize better if trained to spot subtle changes in the data caused by the intervention of an agent, a form of cause and effect inference.
In each case, masking information and then guessing it is made possible by a breakthrough in 2017 called the "Transformer," made by Google scientists. The Transformer has become the basis for surprising language learning, such as OpenAI's "GPT" software. Transformer exploits the notion of "attention," which is what will allow a computer to guess what's missing in masked data. (You can watch a replay of the talks and other sessions on the conference website.)
The prominent panel appearance by the deep learning cohort was a triumphant turnaround for a sub-discipline of AI that had once been left for dead, even by the conference itself. It was a bit paradoxical, too, because all three talks seemed to borrow terms that are usually identified as belonging to the opposing strain in AI, the "symbolic" AI theorists, who were the ones who dismissed Bengio and Hinton and LeCun years ago.
"And yet, some of you speak a little disparagingly of the symbolic AI world," said the moderator, MIT professor Leslie Kaelbling, noting the borrowing of terms. "Can we all be friends or can we not?" she asked, to much laughter from the audience.
Hinton, who was standing at the panel table rather than taking a seat, dryly quipped, "Well, we've got a long history, like," eliciting more laughter.
"The last time I submitted a paper to AAAI, it got the worst review I've ever got, and it was mean!" said Hinton.
"It said, Hinton's been working on this idea for seven years and nobody's interested, it's time to move on," Hinton recalled, eliciting grins from LeCun and Bengio, who also labored in obscurity for decades until deep learning's breakthrough year in 2012. "It takes a while to forget that," Hinton said, though perhaps it was better to forget the past and move forward, he conceded.
Bengio and LeCun look on as Hinton describes mistreatment in the bad days before deep learning broke through. "The last time I submitted a paper to AAAI, it got the worst review I've ever got, and it was mean!"
Kaelbling's question struck home because there were allusions in the three scientists' talk about how their work is frequently under attack from skeptics.
LeCun noted he is "pretty active on social media and there seems to be some confusion" as to what deep learning is, which was an allusion to back-and-forth debates he's had on Twitter with deep learning critic Gary Marcus, among others, that have gotten combative at times. LeCun began his talk by offering a slide defining what deep learning is, echoing a debate in December between Bengio and Marcus.
Mostly, however, the evening was marked by the camaraderie of the three scholars. When asked by the audience what, if anything, they disagreed on, Bengio quipped, "Leslie already tried that on us and it didn't work." Hinton said, "I can tell you one disagreement between us: Yoshua's email address ends in 'Quebec,' and I think there should be a country code after that, and he doesn't."
There was also a chance for friendly teasing. Hinton began his talk by saying it was aimed at LeCun, who made convolutional neural networks a practical technology thirty years ago. Hinton said he wanted to show why CNNs are "rubbish," and should be replaced by his capsule networks. Hinton mocked himself, noting that he's been putting out a new version of capsule networks every year for the past three years. "Forget everything you knew about the previous versions, they were all wrong but this one's right," he said, to much laughter.
Some problems in the discipline, as a discipline, will be harder to solve. When Kaelbling asked whether any of them have concerns about the goals or agenda of big companies that use AI, Hinton grinned and pointed at LeCun, who runs Facebook's AI research department, but LeCun grinned and pointed at Hinton, who is a fellow in Google's AI program. "Uh, I think they ought to be doing things about fake news, but..." said Hinton, his voice trailing off, to which LeCun replied, "In fact, we are." The exchange got some of the biggest applause and laughter out of the room.
They also had thoughts about the structure of the field and how it needs to change. Bengio noted the pressure on young scholars to publish is far greater today than when he was a PhD student, and that something needs to change structurally in that regard to enable authors to focus on more meaningful long-term problems. LeCun, who also has a professorship at NYU, agreed times have changed, noting that as professors, "we would not admit ourselves in our own PhD programs."
With the benefit of years of struggling in obscurity, and with his gentle English drawl, Hinton managed to inject a note of levity into the problem of short-sighted research.
"I have a model of this process, of people working on an idea for a short length of time, and making a little bit of progress, and then publishing a paper," he said.
"It's like someone taking one of those books of hard sudoku puzzles, and going through the book, and filling in a few of the easy ones in each sudoku, and that really messes it up for everybody else!"