What’s in a name? The ‘deep learning’ debate
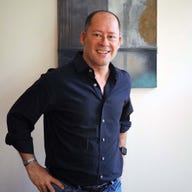
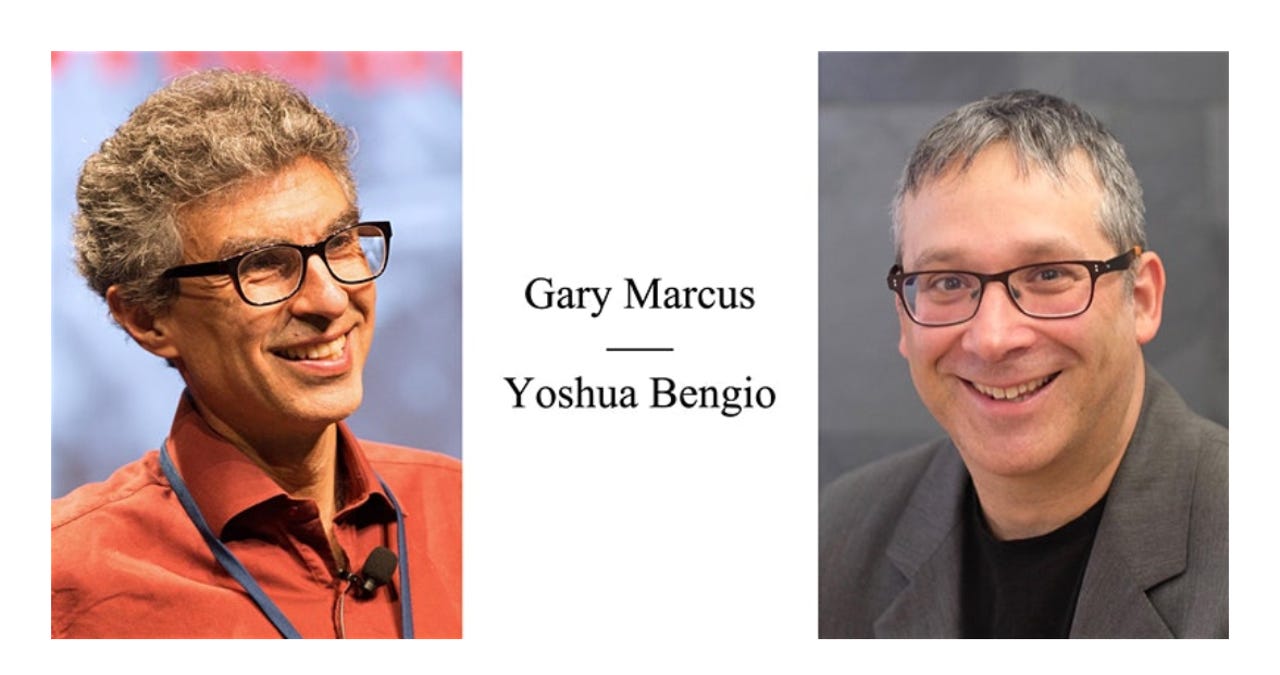
Monday's historic debate between machine learning luminary Yoshua Bengio and machine learning critic Gary Marcus spilled over into a tit for tat between the two in the days following, mostly about the status of the term "deep learning."
The history of the term deep learning shows that the use of it has been opportunistic at times but has had little to do in the way of advancing the science of artificial intelligence. Hence, the current debate will likely not go anywhere, ultimately.
Monday night's debate found Bengio and Marcus talking about similar-seeming end goals, things such as the need for "hybrid" models of intelligence, maybe combining neural networks with something like a "symbol" class of object. The details were where the two argued about definitions and terminology.
In the days that followed, Marcus, in a post on Medium, observed that Bengio seemed to have white-washed his own recent critique of shortcomings in deep learning. And Bengio replied, in a letter on Google Docs linked from his Facebook account, that Marcus was presuming to tell the deep learning community how it can define its terms. Marcus responded in a follow-up post by suggesting the shifting descriptions of deep learning are "sloppy." Bengio replied again late Friday on his Facebook page with a definition of deep learning as a goal, stating, "Deep learning is inspired by neural networks of the brain to build learning machines which discover rich and useful internal representations, computed as a composition of learned features and functions." Bengio noted the definition did not cover the "how" of the matter, leaving it open.
Bengio's MILA institute, where Monday's debate took place, is keeping a running archive of links of the various installments by both individuals.
Also: Devil's in the details in Historic AI debate
The term "deep learning" has emerged a bunch of times over the decades, and it has been used in different ways. It's never been rigorous, and doubtless it will morph again, and at some point it may lose its utility.
Jürgen Schmidhuber, who co-developed the "long-short term memory" form of neural network, has written that the AI scientist Rina Dechter first used the term "deep learning" in the 1980s. That use was different from today's usage. Dechter was writing about methods to search a graph of a problem, having nothing much to do with deep networks of artificial neurons. But there was a similarity: she was using the word "deep" as a way to indicate the degree of complexity of a problem and its solution, which is what others started doing in the new century.
The same kind of heuristic use of deep learning started to happen with Bengio and others around 2006, when Geoffrey Hinton offered up seminal work on neural networks with many more layers of computation than in past. Starting that year, Hinton and others in the field began to refer to "deep networks" as opposed to earlier work that employed collections of just a small number of artificial neurons.
From Yoshua Bengio's slides for the AI debate with Gary Marcus, December 23rd.
So deep learning emerged as a very rough, very broad way to distinguish a layering approach that makes things such as AlexNet work.
In the meantime, as Marcus suggests, the term deep learning has been so successful in the popular literature that it has taken on a branding aspect, and it has become a kind-of catchall that can sometimes seem like it stands for anything. Marcus's best work has been in pointing out how cavalierly and irresponsibly such terms are used (mostly by journalists and corporations), causing confusion among the public. Companies with "deep" in their name have certainly branded their achievements and earned hundreds of millions for it. So the topic of branding is in some sense unavoidable.
Bengio's response implies he doesn't much care about the semantic drift that the term has undergone because he's focused on practicing science, not on defining terms. To him, deep learning is serviceable as a placeholder for a community of approaches and practices that evolve together over time.
Also: Intel's neuro guru slams deep learning: 'it's not actually learning'
Probably, deep learning as a term will at some point disappear from the scene, just as it and other terms have floated in and out of use over time.
There was something else in Monday's debate, actually, that was far more provocative than the branding issue, and it was Bengio's insistence that everything in deep learning is united in some respect via the notion of optimization, typically optimization of an objective function. That could be a loss function, or an energy function, or something else, depending on the context.
In fact, Bengio and colleagues have argued in a recent paper that the notion of objective functions should be extended to neuroscience. As they put it, "If things don't 'get better' according to some metric, how can we refer to any phenotypic plasticity as 'learning' as opposed to just 'changes'?"
That's such a basic idea, it seems so self-evident, that it almost seems trivial for Bengio to insist on it.
But it is not trivial. Insisting that a system optimizes along some vector is a position that not everyone agrees with. For example, Mike Davies, head of Intel's "neuromorphic" chip effort, this past February criticized back-propagation, the main learning rule used to optimize in deep learning, during a talk at the International Solid State Circuits Conference.
Davies's complaint is that back-prop is unlike human brain activity, arguing "it's really an optimization procedure, it's not actually learning."
Thus, deep learning's adherents have at least one main tenet that is very broad but also not without controversy.
The moral of the story is, there will always be something to argue about.