DeepMind AI breakthrough in protein folding will accelerate medical discoveries
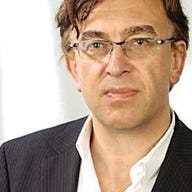

An illustration of the possible structure of a "membrane protein" associated with the coronavirus, according to a model created by DeepMind's AlphaFold program.
DeepMind, a division of Alphabet, says it has solved one of the most difficult computing challenges in the world: predicting how protein molecules will fold. It is key to understanding important biological processes and treating diseases such as COVID-19.
The London-based organization said that its claims of a breakthrough had been verified by organizers of a competition held every two years to test computer models, the Critical Assessment of protein Structure Prediction (CASP).
DeepMind named its protein folding prediction system AlphaFold and said that the latest version has been four years in development.
Writing on its blog, the AlphaFold team described the success of the system being due to methods that "draw inspiration from the fields of biology, physics, and machine learning, as well as of course the work of many scientists in the protein folding field over the past half-century."
There are about 180 million known proteins but only about 170,000 protein structures have been mapped through X-ray crystallography and other techniques. X-ray crystallography is how DNA's double-helix of amino acids structure was discovered and the structure revealed how it copied itself. But it can take months and sometimes years to determine a protein structure.
Complicated chains of amino acids can have vast numbers of permutations. Yet in nature proteins will only fold into a very specific shape and that shape determines its role in biological processes, including in viruses.
Professor Andrei Lupas, Director of the Max Planck Institute for Developmental Biology, writing on the DeepMind blog: "AlphaFold's astonishingly accurate models have allowed us to solve a protein structure we were stuck on for close to a decade, relaunching our effort to understand how signals are transmitted across cell membranes."
DeepMind's approach is ideal for membrane proteins which cannot be easily crystalized.
The AlphaFold team said that in March it predicted two protein structures of SARS-CoV-virus, which had been separately identified months later by researchers. This shows its potential applications in predicting the shape of mutated viruses.
What is digital health?
The CASP competition evaluates competing models of prediction by measuring the variation from actual structure in Angstroms -- the width of an atom. Competitors analyze samples of proteins whose structure has never been published.
Units called Global Distance Test (GDT) are used to evaluate each protein structure prediction. A score of 90 GDT or above is considered equal to experimental analysis. AlphaFold's median score against all target proteins was 92.4 GDT.
What is additionally impressive about this achievement is the seemingly small amount of data AlphaFold was trained on. With only some 170,000 known protein structures in public databases AlphaFold had to determine the rules for a complex structure from very little information.
AlphaFold's training was very fast compared against other types of large computing problems. It took just a few weeks running on hardware consisting of 128 TPUv3 cores.
However, DeepMind cautions: "There are still many questions to answer. Not every structure we predict will be perfect. There's still much to learn, including how multiple proteins form complexes, how they interact with DNA, RNA, or small molecules, and how we can determine the precise location of all amino acid side chains."
More information on AlphaFold is here.