Google DeepMind’s effort on COVID-19 coronavirus rests on the shoulders of giants
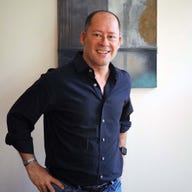
There's been a quest for sixty years to understand the structure of proteins, ever since Nobel Prize winners Max Perutz and John Kendrew in the 1950s gave the world the first glimpse of what a protein looks like.
It was that pioneering work, and the decades of research that followed, that made possible the announcement on Thursday by Google's DeepMind that it has arrived at a guess as to the structure of a handful of proteins associated with the respiratory disease known as COVID-19 that is spreading around the world.
Proteins do the vast amount of the work of organisms, and understanding the three-dimensional shape of the proteins in COVID-19 could conceivably provide a kind of blueprint of the virus behind the disease, which could conceivably aid in coming up with a vaccine. Efforts are underway around the world to determine the structure of those viral proteins, of which DeepMind's is just one effort.
There's always something a bit self-promotional about DeepMind's AI achievements, and so it helps to remember the context in which the science is created. DeepMind's protein-probing program reflects decades of work by chemists and physicists and biologists and computer scientists and data scientists and wouldn't be possible without that intense global effort.
Since the 1960s, scientists have been fascinated by the difficult problem of protein structure. Proteins are amino acids, and the forces that make them curl up into a given shape are fairly straightforward, things like certain amino acids being attracted or repelled by positive or negative charges, and some amino acids being "hydrophobic," meaning, they stay farther away from water molecules.
But those forces, so basic and so easy to understand, lead to startling protein shapes that are hard to predict from just the acids themselves. And so decades have been spent trying to guess what shape a given sequence of amino acids will take, usually by developing more and more sophisticated computer models to simulate the "folding" process of a protein, the interaction of forces that cause a protein to take whatever shape it ends up taking.
An illustration of the possible structure of a "membrane protein" associated with the coronavirus, according to a model created by DeepMind's AlphaFold program.
Twenty-six years ago, a bi-annual contest ensued, called "Critical Assessment of protein Structure Prediction," or CASP. Scientists are challenged to submit their best computer-simulated predictions of a given protein after being told only the sequence of amino acids. The judges know the structure, which is determined via a lab experiment, so it's a test of how good computer work can guess what's found in a lab.
DeepMind took honors at the most recent CASP, CASP13, which was conducted throughout 2018. To take the gold, DeepMind developed a computer model, AlphaFold, which shares a naming convention with the DeepMind model that won at chess and the game of Go, called AlphaZero. In one of those trophy moments similar to other DeepMind headlines, the company trounced its nearest competitor at the CASP13 competition in 2018, producing "high-accuracy structures" for 24 out of 43 "domains" of proteins, with the next-best effort only producing 14 such models.
Writing in Nature magazine this past January, Mohammed AlQuraishi with the Laboratory of Systems Pharmacology at Harvard Medical School, called AlphaFold's achievement a "watershed moment" for protein folding science. His essay accompanies DeepMind's formal AlphaFold scientific paper in that issue, titled "Improved protein structure prediction using potentials from deep learning."
What makes up AlphaFold is a union of AI work at DeepMind, itself the product of decades of machine learning progress, but also those decades of protein knowledge assembled in the public domain. The deep neural network developed by DeepMind consists of a mechanism of measuring the local assembly of atoms in a protein that is similar to the convolutional filter perfected by Turing Award winner Yann LeCun and used in the ubiquitous convolutional neural networks to determine the local structure of an image. To that, DeepMind added so-called "residual blocks" of the kind developed some years ago by Kaiming He and colleagues at Microsoft.
DeepMind calls the resultant structure a "deep two-dimensional dilated convolutional residual network." The goal of that mouthful is to predict the distance between pairs of amino acids given their sequence. AlphaFold does it by optimizing its convolutions and residual connections via the stochastic gradient descent learning rule developed in the 1980s that powers all of deep learning today.
That AlphaFold network would not be possible without decades of knowledge of proteins built up in publicly accessible databases. The deep network takes as input the known sequence of amino acids, in a form called a "multiple sequence alignment," or MSA. These are the equivalent of the pixels in an image that a CNN operates on when it does image recognition. Those MSAs are only at hand because scientists have spent decades assembling them in databases, in particular, the UniProt database, or Universal Protein Resource, which is maintained by a consortium of research centers around the world, and which is funded by a gaggle of government offices, including the U.S.'s National Institutes of Health and National Science Foundation. DeepMind's six protein structures posted this week for COVID-19 started by taking the aminoacid sequences freely available in UniProt, so UniProt is the raw material for DeepMind's science.
In addition, on the way to achieving its stunning results, AlphaFold had to be "trained." The deep network of convolutions and residual blocks had to acquire its shape by being given examples of known structures as labeled examples. That was made possible by another organization, now 49 years old, called the Protein Data Bank, funded by NSF, the US Department of Energy, and others. PDB's "core" database is managed by a consortium of Rutgers University, the San Diego Supercomputer Center/University of California San Diego and the National Institute of Standards and Technology. Those institutions shoulder the awesome task of curating what you might consider Enormous Data that makes possible AlphaFold and other efforts. Over 144,000 structures of proteins have been gathered and can be downloaded, and boy, are they downloaded: nearly half a billion times annually, according to the PDB. PDB also runs the CASP challenge.
DeepMind's structure predictions are themselves posted in the so-called "PDB" file format of the consortium. That means even the language with which DeepMind can express its scientific findings is made possible by the consortium.
The fact that dedicated teams have spent decades meticulously assembling storehouses of knowledge from which researchers can freely draw is an astounding achievement in the history of science and, indeed, of humanity.
DeepMind's release of the protein files was lauded by fellow scientists, such as the Francis Crick Institute. In their blog post about their COVID-19 work, DeepMind's scientists acknowledge lots of work on the virus by other institutions. "We're indebted to the work of many other labs," they write, "this work wouldn't be possible without the efforts of researchers across the globe who have responded to the COVID-19 outbreak with incredible agility."
That's a responsible and dignified acknowledgment. One might add that it's not only today's labs that have made possible the AlphaFold files, but it's also generations worth of work by public and private outfits that have made possible the collective insight of which AlphaFold is just the latest interesting wrinkle.