Ensuring data quality not sexy but critical to biz
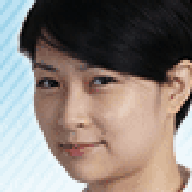
Many of today's major IT initiatives revolve around data such as big data and analytics, so it is ironic that data quality, which helps ensure integrity and relevance of corporate information, has not been accorded the proper attention or budget by companies, analysts note.
Arun Chandrasekaran, research director at Gartner, said data quality, as a process and technology, identifies and manages the accuracy of business-critical data as well as corrects the flaw within the data set.
Companies that take a proactive approach to data quality have been in the minority though, as most organizations would rather endure the pains of poor data quality and deal with it in a reactive manner, he noted.
Agreeing, Madan Sheina, lead analyst for information management software at Ovum, said data quality is not the sexiest of IT topics among enterprises or as straightforward as they would like to believe. Many of the companies are in "self-denial" over the data quality issue, believing there are no "washing machine in which they can throw their data into, add detergent and a spin cycle, and the data comes out clean", he elaborated.
With this mindset, it is no surprise data quality struggles to compete with the likes of business intelligence, analytics, social media, and cloud computing for the organization's IT budget, Sheina noted.
Big data, in particular, has been dominating companies' considerations on IT priorities and expenditure, added Mark Koh, senior industry analyst of Asia-Pacific ICT practice at Frost & Sullivan. This, however, will soon have to change as organizations, in finding new ways to manage big data, recognize the importance and need for data quality checks, he said.
Challenges in ensuring data quality
Sheina pointed out that while the idea of data quality is simple enough, there are several aspects companies will need to grasp in order to achieve the desired quality for their information, especially since data volumes have been growing exponentially.
One aspect would be content accuracy and completeness, in which the data set has all the correct and necessary elements in place for business users to tap on, the Ovum analyst said. Consistency and accessibility across data sources will also need to be achieved so that the information is up to date and appropriate in terms of what can be accessed and by whom, he added.
Chandrasekaran also urged companies not to give up on data quality despite the deluge of information being generated.
"We are entering a new era where the data sources considered as part of the enterprise spectrum of information assets, such as social media, can overwhelm the organization systems. Factors such as timeliness, accuracy, consistency, and completeness [of data] need to be put in the context of the specific use case to derive optimal business value.
"Poor data quality affects operational efficiency, risk mitigation, and agility by compromising the decisions made in each of these areas [and] leads to business initiatives failing to achieve the stated objectives and benefits," the Gartner analyst said.
Not just IT's job
Chandrasekaran pointed out the key to successful data quality initiatives is making sure the organization does not fall into the trap of perceiving it as an IT-only issue as technology alone cannot solve this challenge.
Data quality is not a one-off IT project and carrying out such initiatives require a mindset shift from data ownership to data stewardship, Sheina added. As such, it becomes as much an exercise in change management as it is an IT implementation, he said.
"To some extent, IT has masked the data quality problem, which means business users assume they are working with accurate and trusted data. So business users need to get involved early on," he added.
The tech department would be able to implement the software that automates the cleansing of the information, but this is just a relatively small part of the overall project, the Ovum analyst said. More importantly, business users would have to define the rules that dictate whether the data provided is of sufficient quality in the context of its use case, he explained.
Thus, data quality is a journey, not a destination, and as organizations grapple with these challenges, they will also gain experience and mature their data quality initiatives, Sheina said.