Fight against financial crime requires both artificial and human intelligence
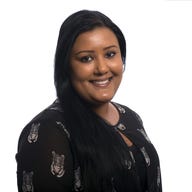
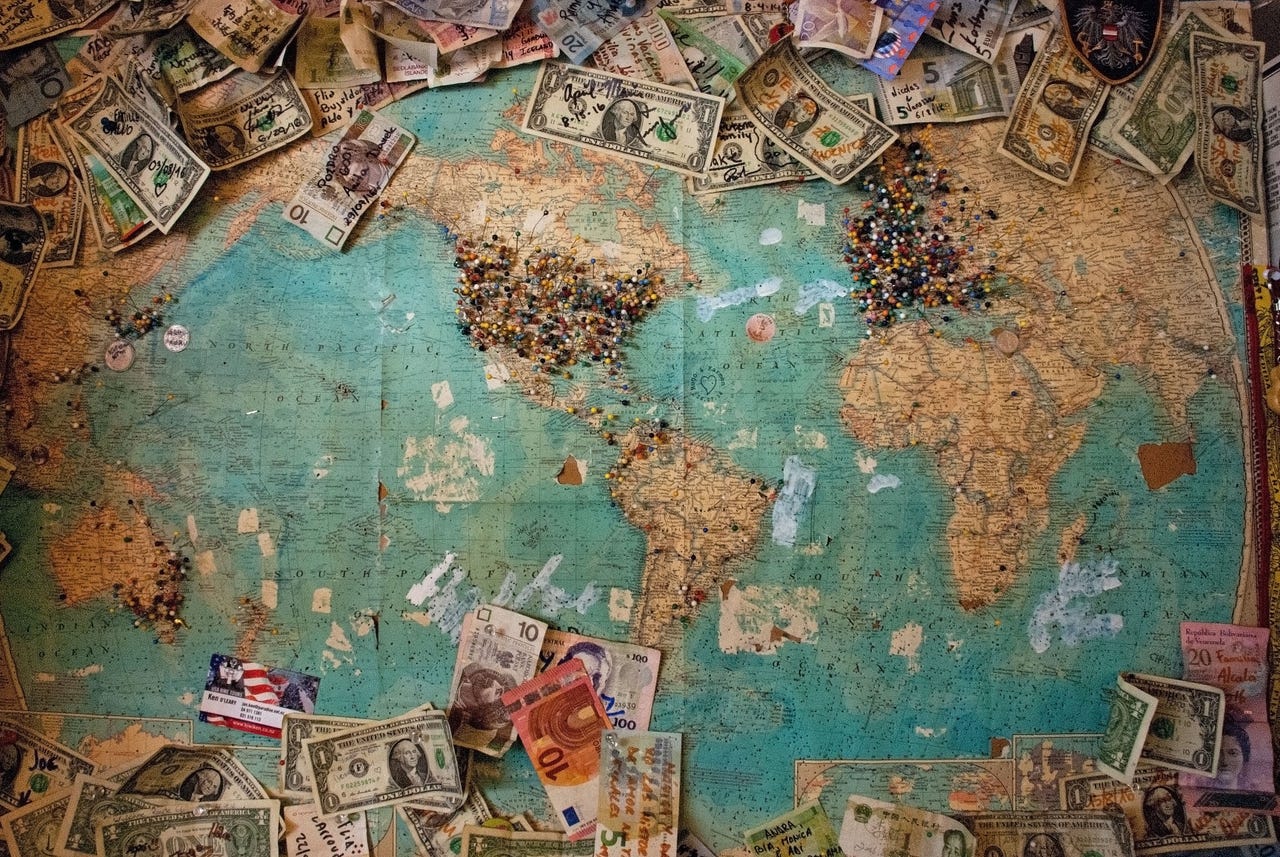
Today's criminals have an ideal playing field called the internet. As technology continues to advance, so too does crime, with criminals using the latest tools to commit a wide array of offences. Some even say criminals are the early adopters of new technology. The one thing that hasn't changed is the criminal's acumen and dexterity when it comes to the act of the crime -- whether it's pickpocketing, cyber-enabled identity fraud, or holding an organisation's critical data hostage for ransom.
Ken Chenis, chief architect at financial crime management solutions company ACI, told ZDNet that advances in technology -- including new software, hardware, algorithms, and data sources -- are allowing banks and financial services organisations around the globe to fine-tune their fraud mitigation strategies and explore approaches that were previously impossible due to technical resource constraints.
Artificial intelligence (AI), including its machine learning subset, is considered one of the most promising technologies that can help combat crime, and financial services is commonly cited as the industry that has much to gain by harnessing AI.
"AI is benefiting financial institutions, intermediaries, and merchants by enabling much more robust and complex fraud strategies that create a fine balance between fraud prevention strategies and maintaining a positive customer experience," Andreas Suma, global head of Fraud and Data at ACI, told ZDNet.
"In the merchant space, AI enables more granular fraud scoring behind the scenes, allowing better conversion rates and reducing false positives. For financial institutions, AI further enables enterprise fraud protection by monitoring transactions and interactions across channels and allowing a customer to have the same experience on all networks, regardless of whether it is in a branch, via call centre, or on a mobile app."
Combining artificial intelligence with human intelligence
Mark Wiesman, SVP and head of Fraud Management Solutions at Mastercard, told ZDNet that while AI is enabling the organisation to "dig deeper into data to understand where there are relationships that are trusted and not trusted", AI needs to be combined with human intelligence.
This is because AI and algorithms, as they exist today, still struggle with ambiguity and grey areas; they are not able to understand context or nuance, and therefore cannot make fully-informed judgment calls.
As such, the technology can end up flagging non-fraudulent transactions, and applying a layer of automation on top of it to halt suspicious transactions without human examination can create additional inefficiencies if the errors are frequent.
"There's this belief that you just plug in some new technology and it just starts to learn and does everything all by itself. Our experience here is that's really not the case. We have a lot of subject matter experts who are able to monitor and check what's going on," Wiesman said.
"I think it's a combination of the technology, the data, and the people that really make all of this work. Even though we're using AI techniques, there is still a need to get people involved."
Michelle Weatherhead, BAE's head of Financial Crime Solutions Australia & New Zealand, said the company's portfolio of products -- including its NetReveal platform used by banks globally -- also requires human analysis. The platform takes in large amounts of data to build profiles, subsequently determining what is normal and not normal.
"It's churning through vast amounts of data in real-time and then highlighting incidents that need to be investigated. For those incidents that are really suspicious, it can block that in real-time. For payment fraud, it's really important to be able to halt that in real-time, because if you have to alert and then have a human look at it, the money is already gone, out the door by the time that you've actually figured out that you need to stop it," Weatherhead told ZDNet.
She explained that BAE uses a combination of analytical models, including rudimentary and sophisticated types, to "find the needle in the haystack more accurately, more efficiently, and more flexibly."
"Basic types, like rules, are binary. For example, if it's jewellery transaction and it's over $10,000, then you might want to have a look at it if that customer doesn't normally do that type of transaction. Then we have more sophisticated models that accumulate statistics about customers and their peer groups, so profiling what's normal for that person and their peer group of customers," Weatherhead told ZDNet.
"The next level of sophistication is machine learning models ... that are much more trained using historical data -- so known good data and known bad data, meaning what has historically been shown to be fraudulent transactions and what has historically been shown to be normal transactions -- and that then builds up a more advanced analytical model that can be applied. There are supervised and unsupervised machine learning models on top of that which build on the sophistication."`
Wiesman said in trying to prevent fraud, organisations need to ensure they're not creating unnecessary barriers for legitimate transactions.
"There's only so much fraud you can cut out of the process before you really start to impact the good transactions that are out there ... What we did is we really tried to change the conversation, not by saying that fraud is irrelevant, but the focus should not just be on fraud detection, it should also be on approvals," he said.
"Driving a better experience for the consumer, driving better approval rates, driving less false declines has a real bottom-line impact for our issuing institutions and ultimately for the cardholders that are using their Mastercard cards.
"So it becomes more of an investment discussion and a bottom-line revenue discussion, rather than an [operating] expense discussion."
Mastercard's approach to increasing fraud detection rates, while reducing false positives, is incorporated in a product it launched last year called Decision Intelligence, Wiesman said.
"We built out a process and some new models that allow us to not only predict what might be fraud, but also added in other capabilities to the model to take a look at things that lead us to believe the transaction might actually be good instead of bad," he added.
Mastercard also uses SafetyNet, a multi-tier threat-thwarting product aimed at protecting all players in the payments ecosystem.
"There's a lot of cases where hackers will go in and potentially breach a financial institution and turn off the controls that the banks have or flood them with so many bad transactions that the banks can't keep up. We have built numerous types of intelligence into that product that's evolved from something that was a little more manual when it started into something that's much more intelligent and much more automated so that not only can it detect when something happens, it can automate the process that it goes through to help throttle down the activity and alert somebody of what's going on," Wiesman said.
"The type of intelligence we've built into it allows us to not only be smarter from a detection perspective but also smarter in terms of actions that are taken and notifications that are made. It's not a matter of having to call somebody and get a bunch of people on the phone as urgently as it would have been previously."
Wiesman additionally noted Mastercard's strategy of using AI for data preparation.
"When you think about artificial intelligence or the concept of structured learning and unstructured learning or supervised and unsupervised learning, supervise is what I know. I know that a transaction is fraud because the bank told me it was fraud because you called up and said 'I didn't do this'. But if a transaction just gets declined and I don't know why it was declined, my ability to better interpret the data and expand my view of what's going on helps me make better decisions," Wiesman said.
"Artificial intelligence to us isn't just about putting it in the network; it was actually changes that we've made to the whole ecosystem as part of our data preparation technology enhancements. It's all kinds of things that we had to do to really enable that capability at scale across multiple datacentres on every transaction. This is rather complicated to manage normally, so we put on top of it some of this new-age machine learning and other artificial intelligence techniques.
"It was a lot of work on our side, but again it's all about the results and we've seen very good results from the new products that we've rolled out since we've enabled some of this type of capability."
Given the increasing number of internet-connected devices predicted to be used for making payments in the future, including virtual reality headsets, Wiesman also said it's important for organisations to understand how to assess trust.
"Clearly we're headed down a path of more and more types of devices facilitating commerce. I think that if we don't figure out the right way to [enable payments through new devices] it's going to be a lot more challenging because the people who are making the purchases are becoming a little more distant from the process. It's really that intelligent device that's facilitating the transaction," he said, adding that Mastercard acquired biometrics specialist NuData Security earlier this year, which will enable the organisation to better understand emerging devices.
"By moving some of our capabilities further out closer to where the purchase starts, we take the idea of collecting that data and that analysis where it happens and we feed that into the authorisation process that we have today. We could essentially layer things on top of each other to enable smarter payments."
Data: A multifaceted challenge
Despite improvements in data management and analytics tools, decreased costs in processing data, and increased availability and experience of experts in the field, Suma said there are challenges around data that financial services organisations have to confront, such as the "velocity, volume, and variety" of data that's available.
"Harnessing that data as a programmatic input into ML/AI based fraud analytics analysis is a key challenge," Suma said.
Additionally, Chenis said that while advances in cloud technologies are opening up opportunities for organisations willing to trust cloud providers with their data, many of them that operate in highly-regulated industries are keeping at least some of their data in non-cloud environments, which brings forth another challenge: Combining data sources from multiple locations, both and private, without having to move the data.
"This opens up further discovery opportunities and potential added commercial opportunities for enterprises to add third-party shared data into their own ML/AI processes," Chenis said.
Suma also identified data governance as a challenge especially as organisations seek to connect disparate data sources, saying that increasing appointments of chief data officers within organisations is indicative of this challenge.
"Regulations around data privacy also paint an evolving picture that must be incorporated into the deployment of ML/AI tools in fraud prevention strategies," he said.
The growing velocity, volume, and variety of data also presents opportunities to significantly improve intelligence. For example, Weatherhead said organisations have started using social network analytics, combining data about the customer with data about the customer's network.
"It's linking this customer at a bank who doesn't seemingly have a relationship with another customer in the bank on the face of it, but if you start looking at other data attributes like email addresses and phone numbers and things like that, you might see that there's some commonality and it's linking it together. Then that network is used to apply models of statistics on top," Weatherhead told ZDNet.
"The difference is that rather than just profiling that one customer in isolation and identifying anomalies with that one person, you're looking at a network and that network approach is really important for picking up collusion, picking up identity manipulation, and also mule-type activity."
One of the biggest challenges -- and opportunities -- around data is the actual sharing of it.
Weatherhead believes combating financial crime requires an integrated and collaborative approach, where financial organisations globally share data and intelligence to improve industry response to increasingly sophisticated criminal activities.
She said BAE has data-sharing arrangements in countries like Malaysia, which allows the organisation to boost its capability to more accurately predict, detect, and halt fraudulent activities.
"Our technology is used by the insurance services of Malaysia; it takes all the claims data from insurers in Malaysia, and our technology sits on top of that pool of data to identify fraud. It's much more effective if you've got a larger set of data; you'll be able to identify trends particularly those fraudsters that are targeting multiple organisations and spreading out their attack so it's harder to detect," she said.
Wiesman also communicated the importance of a collaborative approach, saying that "fraudsters are all really smart; they're well-financed, they're motivated, and they're really good at poking and prodding to figure out where there are gaps."
"It seems like we're always trying to figure out how to stay a step ahead and try to manage where we think the next thing might ultimately be. That's going to be an ongoing challenge for quite some time," he said, agreeing that increased collaboration and data-sharing would help organisations stay ahead.
However, Suma said competitive concerns -- for example, sharing sensitive data with competing organisations -- ultimately limit the extent of collaboration.
"At ACI, for example, our merchant customers collaborate via our cloud-based fraud solution for ecommerce, enabling parties to share fraud trends across retail segment or geographies, as opposed to hard data. Internet of Things technology will provide additional data points that can be consumed through ML/AI tools to monitor transaction streams of payments and detect anomalous behavior that could be indicative of a financial crime," he said.
"This will become an increasing necessity as global fraudsters work to exploit the weakest points in a fraud prevention program."
Chenis said tools are evolving to enable more cross-organisation collaboration around sensitive or controlled data, initially targeting enterprises with proper access controls and security ownership.
"It is natural to assume this evolution will continue to enable consortium collaboration where independent agencies would be able to collaborate across the network, both internally and externally, to a given enterprise -- all while maintaining data confidentiality and control," he said.
Related Coverage
Brazilian banks lead in artificial intelligence planning
The number of institutions that see AI as important to their strategies is higher than other countries - but number of real case studies is still small.
Singapore puts fintech in spotlight with AI investment, global partnerships
Monetary Authority of Singapore sets aside S$27 million to drive the adoption of artificial intelligence in the financial sector and inks global partnerships to facilitate fintech innovation.
Video: Why financial services companies are investing in blockchain tech (TechRepublic)
Jitterbit's Simon Peel explains why banks are investing in the blockchain, and how distributed ledger APIs enable financial services innovations.
The 10 companies that pay AI engineers the most (TechRepublic)
US companies have invested $1.35 billion in AI talent, according to new data from Paysa. Here's where AI professionals can make the most money.