Fighting bias in AI starts with the data

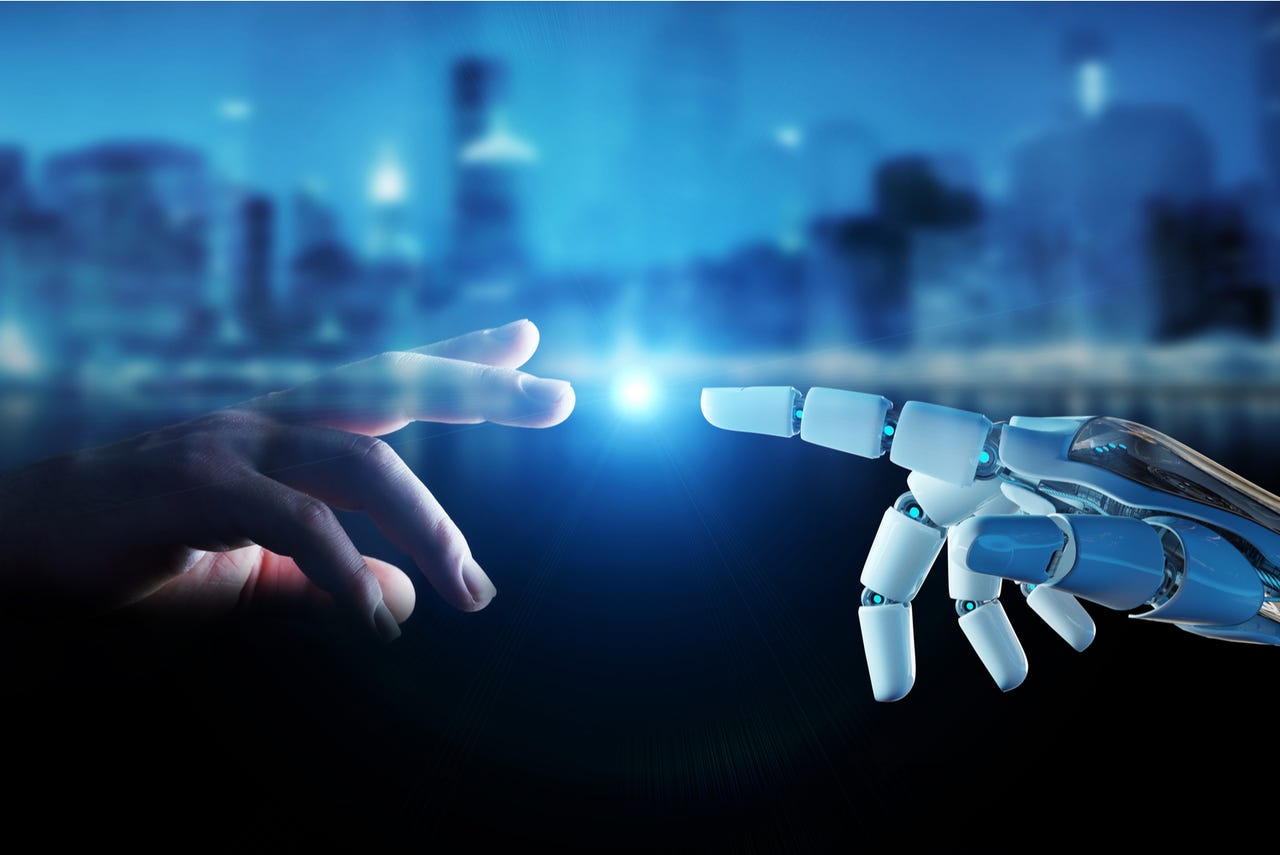
The push to deliver unbiased and responsible artificial intelligence is admirable, but there are many roadblocks to overcome. Chiefly, AI is only as fair as the data that goes into it.
In light of the slow progress addressing AI bias and unfairness, business and technology leaders may be finally arriving at a consensus that they need to concentrate on more "responsible" approaches to AI. A recent survey of 504 IT executives, released by Appen and conducted by The Harris Poll, finds heightened concern about the data that is increasingly driving decisions about customers, markets, and opportunities. It also hints at recognition by both types of leaders that the data they have tends to be problematic, wreaking damage to people, communities, and businesses.
Artificial Intelligence
Even among the most proactive companies, a majority are not yet taking steps to wring out bias from AI, a 2021 survey by McKinsey found.
For example, 47% of respondents, less than half, reported that they scan training and testing data to detect the underrepresentation of protected characteristics and attributes.
The same percentage reported that data professionals in their organization actively check for skewed or biased data during data ingestion. Only 36% reported that data professionals actively check for skewed or biased data at several stages of model development.
The Appen survey shows that sourcing quality data is an obstacle to creating AI. A majority, 51%, said data accuracy is critical to their AI use case -- but only 6% reported achieving full data accuracy (exceeding 90%). "Many are facing the challenges of trying to build great AI with poor datasets," the survey's authors state. "To successfully build AI models, organizations need accurate and high-quality data. Unfortunately, business leaders and technologists report a significant gap in the ideal versus reality in achieving data accuracy."
Also: AI ethics should be hard-coded like security by design
Still, the Appen survey found that companies are shifting their focus to "responsible" AI. "Data ethics isn't just about doing the right thing," the survey's authors point out. "It's about maintaining the trust and safety of everyone along the value chain from contributor to consumer." Almost all, 93%, said they believe they need to deliver responsible AI. They report focusing on improving the data quality behind AI projects to promote more inclusive datasets that will help eliminate bias and unfairness. Eight in 10 respondents described data diversity as extremely important or very important, and 95% agreed that synthetic data will be a key player when it comes to creating inclusive datasets.
Easier said than done, of course; at least 42% of technologists responding said the AI life cycle data-sourcing stage is very challenging. In addition, 90% reported they are retraining their models on at least a quarterly basis.
Also: AI projects grew tenfold over the past year, survey says
This also calls for keeping humans in the AI loop. There's a strong consensus around the importance of human-in-the-loop machine learning, with 81% stating it's very or extremely important and 97% agreeing that human-in-the-loop evaluation is important for accurate model performance.
Interestingly, the gap between data scientists and business leaders is slowly narrowing year over year when it comes to understanding the challenges of AI. "The emphasis on how important data, especially high-quality data that match with application scenarios, is to the success of an AI model has brought teams together to solve for these challenges," the survey's authors point out.