Five ways to use data to make better business decisions
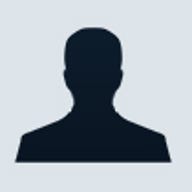
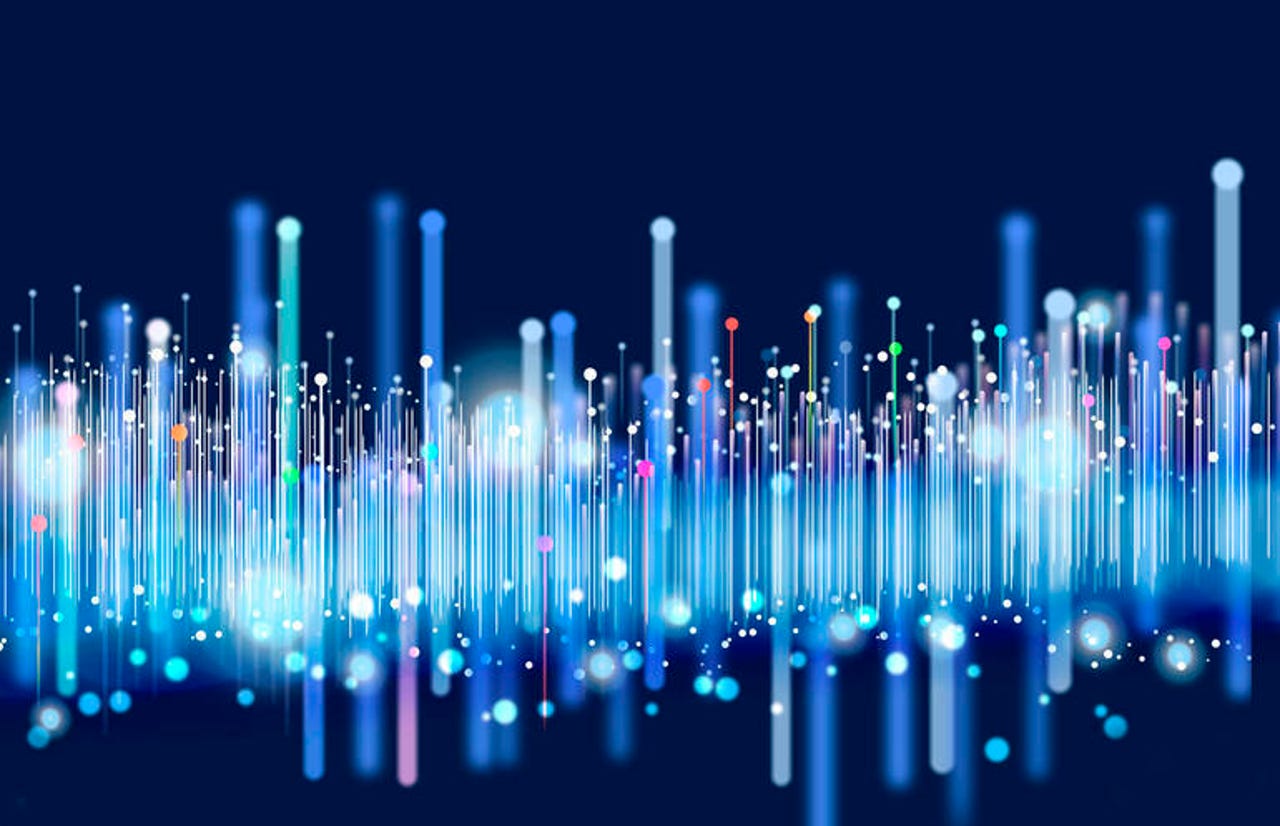
As we continue to navigate our way through the COVID-19 crisis, data quality and sound business practices for using data are more important than ever. They're critical in the trials of drugs and vaccines; in predicting where and when the next disease outbreak will occur; in assessing the availability of resources and personnel; and in evaluating economic impact.
In all of these endeavors, analytics, artificial intelligence (AI), and machine learning (ML) play key roles -- but data quality and sound business data practices are equally important.
SEE: Electronic Data Disposal Policy (TechRepublic Premium)
On the IT side, companies achieve quality data by using a master data management (MDM) methodology that defines how data is collected, aggregated, matched, consolidated, quality-checked and distributed.
There are numerous tools and automation solutions available for master data management. These tools reduce the tedium of manually culling through data, normalizing it, deciding what should go with what, and who gets which data. The tougher part of the job is enacting sound business data practices which will lay the groundwork for the data preparation that IT does with MDM.
Here are five business data practices that are vital for any organization striving to deliver the highest-quality data for decision making.
SEE: Report: SMB's unprepared to tackle data privacy (TechRepublic Premium)
Digitize dark data
Dark data is data that is available but never used. It can exist in the form of a paper-based document created by the business years ago, or as sensor-generated Internet of Things (IoT) output that isn't used.
IBM estimates that 90% of the data companies possess never gets used, and is dark data. While much of this dark data may turn out to be superfluous, some of it isn't. Organizations need to review this data, determine what they want to eliminate, and then digitize the data that they want to keep. This digitized data can then be used in decision-making so it doesn't remain as a non-digitized wasted data asset.
Determine data value in decision-making
Even though data might be digitized, it still may not be relevant for decision-making. If the data isn't valuable, it should be considered for elimination.
Deciding which data to keep is a balancing act. There is data that isn't important today but could become valuable at a later date. However, there is other data (IoT 'jitter', for example, or memos about a company holiday party 20 years ago) that most likely won't ever be relevant to decision-making and should be eliminated.
Be able to aggregate different data types
Master data management frequently focuses on normalizing or consolidating disparate data fields from different systems that refer to the same piece of information. However, there is also the need to aggregate unlike types of data, such as aggregating a weather report with photos or videos of a storm system.
Data aggregation is most successful when business use cases are clearly identified, along with all of the data and data combinations that are needed for decision making.
SEE: Resource and data recovery policy (TechRepublic Premium)
Focus on silo-busting
With the growth of citizen development and separate IT budgets in business user departments, it's more difficult for IT to know where packets of underexploited data might reside, and how to bring these data troves into a central data repository so that everyone in the business can use them. For example, a salesperson would be very interested in the returns of a particular product that a valuable customer made in recent months because of repeated product failures. But if the salesperson doesn't have access to the returns and rework data from manufacturing or engineering, he or she isn't going to know about it. This could become an embarrassing call to pitch that same product to the customer.
One way that IT can track new and existing data assets throughout the organization is with an asset management system that resides on corporate networks and that can detect new data, systems and servers. If standalone business silos are identified, IT or a corporate data officer can get together with the business department hosting it and assess if there are other uses throughout the company for the information. The end objective should always be to create as holistic and transparent a picture of the enterprise as possible.
Educate management
Data digitization and preparation projects don't mean much to anyone outside of IT. This is because they are preparatory IT projects that don't produce deliverables seen by business decision-makers.
The question that IT must answer early on is whether management is willing to fund data preparation processes that don't yield tangible business results.
Educating (and reminding) upper management of the importance of high-quality data for high-quality business decisions is an ongoing process. IT should take every opportunity to do this and ensure that the necessary executive sponsorship for data preparation is in place.
Also see
- How to become a data scientist: A cheat sheet (TechRepublic)
- Top 5 programming languages data admins should know (free PDF) (TechRepublic download)
- Data Encryption Policy (TechRepublic Premium)
- Volume, velocity, and variety: Understanding the three V's of big data (ZDNet)
- Big data: More must-read coverage (TechRepublic on Flipboard)