Google’s DeepMind follows a mixed path to AI in medicine
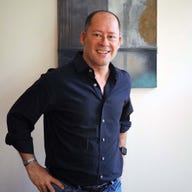
There are many headline studies about artificial intelligence making strides in medicine, but the reality can be somewhat more prosaic. What gets used in hospitals and clinicians' offices may be much simpler, and a lot less like AI than you would think.
Two research papers this week from the DeepMind unit of Google show a disparity between bleeding-edge tools of deep learning and the humble use of software to automate the day-to-day tasks of doctors.
In the latest issue of Nature magazine, DeepMind researchers published the results of a deep learning project that can predict kidney failure of patients in the hospital up to 48 hours before the onset of symptoms, with far greater accuracy than existing computer programs for such predictive uses.
Also this week, the DeepMind team published the results of a third-party survey of the use of a computer program called "Streams," which uses no artificial intelligence but which can be useful to physicians for things such as being alerted to warning signs about a patient.
The first project, the deep learning one, has some ways to go to be put into practice, while the Streams software is already in use by doctors and hospital staff.
A comparison of the old way doctors got alerts, top, and the new "digital pathway" built on DeepMind's "Streams" app, on the bottom. The main innovation is simply to let doctors get alerts on a phone.
The first paper, "A clinically applicable approach to continuous prediction of future acute kidney injury," deals with the "adverse events" that happen after a patient is already in the hospital. One of those events is "acute kidney injury," or AKI, which is defined as "a sudden and recent reduction in a person's kidney function," according to Think Kidneys, a website set up with the help of the UK's National Health Service. The condition can come on as a result of severe dehydration or as a side effect of prescription drugs, among other reasons.
As DeepMind notes in a blog post, the condition, which can be fatal, affects one in five patients in hospitals in the US and the UK, and 30% of those cases could be prevented with proper detection before the condition worsens. AKI and other conditions that come on suddenly are part of the challenge of machine learning to predict what can go wrong, which has been a devilish problem.
As the authors write, "Few predictors have found their way into routine clinical practice, because they either lack effective sensitivity and specificity or report damage that already exists."
Hence, DeepMind partnered with the US Department of Veterans Affairs to see if a neural network could predict the instances of AKI from time-series data. They compiled a dataset of digital health records for over 700,000 patients in VA hospitals across five years with six billion entries in the dataset and 620,000 "features" that could be relevant to AKI. The data was labeled, meaning that the computer was given information about which patients ended up developing AKI.
Primers
It was all done with state-of-art neural networks of the "recurrent neural network," or RNN, variety, including a "deep residual embedding" component that "learns" a "representation" of the AKI factors. The authors emphasize this was a single "end-to-end" network, requiring no special domain-specific pre-training of the network.
The authors report the network blew away results from traditional prediction methods, such as what are known as "gradient-boosted trees," where the risk factors had to be explicitly coded into the algorithm, rather than discovered in the data, as with the RNN model. Their conclusion is that their work opens the way to more predictive deep learning studies of patient degradation.
But there are impediments to be overcome. The data are not balanced by gender and ethnicity, and they need to elucidate confounding factors: "Future work will need to address the under-representation of sub-populations in the training data," they write, "and overcome the effect of potential confounding factors that relate to hospital processes."
The second paper, "A Qualitative Evaluation of User Experiences of a Digitally Enabled Care Pathway in Secondary Care," published in the Journal of Internet Medical Research, is about the actual use of the Streams software. Streams is a mobile app on the iPhone used by doctors at the Royal Free Hospital in London. It is used to send alerts on the phone to doctors that will warn them of a rise in serum creatinine, a waste product in the blood that is one of the main indicators of the onset of AKI. The creatinine levels of patients are continuously monitored, and warning signs are sent to a special team so that they can prioritize patients to look in on who is at risk. The software has been in use since May of 2017 at the hospital.
Streams, DeepMind researchers note their blog post, don't "use artificial intelligence at the moment." Its function is to be a mobile extension of the hospital information system. The point is to replace the task of a physician sitting down at a desktop computer to assess creating levels in tests and instead let them know proactively if levels are changing and require attention.
The novel recurrent neural network DeepMind constructed to process billions of data points for early detection of acute kidney injury. It's an "end-to-end" system, they emphasize, requiring no pre-training of the network.
The paper, compiled by DeepMind staff in collaboration with researchers from the University College in London and the UK's National Health Service, interviewed clinicians using the app from February of 2017 to May of 2018. They quote extensively from the interviews, and the feedback seems fairly positive.
One member of the nephrology team says, "Being able to look up the blood results for anyone in the hospital wherever you are is unparalleled." Another hospital staff member reported checking the app like checking email, saying, "within five minutes or so I could easily flick through the alerts and [...] identify which ones I needed to see."
It wasn't all positive, however. One staff member quoted complaints of "noise" in the app from lots of false alarms. Another complained of anxiety created by getting a lot of alerts when it's not clear which clinician is responsible for responding to the alerts.
The authors of the study conclude there is a "positive impact" of the software for patient care," such as an enhanced ability to "intervene in the treatment of deteriorating patients more quickly." They also acknowledge shortcomings, such as "anxiety associated with increasing numbers of priority patients and information overload, in part exacerbated by false-positive alerts."
The app, while apparently useful, didn't have a statistically significant impact on outcomes, the study found. A companion paper, published this week in Nature's sister pub, NPJ Nature Medicine, notes that it's not enough to just have an app: "Our evaluation also helps to clarify why e-alerting alone might fail to improve outcomes; we demonstrate the need to consider the organizational as well as the technical aspects of digital interventions by coupling the alerting system to specific management pathways."
There you have it: One cutting-edge piece of software that seems to increase early detection of deterioration, and potentially saves lives, but can't be deployed yet; and another piece of software that is already helping doctors but isn't a silver bullet for care. Two worlds, for now, separate in their practical appeal.
DeepMind researchers suggest in a companion blog post that they endeavor to integrate those two worlds at some point: "The team now intends to find ways to safely integrate predictive AI models into Streams in order to provide clinicians with intelligent insights into patient deterioration."