MIT's latest breakthrough? Getting AIs to explain their decisions

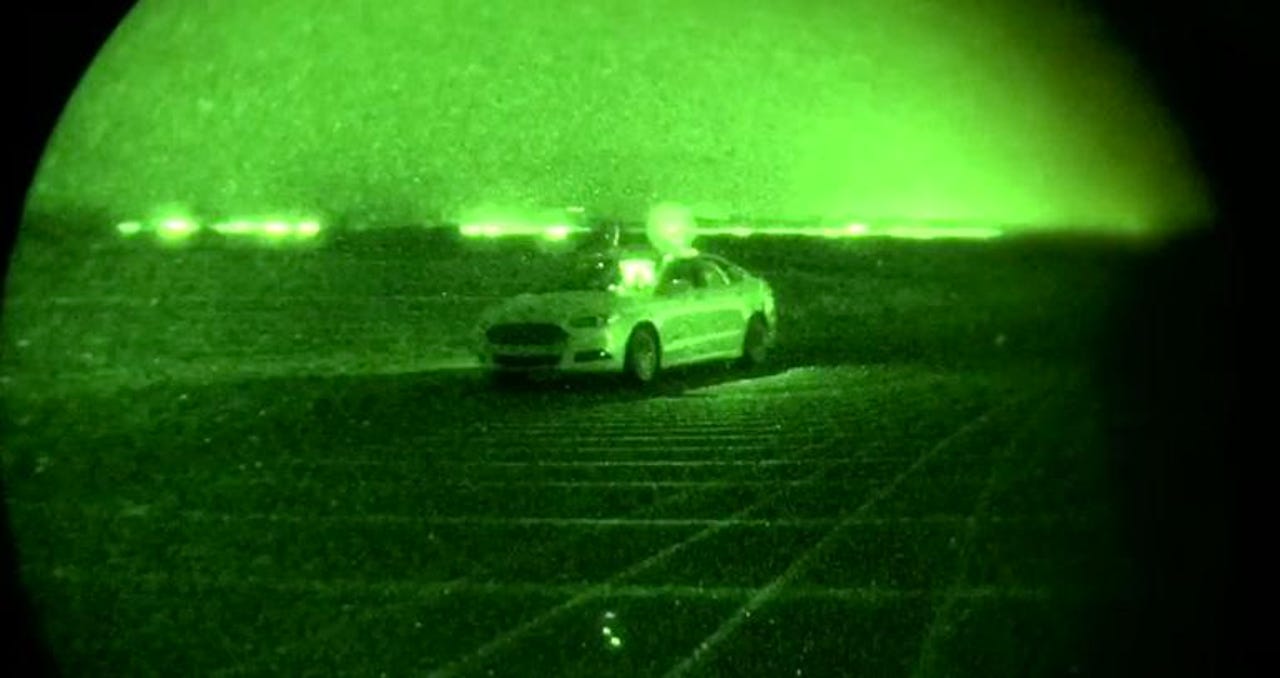
Knowing why a machine is making a certain decision becomes especially important in, say, critical situations involving autonomous vehicles.
As humans grapple with ethical questions about what artificial intelligence should do in life-and-death situations, researchers at MIT have devised a way for machines to explain their decisions.
The method, outlined in a new paper, could be as important for the adoption of artificial intelligence technologies as actual breakthroughs in AI enabled by deep learning and neural networks.
As MIT points out, while neural networks can be trained to excel at a specified task, such as classifying data, researchers still don't understand why some models work and others don't. Neural nets are effectively black boxes.
Not knowing why a neural net can identify animal types in an image might not be a problem, but it becomes one when a machine is making life-or-death decisions, say, as a self-driving vehicle confronted with a choice to save others at the expense of passengers, or a AI helping doctors diagnose illnesses.
Researchers at MIT's Computer Science and Artificial Intelligence Laboratory (CSAIL) tackle this conundrum using a new technique that not just trains neural networks to make predictions, but also to explain why it made a certain decision.
Greater transparency of an AI model's decision-making could help overcome reservations about using AI in the medical profession, argues Tao Lei, an MIT graduate student and first author on the new paper.
"In real-world applications, sometimes people really want to know why the model makes the predictions it does. One major reason that doctors don't trust machine-learning methods is that there's no evidence," he said.
The researchers tested their system on a beer review site in the task of sentiment prediction. The site uses both five-star ratings and written reviews. The system was able use the training data both to accurately predict a beer's rating and identify a phrase, such as "a very pleasant ruby red-amber color" as the rationale for the decision.
MIT notes that the system's agreement with human reviews was 96 percent for appearance, 95 percent for aroma, and 80 percent for palate.
The researchers have also applied the model to "thousands of pathology reports on breast biopsies, where it has learned to extract text explaining the bases for the pathologists' diagnoses", according to MIT.