Nvidia DGX-2 review: More AI bang, for a lot more bucks
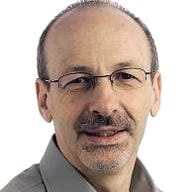
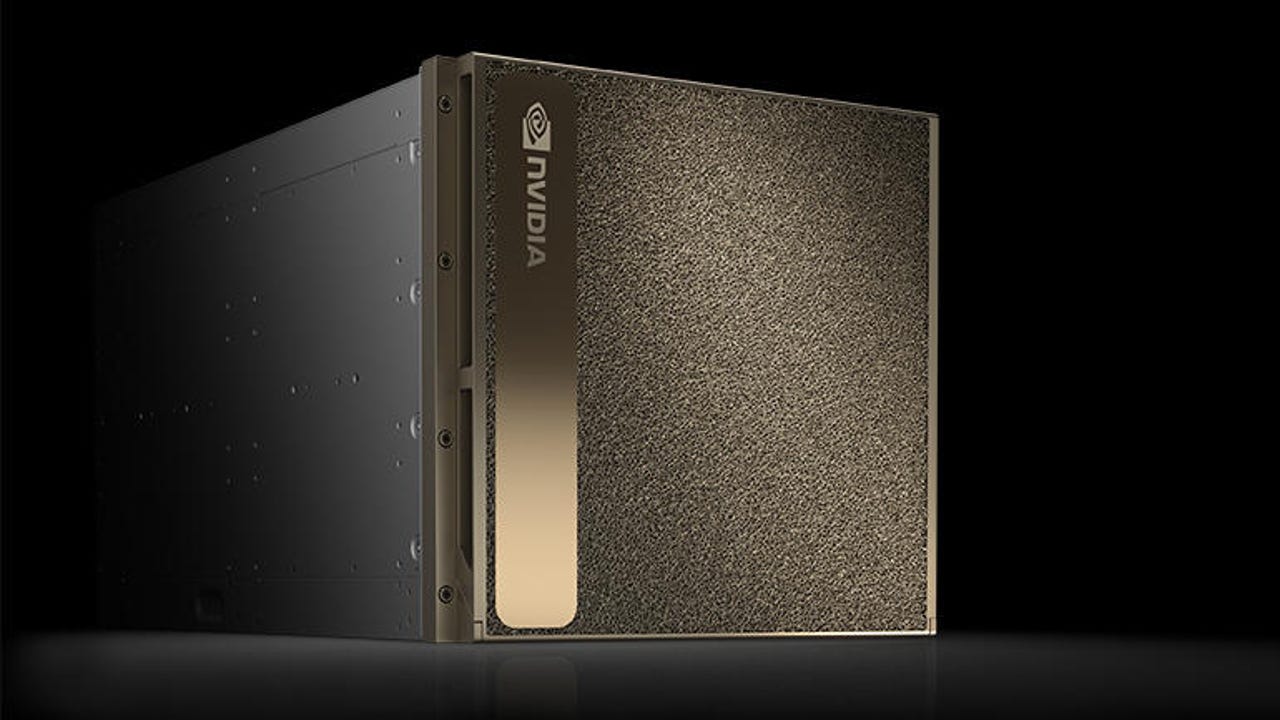
For $400,000, you could get around 400 iPhone X handsets, 300 Surface Pro laptops, or 11 Tesla Series 3 electric cars. But it would take the whole $400K and more to get your hands on just one Nvidia DGX-2 server, which is billed as "the world's most powerful AI system for the most complex AI challenges".
But does the DGX-2 live up to that claim -- and is any server really worth such an eye-watering price tag?
DGX continued
In order to answer those questions you have first to understand that the DGX-2 isn't the first off-the-peg Nvidia server to be targeted at AI. That honour goes to the DGX-1, based on a mix of Intel Xeon processors paired with Nvidia's own AI-optimised Tesla V100 Volta-architecture GPUs. The DGX-2 continues that approach, but instead of eight Tesla V100s joined using Nvidia's NVLink bus, the DGX-2 comes with 16 of these mighty GPUs connected using its more scalable NVswitch technology. According to Nvidia, this setup allows the DGX-2 to handle deep learning and other demanding AI and HPC workloads up to 10 times faster than its smaller sibling.
Although it was announced at the same time as the DGX-1, it has taken a further six months for the larger model to appear. One of the first to make it to the UK was installed in the labs of Nvidia partner Boston Limited. They asked if we'd like to have a look: we did, and here is what we found.
The DGX-2 'unboxed'
The DGX-2 is big and hides behind an imposing gold crackle-finish bezel.
As well as performance, size is a big differentiator with the DGX-2, which has the same crackle-finish gold bezel as the DGX-1 but is physically a lot bigger, weighing in at 154.2kg (340lbs) compared to 60.8kg (134lbs) for the DGX-1 and consuming 10 rack units instead of 3.
This picture shows the back of the 10U DGX-2 chassis with slots for two GPU trays (just one in situ), with empty server and PCIe tray slots below, plus three hot-swap power supplies on either side.
Special attention is needed to power and cooling, especially in a mixed rack. Here, together with a few stray network cables, is how power is fed to the rack in the Boston Labs.
It's also worth noting that the DGX-2 needs a lot more power than its little brother, requiring up to 10kW at full tilt, rising to 12kW for the recently announced DGX-2H model (about which more shortly). The picture below shows the power arrangements at Boston needed to keep this little beast happy. Cooling, similarly, will need careful consideration, especially where more than one DGX-2 is deployed or where it's installed alongside other hardware in the same rack.
Distributing that power is a set of six hot-swap and redundant PSUs that slide in at the rear of the chassis along with the various modules that make up the rest of the system. Cooling, meanwhile, is handled by an array of 10 fans located behind the front bezel with room on either side for 16 2.5-inch storage devices in two banks of eight.
With 8 NVMe SSDs, the DGX-2 comes with 30TB of storage, leaving eight bays free for expansion.
Nvidia includes eight 3.84TB Micron 9200 Pro NVMe drives as part of the base configuration, equating to just over 30TB of high-performance storage. This, however, is mostly to handle local data, with additional storage on the main motherboard for OS and application code. It also leaves eight bays empty to add more storage if needed. In addition, the DGX-2 is bristling with high-bandwidth network interfaces to connect to even more capacity and build server clusters if required.
The Intel bits
A pair of 24-core Xeon Platinum processors, 1.5TB of RAM and a pair of NVMe storage adapters are configured on the DGX-2 motherboard.
Pull out the main server tray and inside you find a conventional-looking Intel-based motherboard with two sockets for Xeon Platinum chips. On the system we looked at these were 24-core Xeon Platinum 8168 processors clocked at 2.7GHz, although Nvidia has since announced the DGX-2H model with slightly faster 3.1GHz Xeon Platinum 8174 processors along with newer 450W Volta 100 modules. This comes at the expense of requiring a lot more power (up to 12kW) and will probably add to the overall cost, although at the time of writing the price of this new model had yet to be confirmed.
Regardless of specification, the Xeon processors sit in the middle of the motherboard surrounded by 24 fully populated DIMM slots, giving buyers an impressive 1.5TB of DDR4 RAM to play with. Alongside this are a pair of 960GB NVMe storage sticks configured as a RAID 1 array both to boot the OS (Ubuntu Linux) and provide space for the DGX software stack and other applications.
The usual USB and network controllers are also built in, with two RJ-45 Gigabit ports at the back -- one for out-of-band remote management and the other for general connectivity. One of the two available PCIe expansion slots also comes ready fitted with a dual-port Mellanox ConnectX-5 adapter that can accommodate Ethernet transceivers up to 100GbE for additional network bandwidth.
As well two built-in Gigabit Ethernet ports, a Mellanox PCIe adapter provides two more Ethernet ports that can take 10-100GbE transceivers.
The second PCIe expansion slot is usually empty but even more connectivity is available courtesy of the separate PCIe tray that sits just above the server motherboard. This adds a further eight PCIe interfaces filled, again, with Mellanox adapters that can be used to connect to clustered storage using either 10GbE Ethernet or InfiniBand EDR 100 transceivers.
A further eight Ethernet or Infiniband ports are available via the PCIe tray.
The Nvidia parts
And now the bit you've all been waiting for -- the 16 Nvidia Tesla V100 GPUs which, partly because of of their large heatsinks (see below), have to be split across two baseboards.
As a reminder, this is what a Tesla Volta 100 module looks like:
And this is what eight Volta 100 modules look like when installed inside one of the GPU trays of a DGX-2:
The 16 Tesla V100 GPUs are divided between two baseboards along with the NVswitch hardware needed to link them together.
The GPU boards also hold the NVswitches that need to be physically joined in order for the Volta 100 modules to communicate and function as a single GPU. This is accomplished by attaching two custom-designed backplanes to the rear of the baseboards once they have been pushed into the chassis.
The NVswitches on the two GPU baseboards are physically joined by these fiendish-looking backplanes, which attach at the rear.
The Tesla V100 GPUs themselves are much the same SXM modules as those in the latest DGX-1. Each is equipped with 32GB of HBM2 memory per GPU, so with sixteen installed there's double the GPU memory -- 512GB -- altogether.
Each GPU also has 5,120 CUDA processing cores as well as 640 of the more specialised AI-optimised Tensor core. Multiplied by sixteen, that gives 10,240 Tensor cores in total and a whopping 81,920 CUDA equivalents. All of which makes for a lot of processing power, which is further enhanced by the interconnect bandwidth of 2.4TB/sec available from the NVSwitch technology with capacity to scale even further in the future.
Performance to go
So much, then, for the hardware. In addition to this you also get a whole stack of preinstalled AI tools ready to power up and begin working.
When reviewing a server, it's at this point that we would normally start talking about performance and the results of tests that we would typically run to see how it stacks up. However, running benchmarks on the DGX-2 is a far from trivial task which, given the type of deep learning and other HPC workloads involved, would require lengthy sessions over several days. So instead we'll have to rely on Nvidia's claims, along with feedback from the experts at Boston.
To this end, the headline figure for the DGX-2 is an impressive 2 petaFLOPS (PFLOPS) of processing power delivered primarily by the Tensor cores to handle mixed AI training workloads. This figure rises to 2.1 PFLOPS on the DGX-2H using faster 450W Tesla V100 modules.
To put that into perspective, this processing power enabled the DGX-2 to complete the FairSeq PyTorch benchmark in just 1.5 days -- that's 10 times faster than the 15 days needed for the same test on the DGX-1 just six months earlier. Moreover, Nvidia reckons that to get the same results using x86 technology would require 300 dual-socket Xeon servers, occupying 15 racks and costing around $2.7 million.
SEE: How to implement AI and machine learning (ZDNet special report) | Download the report as a PDF (TechRepublic)
All of which makes the DGX-2 seem like a bargain at around $400,000 (or the equivalent in GB£), even when you add in the cost of support -- which, in the UK, starts at around £26,000 (ex. VAT) per year. Despite the high price tag, companies already investing in AI will find this very affordable compared to the alternatives, which include renting compute time in shared data centres or the cloud. Nvidia is also keen to stress that the DGX-2 can also be used to handle less exotic HPC workloads alongside its AI duties.
Bear in mind also that, although the DGX-1 and DGX-2 are breaking new ground, alternatives are on their way from other vendors. Not least SuperMicro, which on its website already lists a server based on the same Nvidia HGX-2 reference model as the DGX-2. Others, such as Lenovo, aren't far behind and these alternatives will inevitably work to drive prices down. We'll be following these developments throughout 2019.
RECENT AND RELATED CONTENT
IBM, Nvidia pair up on AI-optimized converged storage system
IBM Spectrum AI with Nvidia DGX is designed for AI and machine learning workloads.
MLPerf benchmark results showcase Nvidia's top AI training times
For the first release of MLPerf, an objective AI benchmarking suite, Nvidia achieved top results in six categories.
Nvidia aims to run neural nets faster, more efficiently
As data gets bigger and models grow larger, deep learning is once again "completely gated by hardware." At the VLSI Symposia, Nvidia suggested some ways to address this problem.
Nvidia unveils the HGX-2, a server platform for HPC and AI workloads
The platform's unique high-precision computing capabilities are designed for the growing number of applications that combine high-performance computing with AI.
GPU computing: Accelerating the deep learning curve
To build and train deep neural networks you need serious amounts of multi-core computing power. We examine leading GPU-based solutions from Nvidia and Boston Limited.
AI skills reign supreme in the fastest-growing jobs of the year (TechRepublic)
Six out of the 15 top emerging jobs in 2018 were related to artificial intelligence, according to LinkedIn.
Nvidia outlines inference platform, lands Japan's industrial giants as AI, robotics customers (TechRepublic)
The news highlights Nvidia's traction in AI and the data center.