SAS talks sentiment analytics and data limits
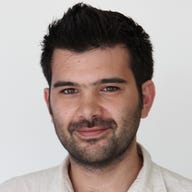
SAS has over 30 years' experience in the data analytics and business intelligence market and is one of the longest standing independent companies in the sector. Its products are used in more than 100 countries across a variety of sectors and its customers include 93 of the top 100 companies in the 2010 Fortune Global 500 list.
With data collection and analysis becoming ever-more important to businesses, ZDNet UK spoke to Radhika Kulkarni, vice president of advanced analytics R&D at SAS, at the company's annual analytics event in Copenhagen. She spoke about where the company's R&D is headed, the perils of sentiment analysis and the challenge of storage limitations.
Q: As a leader of one of SAS's R&D departments, what areas are you focusing on?
A: Distributed computing and in-database computation are two areas where we're spending quite a bit of energy.
In my keynote, I talked about one particular big milestone where we went from having software running on one host to multiple hosts. I think that we're now at the second huge wave of a similar change, where it's not just multiple hosts or operating systems. We want to take the computations down to an even lower level — to the database — for efficiency because now the volume of data that resides in the databases is humongous.
For example, [there are cases when you] have petabytes of data, with many millions of customer records in the database, but if you want to score them and create prediction values for them, then you couldn't do the scoring inside the database. You would need to bring all of that data back up to temporary storage, do the scoring, then push it back.
This presents its own problems: Firstly, that's a lot of I/O that gets spent, and secondly, there may be some security issues because you would not want the data to be copied.
The sheer amount of data involved — the numbers — are quite mind boggling. Do you have specific security considerations?
We do. More and more, we're seeing that there's a lot of IT departmental involvement in the purchase of software, so it's not just enough for us to convince the analytical people that we have the best statistical algorithms. We need to be able to convince the IT departments of the major corporations.
Any time you get into an enterprise situation, that's always going to be the case. There's data sharing; there's security concerns. "What is it you don't want to leak?": stuff like that. And so, being able to partner with IT is a big factor.
More and more, we need to be able to convince the IT people that we understand and recognise the challenges and that we're making sure that we provide for those. Now our job has become convincing two different organisations in any company as well as the analytical people, the IT department and the business unit — and a lot of time you have analytical people that work across the business units. They might know you from your professional organisations and say 'these algorithms make sense, but is it solving my business problems?'. You need to be able to talk to the businesses.
You've spoken about the overlap between the amount of data that will be created [in 2011] and the amount of available storage. Will you see the same issues with data overlap as you referred to from 2007? Do you have an R&D wish list, or indeed, a planned solution for this problem?
The wish list for the research areas I think about is: "How do you decide which data to throw away?"
I don't think the answer is readily available yet. It depends on the problem you're trying to solve, but if you don't know what problem you're going to solve, how do you solve it?
There's duplicate information you can throw away — that's for sure. De-duping is going to be a big area for us. But what's extraneous? How do you decide? I don't know the answer to that.
We're absolutely going to have an overlap, so data storage and data management are a big part of what we do. All of the analytics that we do relies on the data being valuable and relevant, not just data for data's sake. If you're looking in the wrong haystack, you'll never find the needle.
Other vendors are integrating their platforms to make business intelligence (BI) products accessible from mobile devices. Is that a demand that you're seeing from customers? You addressed developing for mainframes and then becoming more platform agnostic in your keynote. Is that extending into the mobile arena?
Yes, that's a new area for business, where you need to encapsulate the questions in a way that can be displayed easily, not just for non-analytics user but also on a mobile device, for instance. One example that doesn't happen a lot in the US, but more so in India, is that as you enter a new mobile phone service area you'll get a beep with some [marketing] offer.
Those are all taking advantage of the mobile device to make you an offer. As you have devices which have a lot more real-estate to show pictures, you can even start to find and display graphical trends, which is great for analytics. All things which are within reach now. The mobile devices have become adept at that kind of application, and the connectivity [is there now]. We have a team that looks at BI reporting in Flash applications, that sort of thing.
One of the interesting areas is that answers can be displayed in multiple ways and generated from lots of different, potentially complex methods. If it's fast enough and you get your result, you don't care about the method. The value of the result depends on what happened before you got the number. There's a lot of computation behind the scenes, but you don't know it.
From a consumer point of view, most people don't realise just how much data is there, how much is collected, how much is acted upon...
Yes, exactly. Here's an example everyone can relate to: I phone my bank when I'm travelling to tell them not to stop my card, which is something I did last time I went to India. I went to a jewellery store and bought one item of jewellery, and it went through. I went to another one, and it went through too.
Within an hour I went to another jewellery store and bought another [item of jewellery] and they stopped it and said: "you need to call your credit card company". The company hadn't queried the first two or three expensive items when it was happening, but in the next one [from the bank's point of view] it was "let's just check on the off-chance". It wasn't that they were trying to stop it, it was just that the bank wanted to make sure it was me. Even after calling the bank before travelling, I could have lost my card. It's irritating when it happens, but then you think "hey, it's protecting me".
From the analytic perspective, the first few times it worked because of historical data. They probably had a flag that said I was going to be in India, but the fact that the third purchase was stopped meant the company also knew that the last two transactions were so close to each other. If you think about that, the amount of intelligence it's getting is large, but it needed it all to make a decision.
Some people may have concerns over the mass harvesting of data. For example, SAS does sentiment analysis. Do you see any concerns in using people's data in this way?
Sentiment analysis can actually be done on two different types of source: public and private. If you're a hotel chain and have a website where people comment, that's your data. Then there's public data.
Well, it's public data. Take a bank or hotel chain, for example, that comes to us and says it would like us to analyse the sentiments that we are noticing from the past two years of data. We could analyse that, and as it's public data, there's no issue. We could share our findings with the bank or hotel. It's public data, and I don't see a problem with the analysis. A lot of companies are seeing value in being able to monitor that public data.