Stripe banking on machine learning to curb payments fraud
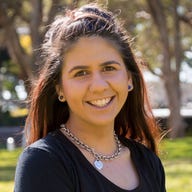
Globally, billions of dollars are lost to fraud every year, and with the growth of ecommerce business models over the past five years, payments startup Stripe is putting its transaction-based insights to good use in an effort to block fraudulent activity from its platform.
Featured
Speaking with ZDNet, Stripe's head of machine learning Michael Manapat said that internet businesses are in a uniquely liable situation of having to screen transactions for fraudulent activity while many customers are shopping from their lounge rooms.
"If you open a coffee shop on your street corner and a customer walks in and throws down a credit card to buy coffee, you aren't responsible for vetting that customer for fraud; but if that same person goes into your online web store to buy some beans you're going to ship to them, you are unfortunately on the hook if the transaction is fraudulent," he explained.
Manapat believes Stripe is in a unique position to help thwart fraudulent activity, as it has insight into the end customer's card details, IP address, name, email address, and so forth, in comparison to what card issuing banks or card networks like Visa or Mastercard have available to them.
In response, Stripe launched Radar, a set of machine learning-powered tools to help businesses block fraud.
Stripe Radar learns from the hundreds of thousands of businesses processing transactions on the Stripe network every second and automatically identifies fraudulent activities based on patterns learned from the payment platform's users.
In marking a transaction as risky, the machine learning platform looks up thousands of combinations of a particular card's use and other purchases from the same IP address, as some examples. It also allows Stripe's customers to submit their own suspected fraudulent activity for review.
"The mass of information to sift through is generally too much for a human," Manapat explained.
"It's not just about the information that's available for making decisions, it's that humans tend to make decisions with a bunch of prior bias. We've seen this a few times where we'll have a user who, when encountering the very first instance of fraud from say an international customer, will immediately declare that everything non-domestic is fraudulent.
"Humans will tend to generalise a little bit too broadly and that can be bad for your business. If you just decide to block every transaction that's not domestic, you probably are blocking some fraud, but more likely you're blocking an even larger fraction of good or legitimate transactions."
As the Stripe platform has developed, Manapat said his company has realised that many of the problems its customers have highlighted can be solved with machine learning.
Although tight-lipped on the specifics, Manapat said Stripe is currently working on extending its machine learning scope, which could include a platform that identifies trends in a shopper's purchases and makes recommendations on like-products, similar to that used by Amazon.com.
Another example could see Stripe head down the business intelligence or analytics path, which in theory would allow a new business to project what its revenue, for example, might look like over the next six months. Such a platform also has the potential to be accompanied with industry-wide competitive information.
Australian internet businesses have suffered more card fraud than the global average, according to a recent report [PDF] published by the Australian Payments Clearing Association (APCA).
APCA found that card not present fraud -- fraud that occurs when card details are stolen, but not the physical card -- increased 38 percent from 2014 to AU$136.7 million in 2015.