What's next for AI: Gary Marcus talks about the journey toward robust artificial intelligence
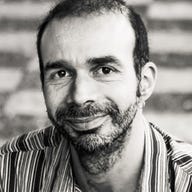
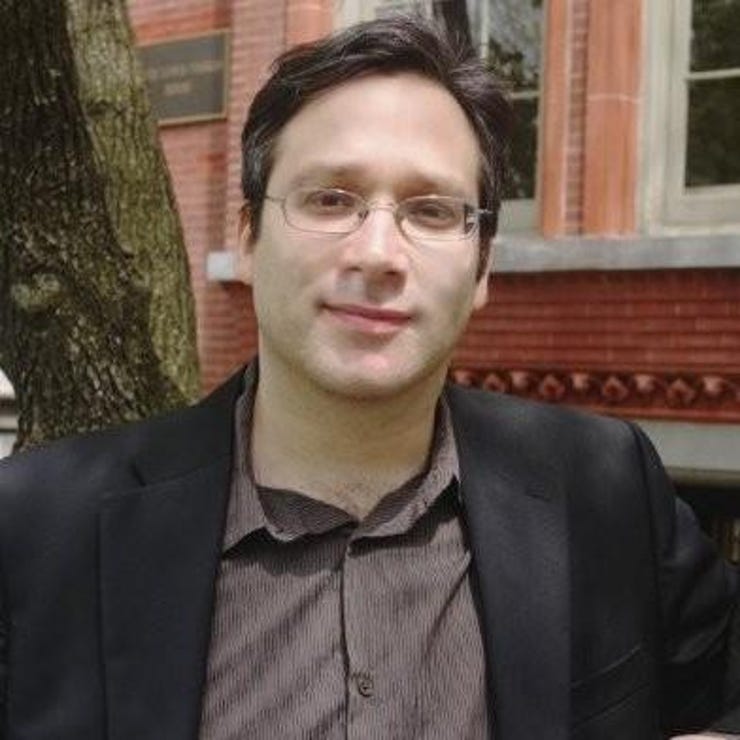
Gary Marcus is a prominent figure in AI. He dedicated his career to understanding intelligence, and this is what his approach to AI is based on.
He was not particularly talented at writing, yet became a best-selling author. He is an academic who founded two startups -- one acquired by Uber, another just scored $15 million to make building smarter robots easier. He has a humanities background, yet became one of the more prominent, and controversial, figures in AI.
If you know Gary Marcus, then you probably know it's very hard to summarize someone like him. If you don't, here's your chance to change that. Gary Marcus is a scientist, best-selling author, and entrepreneur. Marcus is well known in AI circles, mostly for his critique on -- and ensuing debates around -- a number of topics, including the nature of intelligence, what's wrong with deep learning, and whether four lines of code are acceptable as a way to infuse knowledge when seeding algorithms.
Although Marcus is sometimes seen as "almost a professional critic of organizations like DeepMind and OpenAI", he is much more than that.
As a precursor to Marcus's upcoming keynote on the future of AI in Knowledge Connexions, ZDNet caught up with Marcus on a wide array of topics. We publish the first part of the discussion today -- check back for the second part next week.
From cognitive psychology to AI
In February 2020, Marcus published a 60-page long paper titled "The Next Decade in AI: Four Steps Towards Robust Artificial Intelligence". In a way, this is Marcus' answer to his own critics, going beyond critique and putting forward concrete proposals.
Unfortunately, to quote Marcus, the world has much larger problems to deal with right now, so the paper has not been discussed as it might have been in a pre-COVID world. We agree, but we think it's time to change that. We discussed everything from his background to the AI debates and from his recent paper to knowledge graphs.
Marcus is a cognitive psychologist by training. That may seem strange for some people: how can someone who has a background in humanities be considered one of the top minds in AI? To us, it did not seem that strange. And it made even more sense after Marcus expanded on the topic.
Marcus comes to AI from a perspective of trying to understand the human mind. As a child and teenager, Marcus programmed computers - but quickly became dissatisfied with the state of that art in the 1980s.
Marcus realized that humans were a whole lot smarter than any of the software that he could write. He skipped to the last couple of years of high school, based on a translator that he wrote that worked from Latin into English, which he said was one his first serious AI projects. But then another realization hit home:
"I could do a semester's worth of Latin by using a bunch of tricks, but it wasn't really very deep and there wasn't anything else out there that was deep. This eventually led me to studying human language acquisition, and human cognitive development".
Marcus teamed up with Steven Pinker, who was his mentor as a PhD student. They worked on how human beings acquire even simple parts of language like the past tense of English. Marcus spent a lot of time comparing neural networks that were popular then to what human children did. Those neural networks went into obscurity, and then they reemerged in 2012.
When they reemerged, Marcus realized that they had all the same problems he had criticized in some of his early technical work. Marcus has spent a good part of the last decade trying to look at what we know about how children learn about the world, language and so forth, and what can that tell us about what we might need to do to make progress in AI.
Interdisciplinarity, debates, and doing it wrong
As an interdisciplinary cognitive scientist, Marcus has been trying to bring together what we know from many fields in order to answer some really hard questions: How does the mind work? How does it develop? How did it evolve in time?
That led him to be a writer as well, as he found that people in different fields didn't speak each other's languages. The only way to bring different fields together was to get people to understand one another. This was his motivation to become a writer. He started writing for The New Yorker, and has written five books, including The Algebraic Mind, Kluge, The Birth of the Mind, and The New York Times best seller Guitar Zero.
At some point, AI came back in fashion, and Marcus felt like "everybody was doing things all wrong". That led him to be an entrepreneur, as he wanted to take a different approach on machine learning. Marcus founded Geometric Intelligence, his first company, in 2014. Uber acquired it relatively early in the history of the company, and Marcus helped launch Uber AI Labs.
Marcus launched a new company called Robust AI in 2019. The goal is "to build a smarter generation of robots that can be trusted to act on their own". Rather than just being operated and working the assembly lines, Robust AI wants to build robots that work in a wide range of environments -- homes, retail, elder care, construction and so forth. Robust AI just raised $15 million, so apparently progress is under way.
Marcus believes his activities inform one another in a constructive way. But he also thinks that the culture of AI right now is unfortunate, referring to his debates with people in the deep learning camp, such as Yoshua Bengio, Geoff Hinton and Yan LeCun - who recently won the Turing Award:
You have this set of people that were toiling in obscurity. They are now in power. And I feel like rather than learning from what it's like to be disregarded, they're disregarding a lot of other people. And because I'm not shy and I have a certain kind of courage..not the kind of courage that our health care workers have, but I have gotten out on to a different kind of front line and said:
"This is what we need to do. It's not popular right now, but this is why the stuff that is popular isn't working. And that's led to a lot of people to be irritated with me. But I think, you know, I learned from my father to stand up for what I believe and that's what I do".
Language models don't know what they are talking about
Getting personal is not the best way to make progress in science, or technology. Marcus admitted to having spent a lot of time in these debates, and said he was subject to a lot of (verbal) abuse, especially between 2015 to 2018, which his Twitter feed attests to. The good side, he went on to add, was that this brought attention to the topic.
"Even leaders in the deep learning field are acknowledging the hype, and some of the technical limitations about generalization and extrapolation that I've been pointing out for a number of years. AI has a lot to offer, or could have a lot to offer if we did it better. And I'm pleased to see people starting to look at a broader range of ideas. Hopefully that will lead us to a better place".
The epitome of Marcus' critique on deep learning focuses on language models such as GPT-2, Meena, and now GPT-3. The gist of it is that these models are all based on a sort of "brute force" approach. Although they are hailed as AI's greatest achievement, Marcus remains critical and unimpressed. In his articles, as well as in his "Next Decade in AI" paper, Marcus puts forward a convincing critique of language models:
"These things are approximations, but what they're approximations to is language use rather than language understanding. So you can get statistics about how people have used language and you could do some amazing things if you have a big enough database. And that's what they've gone and done.
"So you can, for example, predict what's the next word likely to be in a sentence based on what words have happened in similar sentences over some very large database of gigabytes of data and often, locally, it's very good. The systems are very good at predicting category.
"So if you say I have three of these and two of these, how many do have in total? It will definitely give you a number. It will know that a number is supposed to come next because people use numbers in particular contexts. What these systems don't have at all is any real understanding about what they're talking about".
Marcus has done benchmarks to demonstrate his point, and shared his results. He uses simple questions to show the difference between understanding the general category of something that's going to happen next and the details of what really happens in the world:
"If I say, I put three trophies on a table and then I put another one on the table. How many do I have? You as a human being can easily add up three plus one. You build a mental model of how many things are there. And you can interpret that model. But these systems, if you say I put three trophies on the table and then another one, how many are there?
"They might say seven or twelve. They know it's supposed to be a number, but they don't actually understand that you're talking about a set of objects that are countable in a particular place and they don't know how to do the counting. Then more models came out with even bigger databases and i had examples like, what's the name of your favorite band? Avenged Sevenfold.
"Then they asked the same system was the name of your least favorite band? And again it says Avenged Sevenfold. Any human would realize your favorite band and your least favorite band can't be the same thing, unless you're lying or trying to be funny. These systems don't understand that".
The Next Decade in AI: Four Steps Towards Robust AI
Marcus points out this is a really deep deficiency, and one that goes back to 1965. ELIZA, the first expert system, just matched keywords and talked to people about therapy. So there's not much progress, Marcus argues, certainly not exponential progress as people like Ray Kurzweil claim, except in narrow fields like playing chess.
We still don't know how to make a general purpose system that could understand conversations, for example. The counter-argument to that is that we just need more data and bigger models (hence more compute, too). Marcus begs to differ, and points out that AI models have been growing, and consuming more and more data and compute, but the underlying issues remain.
Recently, Geoff Hinton, one of the forefathers of deep learning, claimed that deep learning is going to be able to do everything. Marcus thinks the only way to make progress is to put together building blocks that are there already, but no current AI system combines.
AI can't just be machine learning, or deep learning, argues Gary Marcus. We need a richer synthesis of approaches to make progress. Image: Gary Marcus, The Next Decade in AI: Four Steps Towards Robust Artificial Intelligence
Building block number one: A connection to the world of classical AI. Marcus is not suggesting getting rid of deep learning, but using it in conjunction with some of the tools of classical AI. Classical AI is good at representing abstract knowledge, representing sentences or abstractions. The goal is to have hybrid systems that can use perceptual information.
Number two: We need to have rich ways of specifying knowledge, and we need to have large scale knowledge. Our world is filled with lots of little pieces of knowledge. Deep learning systems mostly aren't. They're mostly just filled with correlations between particular things. So we need a lot of knowledge.
Number three: We need to be able to reason about these things. Let's say we have knowledge about physical objects and their position in the world -- a cup, for example. The cup contains pencils. Then AI systems need to be able to realize that if we cut a hole in the bottom of the cup, the pencils might fall out. Humans do this kind of reasoning all the time, but current AI systems don't.
Number four: We need cognitive models -- things inside our brain or inside of computers that tell us about the relations between the entities that we see around us in the world. Marcus points to some systems that can do this some of the time, and why the inferences they can make are far more sophisticated than what deep learning alone is doing.
Interestingly, what Marcus proposes seems very close to what the state of the art is in real life. But we can only scratch the surface in trying to summarize such a rich conversation on such a deep topic. We will revisit to expand with more topics and nuance, and anchor the conversation to specific approaches and technologies next week.