Google’s ‘Meena’ advances the exquisite banality of chatbots
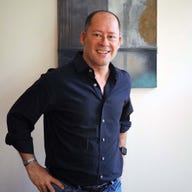
A: You're probably one of those cheerful people who dots their "i"s with little hearts.
B: I have just as much of a dark side as the next person.
A: Oh, really? When I buy a new book I always read the last page first. That way, in case I die before I finish, I know how it ends. That, my friend, is a dark side.
B: That doesn't mean you're deep or anything. I mean, yes, basically, I'm a happy person.
A: So am I.
B: And I don't think that there's anything wrong with that.
A: Of course you don't, you're too busy being happy.
Primers
The above exchange, of course, is not from any chatbot. It's from the movie When Harry Met Sally. You won't find anything this interesting in a chatbot, despite significant advances in the state of the art.
Google's AI scientists unveiled this week a breakthrough in chatbots. Dubbed "Meena," the program has few of the absurd, nonsensical statements that have tended to characterize chatbots to date. It stays pretty much on topic and is responsive to details in conversation, as rated by human reviewers who scored Meena's output.
It's also a thrilling new level of engineering. Meena's authors, led by Daniel Adiwardana, used a version of Google's "Evolved Transformer" program, which was introduced last year with a whopping 2.6 billion parameters, to digest 341 gigabytes of web text in a training session that ran for 30 days on a Google tensor processing unit "pod" (version 3), that consists of 1,024 TPU cores. This is one of the biggest language models ever run.
Meena also, unfortunately, produces some of the banalest exchanges ever between two interlocutors.
The sample text snippets, included in the published paper by Adiwardana and colleagues, are mostly about as interesting as a speed dating encounter, random exchanges of information between parties that don't know each other and who have a fairly shallow basis for conversation.
It's not surprising, given that the training data for Meena, 341 gigs, is scraped from internet social networking conversations. Social media can tend to be a lot like speed dating: Cold, impersonal, competitive, and adrift.
Here's a sample of Meena interacting with a human, where the human, in this case, a volunteer inside Google, was instructed to start every conversation with "Hi":
Human: Hi!
Meena: Hey there! What's up?
Human: Same old, Monday blues...what are you up to?
Meena: I'm procrastinating on a paper I'm supposed to be writing.
Human: are you thinking of pulling an all nighter?
Meena: Possibly. What's your paper on?
Human: Nah, i just do the grunge work around here...one day I wish to write a paper that will change the world!
Meena: What's the topic?
Human: not sure, any suggestions?
Meena: Well what's the assignment on?
Human: breathing under water
Meena: How to breathe underwater.
Human: that's what i'm hoping to figure out!
Meena: I hope you figure it out! Because I can't.
Human: i'm sure you can if you try :) you're the best!
Meena: Thank you, but I'm pretty sure you're the best.
Human: how was your weekend?
Meena: Pretty good. Yours?
Human: good, I practiced the violin
Meena: That's awesome! How long have you been playing?
Human: just started learning last month
Meena: That's awesome! What made you want to learn the violin?
Humans were employed as crowd workers to rate each such conversation for its "sensibility" and its "specificity," and such examples do, indeed, make big progress from prior chatbots. Each turn of Meena's is relevant to the human's last utterance. And Meena picks up on specifics of the human phrase, such as breathing underwater.
Sadly, the humans were not asked by Adiwardana and colleagues to rate conversations for "interestingness," because this and other exchanges in the sample are incredibly dull, like the worst text message exchange you've ever peeked at.
The example of the dialogue from When Harry Met Sally, by a master writer Nora Ephron, is not natural human speech. But it shows qualities of human interaction that highlight what is missing in all of Meena's interactions.
For one thing, the responses don't simply continue the last utterance, they can take the discussion in a surprising next direction. That's something that happens in an encounter where two humans have a subtext, such as to impress or challenge one another, as is the case between actors Billy Crystal and Meg Ryan in the scene. There's nothing especially surprising or interesting in the above exchange between Meena and the human. Meena's expressions tend to cooperate with the theme, which may be desirable in a sense but also leads to interactions that are trivial and dull.
Conversations with Meena in all the examples tend to wander in a purposeless fashion, rather than to build like in the scripted exchange, or in most human exchanges where something is happening.
In the Meena example above, while the individual turns of dialogue are plausible, there is no "throughline," so to speak, there is nothing that shapes the conversation. It's plausible overall, but it's not going anywhere; it's a random interlocking of associated phrases within the structure of turn-taking.
Meena's finest moments are when its word association turns into humorous wordplay, such as in this exchange with a human.
And those shortcomings in Meena point to the profound challenge at the heart of Meena, even as good as it is.
The objective function, in this case, was what's known as "perplexity." Perplexity represents how many words the Meena program finds probable as the next word in any sentence. Meena's best performance achieves a state of the art perplexity of 10.2, meaning it has narrowed all the tens of thousands of words in the English language that could be in any one position in a sentence to just 10 likely words.
That's an astounding accomplishment for which Adiwardana and colleagues can be very proud. Unlike prior efforts at chatbots, Meena isn't being given help to narrow the perplexity through cues about domain of speech. Nor is it subject to constraints that would shape its perplexity computation thematically. It is simply ingesting a giant gob of social media and reflecting it, but with very specific choices at each word.
Google's Meena chatbot scores low on "perplexity," which is good, meaning it has less of a hard time finding the right word. The authors find low perplexity, the X axis, corresponds with a human measure of dialogue, "sensibleness and specificity average," or "SSA," on the Y axis.
In a sense, that's quite amazing, but that's an objective that is also a problem. Meena is recreating a distribution of language that is startlingly accurate, but also merely a recreation of information, which is boring. Patterns of language in the Meena form are highly associative at a word level, which makes Meena's best examples those of wordplay. Wordplay is interesting up to a point, and it feels like it reflects intelligence, in some fashion. But it also quickly becomes superficial and tiresome.
Humans don't tend to speak only as an exchange of information or as wordplay. They have agendas, they have goals, they use rhetorical devices. The most interesting parts of human exchange are not information exchange, in general.
It's possible some future objectives for Meena could create more interesting exchanges. One objective might be to optimize for what may be an utterance-level perplexity. Meena optimizes how to drop in the right word, but its phrases overall tend to be way too obvious, leading to a kind of bland, general quality about the way it engages. Probably, human utterances represent greater perplexity at the level of the phrase, to give dialogue greater surprise and variety.
Perhaps more important, Meena needs some over-arching objective that is not merely correctness. It's possible that a domain constraint needs to be re-introduced to shape Meena's interactions. For example, one can imagine the following exchange with a chatbot that is not only sensible and specific but driving toward a goal:
Customer: Hi. I just dumped coffee on my laptop. It's not turning on.
Bot: Is it plugged in, or is this off of battery?
Customer: I've tried both. Right now, it's just on battery. The screen won't even turn on.
Bot: How long ago was the incident?
Customer: About a half an hour ago.
Bot: Did you try the method of unfolding the laptop and laying it, upside down, with the keyboard down on a flat service, like the edge of a table, on top of a towel, with the screen hanging off the edge?
Customer: No, I didn't even know that's a thing to do.
Bot: Do that right away, it can help to empty liquid still trapped in the keyboard, if there is any.
Such an exchange may still be beyond what Meena can handle despite its highly efficient perplexity. But being tested on that kind of practical task may be a promising next direction for the program. Meena needs to be employed, it needs to be given more interesting objectives, in other words, if it is to progress beyond abstract information exchanges.
As it stands today, Meena can "chat about ... anything," as Google puts it. But not in a particularly interesting way.