The role of data governance in the enterprise
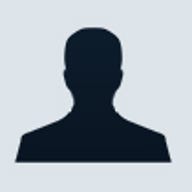

Yet, many companies struggle to properly manage their data. According to a research report by Sirius Decisions, the volume of enterprise data doubles every 18 months. Complicating matters are issues with inaccurate, duplicate or out-of-date data. Without stringent quality measures, organizations could be basing critical business decisions on flawed or inaccurate data. While most enterprise architects leave the quality of data up to database administrators–with the assumption that if you own the data, you’re responsible for its quality–this is not always the most sensible approach. Businesses should adhere to three fundamental best practices to ensure that their data is complete and up-to-date.
What best practices can businesses implement to ensure the quality of their data?
First, profile your data to determine a collection of aggregate statistics about the quality of the underlying source data. Think of your corporate data as the foundation of your house. No one would think of living in a house with a rotten foundation. Rather, prudent homeowners would make sure their foundation was inspected by a qualified inspector. An in-depth profile of your data will provide counts on what percentage of the fields are populated, in addition to providing a true understanding of the quality of the data by examining the data values.
A good profile will ask questions such as – How many unique keys are not unique? Are there symbols or commands where characters should be? Are the numbers in an appropriate format? Are fields, such as Social Security number, populated with all ones or all X’s? By comparing the universe of values within a database, you can identify outliers, anomalies and other questionable data.
Second, clean your data. Once you understand the make-up of your designated data, you must improve the quality. There are four steps to follow:
- Format Fields: Consistent style and format is key. Often with multiple data entry processes in place, the consistency suffers. Data normalization helps ensure that consistent terms and formats are used across a given field.
- Parse Components: Data parsing makes it possible for you to turn data into the usable components needed to perform automated data quality operations.
- Check Content: Some records may have accurate information, but it is located in the wrong field and some fields may have missing or inaccurate information (such as all ones). These anomalies can be either automatically or manually corrected, depending on your processes.
- Remove Duplicates: Once you have standardized and cleaned data, you can identify matches and duplicate records with a high degree of confidence.
-
Third, establish an on-going data maintenance process to validate and correct data as it comes in. Or at least put into place a program that includes both batch and real-time efforts. A research study published earlier this year, reported that data quality practices boosted revenue in B-to-B organizations by 66 percent. Sirius Decision estimated that data doubles every 12 to 18 months and if you are an average company, (i.e., one that doesn’t follow best practices for data quality), your data error rate soars to 25 percent or more.
Bad data is like a virus. Contamination rates can quickly cause serious problems and cost considerable money to fix. The consequences of poor data quality can be pervasive and far reaching such as incorrect billing for products and services, inefficient asset and inventory management and even possible regulatory issues. Errors are inevitable, so it is best to catch errors upfront and early. A best practice is to perform data maintenance at least quarterly.
What role should data quality and governance play in the enterprise?
Data governance is a key component of any enterprise information management endeavor. Data quality and data governance fit like a hand in a glove. Data governance is the collection of a corporation’s policies and practices that are essential to keeping the data secure and healthy. Your data is like a working hand; it is most productive when protected. Data governance practices determine the business rules to which your data quality processes should align. Developing data governance guidelines should be a collaborative effort between business and IT. Guidelines should be written out and must be easy to understand. Data quality guidelines should also be reviewed regularly to ensure that they still meet the needs of the enterprise. A recent benchmark study conducted by Ventana Research on data governance indicated that customer data was the most important category of data to govern, followed by financial data and data for business intelligence (BI). These areas represent a substantial part of any enterprise’s data, and you can quickly see that the roles of data quality and data governance are critical for success.
Who should be responsible for an organization’s data quality? Frankly, it is everyone’s job to ensure data quality. And it should begin at the top of the organization, with the executives and then include stakeholders from every level. For most companies, the operational responsibility lies within the data governance committee. Best practices demonstrate that most data governance committees represent exactly this mix. On a day-to-day basis, many organizations are embracing the role of the data steward. This role began in the IT arena, but trends indicate that the role is branching out as the level of accountability entrusted to them increases. It is largely the data steward (also a member of the data governance team) who will determine the business rules for the organization’s data quality.
Ensuring your organization’s data quality is satisfactory is an ongoing process. After assessing your data quality and taking steps to fix incomplete, inaccurate or duplicate data, organizations need to create data governance programs. Without a plan and routine maintenance, companies may find themselves having to deal with bad data on a continual basis, which can derail the success of any business initiative. By ensuring that your organization has a solid foundation, you can be confident that all your efforts – whether it’s targeted marketing, managing inventory or even bill production– are based on accurate and complete data.
biography
As Director of Product Management for Global Data Quality (GDQ) at Pitney Bowes Business Insight, Navin manages a full portfolio of products. He has more than 10 years of experience in the area of data management helping companies with the modeling, analysis, design and implementation of their data management strategies.