Weird, but inevitable: algorithm now serves on a corporate board

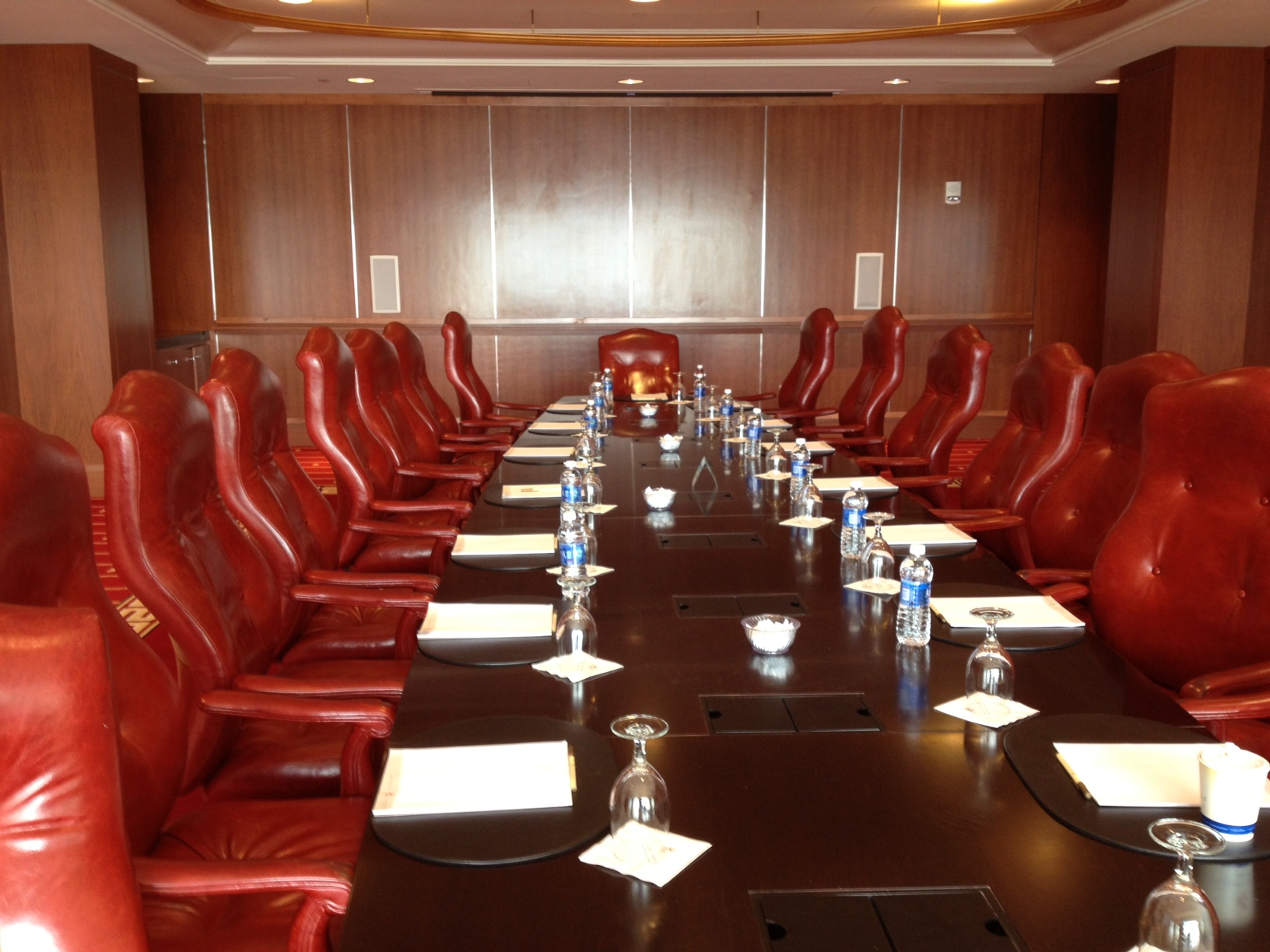
The rise of algorithms in corporate spaces is part of Dr. John Bates' latest book: Thingalytics: Smart Big Data Analytics for the Internet of Things. We shouldn't be surprised about "algos" achieving such stature these days, he observes. "Algorithms feed on sensory inputs and give birth to analysis, recommendations, decisions and actions. When they are fed with the rich inputs from streaming data and the Internet of Things, they drive a smarter world. From Amazon deciding which books you might like to Match.com choosing your ideal life partner, algorithms have become ubiquitous."
Bates adds that the idea of an algorithm being promoted to the board may seem peculiar, but "it can't be any worse than some boards I have served on."
But what about the day-to-day stuff needed to support and sustain these algorithms? Are enterprises ready to handle all the requirements for maintaining streaming data and related applications that can deliver IoT capabilities, even for mundane things such as suggesting changes in logistics or production schedules? A recent study out of State Street suggests that organizations are only just beginning to get a grip on their rapidly bursting data environments. The survey of 400 investment firms (where VITAL works, by the way) finds that while 81 percent view data and analytics as one of their top strategic priorities, only 37 percent have advanced data infrastructure, expertise and high quality governance in place. This likely mirrors the state of big data management across many industries as well.
Still, Bates says the opportunity offered by IoT is huge, and there are ways to deftly address concerns about infrastructure, or lack thereof. At this week's CeBIT event in Hannover, Germany, I had the opportunity to sit down with Bates, who is CTO of Software AG, to chat about his book and experience with IoT. IoT and analytics isn't just for large corporations with huge budgets, he says, adding that "I think everybody is ready for this." With resources such as cloud now widely available, hardware and underlying infrastructure should no longer be a concern to enterprises seeking to dive deep into IoT analytics and process huge data sets. "It's a mindset," he adds. Whether large or small, companies can act on the opportunities. Uber was a startup that embraced IoT, with virtually no infrastructure in the beginning. "Uber is the absolute definition of thingalytics," he notes. "It's the connection of people with cabs when you need them."
Here are the things one needs to think about when looking at thinganalytics, outlined by Bates in his book:
Big data streaming analytics. Bates channels Forrester analyst Mike Gualteri: "One key innovation that software architectures have to manage is data streaming.You are not asking the sensor for the data; the sensor is always reporting. A traditional app is more the request/response model.When the streaming data comes in, technology has to digest the information it contains, determining what it means and whether it fits into a pattern that has business significance."
Streaming analytics engine. The streaming analytics engine is responsible for running continuous query rules that drive the logic behind thingalytics," Bates writes. "The engine continuously monitors everything that is happening to identify situations that a user has defined as important. The key difference between a streaming analytic engine and a database is that the former is event driven and is always on. Unlike a database, a streaming analytics engine can be programmed ti take autonomous actions, immediately driving a decision when detecting a problem."
Fast big data acquisition and integration. "High-performance messaging and integration should always underpin a big streaming data analytics platform," says Bates. "This integration should support plugging into a wide range of systems, collecting real-time updates and communicating the results. Existing enterprises are awash with data, much of it already connected to messaging buses that ship data between applications." In addition, Bates recommends a "highly scalable messaging architecture to stream real-time events; to support communications among multiple streaming engines; in-memory caches and visual dashboards; and to communicate events out to applications and processed. This architecture requires adapters to connect to common APIs -- such as machine-to-machine connectivity protocol (MQTT)."
Cloud. Back-end capabilities "run on a server somewhere, utilizing one or more CPU cores," Bates explains. "As the data loads of such an application ebb and flow, underlying hardware requirements change in tandem. The optimal strategy to navigate these peaks and troughs is for the platform to run in a cloud with the ability to provision computing resources automatically in response to the changing load. In the coming years, more and more streaming analytics platforms, along with the data they analyze, will be located in the cloud." In one case, a company created a "machine cloud" to manage sensor events.
(Disclosure: Software AG assisted with the author's travel expenses to CeBIT.)