What AI forgets could kill us, but new research is helping it remember
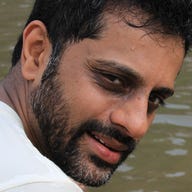
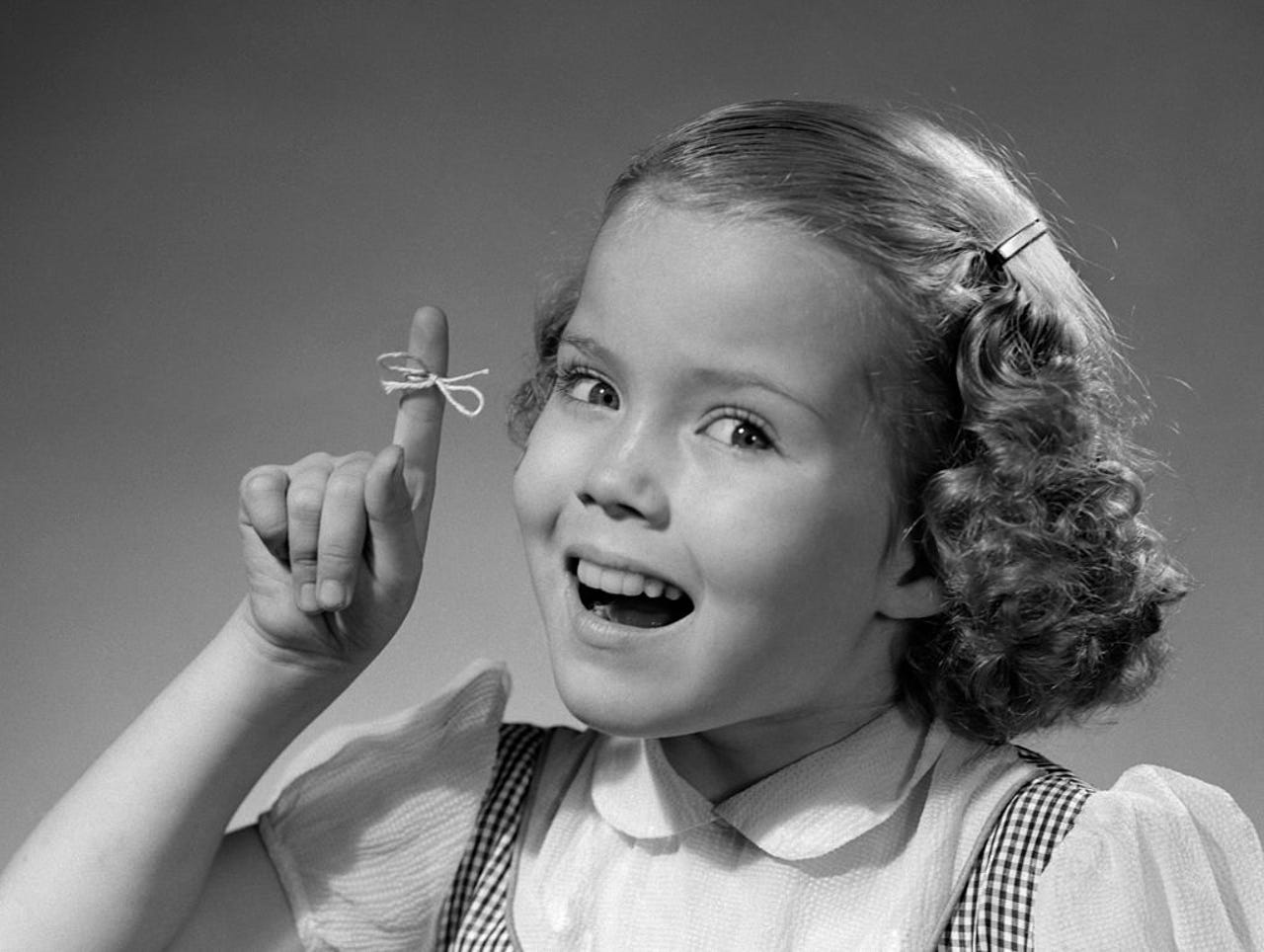
According to most reports, AI will soon be everywhere, pretty much like sugar, or Taylor Swift.
AI systems will soon form an intelligent backbone to everything we use or do and transform industries and society as a whole, say experts.
Also: AI safety and bias: Untangling the complex chain of AI training
So, imagine if you're flying somewhere up there in the friendly skies, and your aircraft's central nervous system which is now AI-managed suddenly shuts down, leaving the plane powerless.
Or, the New York Stock Exchange decides to take an unexpected and immediate holiday by shutting down and sending the economy – and your life savings – into a death spiral.
Or your toaster refuses to deliver that one piece of food that your body will accept before you embark on your daily trudge to work.
Believe it or not, the killer technology that was going to send humanity hurtling to its demise – a conviction belonging to no less an AI authority than the very man who invented it, Geoffrey Hinton – has a little problem to attend to before it lives up to its murderous potential.
As problems go, it's a terrible one to have – it simply cannot remember older things.
And when it doesn't remember, it proceeds to shut down instantly in an act called "catastrophic forgetting" that may have eerie parallels to your entire high school educational experience.
Also: Can generative AI solve computer science's greatest unsolved problem?
In remembrance of things past
Any basic AI system worth its chips is one that should be able to successfully learn a sequence of tasks in a process called continual learning.
In humans, learning happens when our brain is able to summon up memories of past instances of doing something.
This hinges on the REM cycle part of our sleep phase where recent memories are shunted to the long-term bin so new ones can be made.
Also: ChatGPT vs. Bing Chat vs. Google Bard: Which is the best AI chatbot?
AI neural networks essentially mimic how the human brain works so there has long been an expectation that an algorithm can use its stored knowledge of executing all its old jobs to learn new ones very much like we humans do -- but this just doesn't seem to be working as expected.
Something is going on -- or not going on, as the case may be -- in the training of artificial neural networks that is causing huge gaps in cognition. The neural networks will forget all their old information while learning new things -- and then they will proceed to freeze.
To fix this, researchers embarked upon a novel strategy -- they began feeding AI systems old data while processing new ones, a process called interleaved training, which they thought was how the brain works when asleep.
It turns out that this process doesn't actually happen in the brain; from just a practical point of view, there isn't anywhere close to the time needed for the brain -- or its machine imitator -- to digest all this old learning data while asleep.
The answer had to lie elsewhere.
Also: Generative AI will far surpass what ChatGPT can do. Here's everything on how the tech advances
Researchers from the Institute of Computer Science of the Czech Academy of Sciences in Prague, Czech Republic, and the University of California, San Diego, also looked at sleep, but through another lens.
They eschewed a conventional neural network -- one that constantly adjusts its synapses (the links between neurons) until it is able to find a solution -- for a 'spiking' one that they thought most closely resembles the human brain.
A 'spiking' network sends an output only after receiving a whole bunch of signals over time and therefore shifts around much less data and uses much less power and bandwidth, according to the researchers. In doing so, it is able to re-activate neurons involved in learning old tasks. It seemed to work.
The spiking neural network was capable of performing both tasks after undergoing sleeplike phases.
"Our work highlights the utility in developing biologically inspired solutions," says one of the study's researchers, Jean Erik Delanois, from the University of California, San Diego.
In the image of thy creator
Meanwhile, more recently, researchers from Ohio State University steered clear of sleep while tackling the same problem of catastrophic forgetting in deep-learning neural nets.
They used an entirely different and ingenious approach to solve this problem.
"Our research delves into the complexities of continuous learning in these artificial neural networks, and what we found are insights that begin to bridge the gap between how a machine learns and how a human learns," said Ness Shroff, a professor of computer science and engineering at Ohio State.
Also: 6 AI tools to supercharge your work and everyday life
Shroff and his colleagues discovered that traditional machine learning algorithms are force-fed data in one big push, but that's not necessarily good for the machine. In fact, how close tasks resemble each other, what they have in common, and even what order the tasks are taught in all affect how well the algorithm remembers them.
In what may just be one of the more curious ironies of our times, Shroff and his colleagues found that algorithms, much like humans, were able to remember much better when fed with very different tasks in succession instead of a series of similar tasks.
Human brains also function like this. The same events -- parties, vacations, even days of the week -- blur into each other if the same location or experience for them is repeated. But the different ones stand out.
The Ohio State researchers discovered that dissimilar tasks should be introduced very early in the continual learning process for the AI to learn new things as well as tasks similar to old ones.
Also: ChatGPT is more like an 'alien intelligence' than a human brain, says futurist
"Their work is particularly important as understanding the similarities between machines and the human brain could pave the way for a deeper understanding of AI, said Shroff.
For AI to be truly effective and safe, algorithms need to be able to learn better, handle different and unexpected situations, and be scalable.
These two solutions for impaired machine memories should help considerably toward that goal.