AI Debate 2: Night of a thousand AI scholars
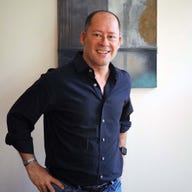
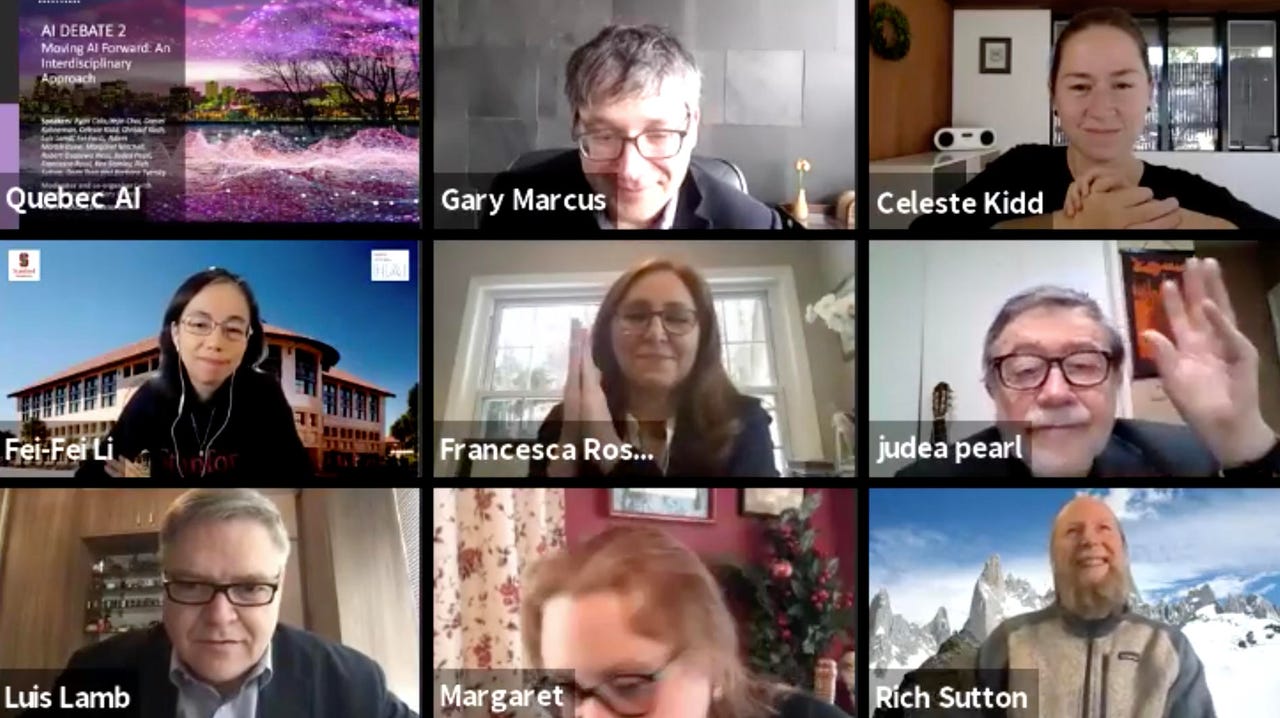
Gary Marcus, top, hosted presentations by sixteen AI scholars on what things are needed for AI to "move forward."
A year ago, Gary Marcus, a frequent critic of deep learning forms of AI, and Yoshua Bengio, a leading proponent of deep learning, faced off in a two-hour debate about AI at Bengio's MILA institute headquarters in Montreal.
Wednesday evening, Marcus was back, albeit virtually, to open the second installment of what is now planned to be an annual debate on AI, under the title "AI Debate 2: Moving AI Forward." (You can follow the proceedings from 4 pm to 7 pm on Montreal.ai's Facebook page.)
Vincent Boucher, president of the organization Montreal.AI, who had helped to organize last year's debate, opened the proceedings, before passing the mic to Marcus as moderator.
Marcus said 3,500 people had pre-registered for the evening, and at the start, 348 people were live on Facebook. Last year's debate had 30,000 by the end of the night, noted Marcus.
Bengio was not in attendance, but the evening featured presentations from sixteen scholars: Ryan Calo, Yejin Choi, Daniel Kahneman, Celeste Kidd, Christof Koch, Luís Lamb, Fei-Fei Li, Adam Marblestone, Margaret Mitchell, Robert Osazuwa Ness, Judea Pearl, Francesco Rossi, Ken Stanley, Rich Sutton, Doris Tsao and Barbara Tversky.
"The point is to represent a diversity of views," said Marcus, promising three hours that might be like "drinking from a firehose."
Also: Devil's in the details in Historic AI debate
Marcus opened the talk by recalling last year's debate with Bengio, which he said had been about the question, Are big data and deep learning alone enough to get to general intelligence?
This year, said Marcus, there had been a "stunning convergence," as Bengio and another deep learning scholar, Facebook's head of AI, Yann LeCun, seemed to be making the same points as Marcus about how there was a need to add other kinds of elements to deep learning to capture things such as causality and reasoning. Marcus cited with approval LeCun's tweets this year about how giant neural network models such as GPT-3 fell down on some tasks.
There was also a paper co-written by machine learning pioneer Jürgen Schmidhuber that was posted this month that seemed to be part of that convergence, said Marcus.
Following this convergence, said Marcus, it was time to move to "the debate of the next decade: How can we move AI to the next level."
Each of the sixteen speakers spoke for roughly five minutes about their focus and what they believed AI needs. Marcus compiled a nice reading packet you can check from each of the scholars as background.
INTERACTION, NEUROSYMBOLISM, COMPUTATIONAL THEORY
Fei-Fei Li, the Sequoia Professor of computer science at Stanford University, started off the proceedings. Li talked about what she called the "north star" of AI, the thing that should be serving to guide the discipline. In the past five decades, one of the north stars, said Li, was the scientific realization that object recognition is a critical function for human cognitive ability. Object recognition led to AI benchmark breakthroughs such as the ImageNet competition (which Li helped create.)
The new north star, she said, was interaction with the environment. Li cited a 1963 study by Richard Held and Alan Hein, "Movement-Produced Stimulation in the Development of Visually Guided Behavior." The researchers conducted experiments on pairs of neonatal kittens, where one kitten was allowed to move about to explore its environment, while the other kitten was moved about in a carrier, so that it had passive, not active exploration. Held and Hein concluded that being passive versus active in early stages of exploration leads to diminished spatial perception and coordination in kittens. Hence, Held and Hein concluded that self-directed behavior in an environment is necessary to develop an ability to navigate the world.
Following LI, Luís Lamb, who is professor of computer science at the Universidade Federal do Rio Grande do Sul in Brazil, talked about "neurosymbolic AI," a topic he had presented a week prior at the NeurIPS AI conference.
Calling Marcus and Judea Pearl "heroes," Lamb said the neurosymbolic work built upon Marcus's concepts in Marcus's book, "The Algebraic Mind," including the need to manipulate symbols, on top of neural networks. "We need a foundational approach based both on logical formalization" such as Pearl's "and machine learning," said Lamb.
Lamb was followed by Rich Sutton, distinguished scientist at DeepMind. Sutton recalled neuroscientist David Marr, who he called one of the great figures of AI. He described Marr's notion of three levels of processing that were required: computational theory, representation and algorithm, and hardware implementation. Marr was particularly interested in computational theory, but "there is very little computational theory" today in AI, said Sutton.
Things such as gradient descent are "hows," said Sutton, not the "what" that computational theory needed.
"Reinforcement learning is the first computational theory of intelligence," declared Sutton. Other possibilities were predictive coding, bayesian inference, and such.
"AI needs an agreed-upon computational theory of intelligence," concluded Sutton, "RL is the stand-out candidate for that."
'DEEP UNDERSTANDING,' PROBABILISTIC PROGRAMS, EVOLUTION
Next came Pearl, who has written numerous books about causal reasoning, including the Times best-seller, The Book of Why. His talk was titled, "The domestication of causality."
"We are sitting on a goldmine," said Pearl, referring to deep learning. "I am proposing the engine that was constructed in the causal revolution to represent a computational model of a mental state deserving of the title 'deep understanding'," said Pearl.
Deep understanding, he said, would be the only system capable of answering the questions, "What is?" "What if?" and "If Only?"
Next up was Robert Ness, on the topic of "causal reasoning with (deep) probabilistic programming."
Ness said he thinks of himself as an engineer, and is interested in building things. "Probabilistic programming will be key," he said, to solving causal reasoning. Probabilistic programming could build agents that can reason counter-factually, a key to causal reasoning, said Ness. That was something he felt was "near and dear to my heart." This, he said, could address Pearl's question pertaining to the "If only?"
Also: What's in a name? The 'deep learning' debate
Next was Ken Stanley, who is Charles Millican professor of computer science at the University of Central Florida. Stanley took a sweeping view of evolution and creativity.
While computers can solve problems, said Stanley, humans do something else that computers don't do: open-ended innovation.
"We layer idea upon idea, over millennia, from fire and wheels up to space stations, which we would call an open-ended system." Stanley said evolution was a parallel, a "phenomenal system," he said, that had produced intelligence. "Once we began to exist, you got all the millennia of creativity," said Stanley. "We should be trying to understand these phenomena," he said.
Following Stanley was Yejin Choi, the Brett Helsel Associate Professor of Computer Science at the University of Washington.
She recalled Roger Shepard's "Monsters in a Tunnel," a famous visual illusion. Shepard drew two creatures in a tunnel with pronounced perspective. Although the figures on the picture plane of the two entities are the exact same size, at a glance, one figure looks smaller than the other, the effect of perspective.
Shepard's illustration, noted Choi, was discussed in the 2017 book The Enigma of Reason, by Hugo Mercier and Dan Sperber, as an example of how the brain uses context to understand an image.
That lead Choi to talk about the importance of language, and also "reasoning as generative tasks."
"We just reason on the fly, and this is going to be one of the key fundamental challenges moving forward."
Choi said new language models like "GPT-4, 5, 6 will not cut it."
MODULARITY, UNKNOWN VARIABLES
Following Choi's presentation, Marcus began the first of several Q&A sessions that interspersed the presentations.
Marcus took a moment to identify what he deemed convergence among the speakers: on counter-factuals; on dealing with the unfamiliar and open-mindedness; on the need to integrate knowledge, to Pearl's point about data alone not being enough; the importance of common sense (to Choi's point about the "generative.")
Marcus asked the panel, "Okay, we've seen six or seven different perspectives, do we want to put all those together? Do we want to make reinforcement learning compatible with knowledge?"
Lamb noted that neurosymbolic systems are not about "the how."
"It's important to understand we have the same aims of building something very solid in terms of learning, but representation precedes learning," said Lamb.
Marcus asked the scholars to reflect on modularity. Sutton replied that he would welcome lots of ways to think about the overall problem, not just representation. "What is it? What is the overall thing we're trying to achieve?" with computational theory, he prompted. "We need multiple computational theories," said Sutton.
Among audience questions, one individual asked how to reason in a causal framework with unknown or novel variables. Ness talked about COVID-19 and studying viruses. Prior viruses helped to develop causal models that were able to be extended to SARS-CoV-2. He referred to dynamic models such as the ubiquitous "SEI" epidemiological model, and talked about modeling that in probabilistic languages. "You encounter it a lot where you hit a problem, you'r unfamiliar, but you can import abstractions from other domains," said Ness.
Choi pointed out "Humans are capable of believing strange things, and do strange causal reasoning."
Choi asked , "do we want to build a human-like system?" Choi said one thing that is interesting about humans is the ability to communicate so much knowledge in natural language, and to learn through natural language.
Marcus asked Li and Stanley about "neuro-evolution." "What's the status of that field?" he asked.
Stanley said the field might use evolutionary algorithms, but also might just be an attempt to understand open-ended phenomena. He suggested "hybridizing" evolutionary approaches with learning algorithms.
"Evolution is one of the greatest, richest experiments we have seen in intelligence," said Li. She suggested there will be "a set of unifying principles behind intelligence." "But I'm not dogmatic about following the biological constraints of evolution, I want to distill the principles," said Li.
GESTURES, INNATE-NESS, NEUROSCIENCE
After the question break, Marcus brought on Tversky, emeritus professor of psychology at Stanford. Her focus was encapsulated on the slide titled, "All living things must act in space … when motion ceases, life ends." Tversky talked about how people make gestures, making spatial motor movements that can affect how well or how poorly people think.
"Learning, thinking, communication, cooperation, competing, all rely on actions, and a few words."
Daniel Kahneman was next, Nobel laureate and author of Thinking, Fast and Slow, a kind of bible for some in issues of reasoning in AI. Kahneman noted he has been identified with the book's paradigm, "System 1 and System 2 thinking." One is a form of intuition, the other a form of higher-level reasoning.
Kahneman used his time to clear up some misconceptions in the use of the terms.
Kahneman said System 1 could encompass many things that are non-symbolic, but it was not true to say System 1 is a non-symbolic system. "It's much too rich for that," he said. "It holds a representation of the world, the representation that allows a simulation of the world," which humans live with, said Kahneman.
"Most of the time, we are in the valley of the normal, events that we expect." The model accepts many events as "normal," said Kahneman, even if unexpected. It rejects others. Picking up Pearl, Kahneman said a lot of counter-factual reasoning was in System 1, where an innate sense of what is normal would govern such reasoning.
Following Kahneman was Doris Tsao, a professor of biology at CalTech. She focused on feedback systems, recalling early work on neurons of McCulloch and Pitts. Feedback is essential, she said, citing back-propagation in multilayer neural nets. Understanding feedback may allow one to build more robust vision systems, said Tsao. Tsao said feedback systems might help to understand phenomena such as hallucinations. Tsao said she is "very excited about the interaction between machine learning and systems neuroscience."
Next was Marblestone of MIT, previously a research scientist at DeepMind continued the theme of neuroscience. He said making observations of the brain, trying to abstract to a theory of functioning, was "right now at a very primitive level of doing this." Marblestone said examples of neural nets, such as convolutional neural networks, were copying human behavior.
Marblestone spoke about moving beyond just brain structure to human activity, using fMRIs, for example. "What if we could model not just how someone is categorizing an object, but making the system predict the full activity in the brain?"
Next came Koch, a researcher with the Allen Institute for Brain Science in Seattle. He started with the assertion, "Don't look to neuroscience for help with AI."
The Institute's large-scale experiments reveal complexity in the brain that is far beyond anything seen in deep learning, he said. "The connectome reveals that brains are not just made out of millions of cells, but a thousand different neuronal cell types that differentiate by the genes expressed, the addresses they send the information to, the differences in synaptic architecture in dendritic trees," said Koch. "We have highly heterogeneous components," he said. "Very different from our current VLSI hardware."
"This really exceeds anything that science has ever studied, with these highly heterogenous components on the order of 1,000 with connectivity on the order of 10,000." Current deep neural networks are "impoverished," said Koch, with gain-saturation activation units. "The vast majority of them are feed forward," said Koch, whereas the brain has "massive feedback."
"Understanding brains is going to take us a century or two," he advised. "It's a mistake to look for inspiration in the mechanistic substrate of the brain to speed up AI," said Koch, contrasting his views to those of Marblestone. "It's just radically different from the properties of a manufactured object."
DIVERSITY, CELLS, OBJECT FILES, EMMANUEL KANT
Following Koch, Marcus proposed some more questions. He asked about "diversity," what could individual pieces of the cortex tell us about other parts of the cortex?
Tsao responded by pointing out "what strikes you is the similarity," which might indicate really deep general principles of brain operation, she said. Predictive coding is a "normative model" that could "explain a lot of things." "Seeking this general principle is going to be very powerful."
Koch jumped on that point, saying that cell types are "quite different," with visual neurons being very different from, for example, pre-frontal cortex neurons.
Marblestone responded "we need to get better data" to "understand empirically the computational significance that Christof is talking about."
Marcus asked about innateness. He asked Kahneman about his concept of "object files." "An index card in your head for each of the things that you're tracking. Is this an innate architecture?"
Kahneman replied that object files is the idea of permanence in objects as the brain tracks them. He likened it to a police file that changes contents over time as evidence is gathered. It is "surely innate," he said.
Marcus posed that there is no equivalent of object files in deep learning.
Marcus asked Tversky if she is a Kantian, meaning, Emmanuel Kant's notion that time and space are "innate." She replied that she has "trouble with innate," even object files being "innate." Object permanence, noted Tversky, "takes a while to learn." The sharp dichotomy between innate and what is acquired made her uncomfortable, she said. She cited Li's notion of acting in the world as important.
Celeste Kidd echoed Tversky in "having a problem saying what it means to be innate." However, she pointed out "we are limited in knowing what an infant knows," and there is still progress being made to understand what infants know.
BIAS, VALUE STATEMENTS, PRINCIPLES, LAW
Next was Kidd's turn to present. Kidd is a professor at UC Berkeley whose lab studies how humans form beliefs. Kidd noted algorithmic bias is dangerous for the ways they can "drive human belief sometimes in destructive ways." She cited AI systems for content recommendation, such as on social networks. Such systems can push people toward "stronger, inaccurate beliefs that despite our best efforts are very difficult to correct." She cited the examples of Amazon and LinkedIn using AI for hiring, which had a tendency to bias against female job candidates.
"Biases in AI systems reinforce and strengthen bias in the people that use them," said Kidd. "Right now is a terrifying time in AI," said Kidd, citing the termination of Timnit Gebru from Google. "What Timnit experienced at Google is the norm, hearing about it is what's unusual."
"You should listen to Timnit and countless others about what the environment at Google was like," said Kidd. "Jeff Dean should be ashamed."
Next up was Mitchell, who was co-lead with Gebru at Google. "The typical approach to developing
machine learning collects training data, training the model, output is filtered, and people can see the output." But, said Mitchell, human bias is upfront in the collection of data, the annotation, and "throughout the rest of development. It further affects model training." Things such as loss function put in a "value statement," said Mitchell. There are biases in post-processing, too.
"People see the output, and it becomes a feedback loop," you home in on the beliefs, she said. "We were trying to break this system, which we call bias laundering." There is no such thing as neutrality in development, said Mitchell, "development is value-laden."
Among the things that need to be operationalized, she said, is to break down what is packed into development, so that people can reflect on it, and work toward "foreseeable benefits" and away from "foreseeable harms and risks." Tech, said Mitchell, is usually good at extolling the benefits of tech, but not the risks, nor pondering the long-term effects.
"You wouldn't think it would be that radical to put foresight into a development process, but I think we're seeing now just how little value people are placing on the expertise and value of their workers," said Mitchell. She said she and Gebru continue to strive to bring the element of foresight to more areas of AI.
Next up was Rossi, an IBM fellow. She discussed the task of creating "an ecosystem of trust" for AI. "Of course we want it to be accurate, but beyond that, we want a lot of properties," including "value alignment," and fairness, but also explainability. "Explainability is very important especially in the context of machines that work with human beings."
Rossi echoed Mitchell about the need for "transparency," as she called it, the ways bias is injected in the pipeline. Principles, said Rossi, are not enough, "it needs a lot of consultation, a lot of work in helping developers understand how to change the way they're doing things," and some kind of "umbrella structure" within organizations. Rossi said efforts such as neurosymbolic and Kahneman's Systems 1 and 2 would be important to model values in the machine.
The last presenter was Ryan Calo, a professor of law at University of Washington. "I have a few problems with principles," said Calo, "Principles are not self-enforcing, there are no penalties attached to violating them," he said.
"My view is principles are largely meaningless because in practice they are designed to make claims no one disputes. Does anyone think AI should be unsafe? I don't think we need principles. What we need to do is roll up our sleeves and assess how AI affects human affordances. And then adjust our system of laws to this change. Just because AI can't be regulated as such, doesn't mean we can't change law in response to it."
Marcus replied, saying the "Pandora's Box is open now," and that the world is in "the worst period of AI. We have these systems that are slavish to data without knowledge," said Marcus.
Marcus asked about benchmarks. Can machines have some "reliable way to meаsure our progress toward common sense." Choi replied that the training of machines through examples is a pitfall. Or self-supervised learning, also problematic.
"The truth about the current deep learning paradigm is, I think it's just wrong, so the benchmark will have to be set up in more of a generative way." There's something about this generative ability humans have that if AI is going to get closer to, we really have to test the generative ability."
Marcus asked about the literature on human biases. What can we do about them. "Should we regulate our social media companies? What about interventions," he asked Kahneman, who has done a lifetime of work on human biases.
Kahneman was not optimistic, he said. Efforts at changing the way people think intuitively have not been very successful. "Two colleagues and I have just finished a book where we talk about decision hygiene," he said. "That would be some descriptive principles you apply in thinking about how to think, without knowing what are the biases you are trying to eliminate. It's like washing your hands: you don't know what you are preventing."
Tversky followed, saying "there are many things about AI initiatives that mystify me, one is the reliance on words when so much understanding comes in other ways, not in words."
Mitchell noted that cognitive biases can lead to discrimination. Discrimination and biases are "closely tied" in model development, said Mitchell. Pearl offered in response that some biases might be corrigible via algorithms if they can be understood. "Some biases can be repaired if they can be modeled." He referred to this as bias "laundering."
Kidd noted work in her lab to reflect upon misconceptions. People all reveal biases that are not justified by evidence in the world. Ness noted that cognitive biases can be useful inductive biases. That, he said, means that problems in AI are not just problems of philosophy but "very interesting technical problems."
ROBOTS
Marcus asked about robots. His startup, Robust AI, is working on such systems. Most robots that exist these days "do only modest amounts of physical reasoning, just how to navigate a room," observed Marcus. He asked Li, "how are we going to solve this question of physical reasoning that is very effective for humans, and that enters into language."
Li replied that "embodiment and interaction is a fundamental part of intelligence," citing pre-verbal activity of babies. "I cannot agree more that physical reasoning is part of the larger principles of developing intelligence," said Li. It was not necessary to go through necessarily the construction of physical robots, she said. Learning agents could serve some of that need.
Marcus followed up asking about the simulation work in Li's lab. He mentioned "affordances." "When it comes to embodiment," said Marcus, "a lot of it is about physical affordances. Holding this pencil, holding this phone. How close are we to that stuff?"
"We're very close," said Li, citing, for example, chip giant Nvidia's work on physical modeling. "We have to have that in the next chapter of AI development," said Li of physical interactions.
Sutton said "I try not to think about the particular contents of knowledge," and so he's inclined to think about space as being like thinking about other things. It's about states and transitions from one state to another. "I resist this question, I don't want to think about physical space as being a special case."
Tversky offered that a lot is learned by people from watching other people. "But I'm also thinking of social interactions, imitation. In small children, it is not of the exact movements, it's often of the goals."
Marcus replied to Tversky that "Goals are incredibly important." He picked up the example of infants. "A fourteen-month-old does an imitation — either the exact action, or they will realize the person was doing something in a crazy way, and instead of copying, they will do what the person was trying to do," he said, explaining experiments with children. "Innateness is not equal to birth, but early in life, representations of space and of goals are pretty rich, and we don't have good systems for that yet" in AI, said Marcus.
Choi replied to Marcus by re-iterating the importance of language. "Do we want to limit common sense research to babies, or do we want to build a system that can capture adult common sense as well," she prompted. "Language is so convenient for us" to represent adult common sense, said Choi.
CURIOSITY
Marcus at this point changed topics, asking about "curiosity." A huge part of humans' ability, he said, "is setting ourselves an agenda that in some way advances our cognitive capabilities."
Koch chimed in, "This is not humans restricted, look at a young chimp, young dog, cat… They totally want to explore the world."
Stanley replied that "this is a big puzzle because it is clear that curiosity is fundamental to early development and learning and becoming intelligent as a human," but, he said, "It's not really clear how to formalize such behavior."
"If we want to have curious systems at all," said Stanley, "we have to grapple with this very subjective notion of what is interesting, and then we have to grapple with subjectivity, which we don't like as scientists."
Pearl proposed an incomplete theory of curiosity. It is that mammals are trying to find their way into a system where they feel comfortable and in control. What drives curiosity, he said, is having holes in such systems. "As long as you have certain holes, you feel irritated, or discomfort, and that drives curiosity. When you fill those holes, you feel in control."
Sutton noted that in reinforcement learning, curiosity has a "low-level role," to drive exploration. "In recent years, people have begun to look at a larger role for what we are referring to, which I like to refer to as 'play.' We set goals that are not necessarily useful, but may be useful later. I set a task and say, Hey, what am I able to do. What affordances." Sutton said play might be among the "big things" people do. "Play is a big thing," he said.
An audience member asked the notion of "meta-cognition." Rossi replied that there must be "some place" in Kahneman's System 1 or System 2 were there is reasoning about the entity's own capabilities, "and to say, I would like to respond in this way, or in this other way. Or devise a new procedure to respond to this stimulus from outside." Rossi suggested such ability might help with curiosity and with Sutton's notion of play.
Lamb followed that "eventually, at the end of the day, we want to get the reasoning sound, in terms of providing this System 1 and System 2, integrated. The learning component of truly sound AI systems have to see them in a convergent way. Eventually the reasoning process has to be built in a sound way."
WHERE DO YOU WANT TO GO?
Marcus took another audience question and demanded each person answer it : "Where do you want AI to go, what would make you happy? What is the objective function for those of us building AI."
Li: As a scientist, I want to push the scientific knowledge and principles of AI further and further. I still feel our AI era is pre-Newtonian physics. We are still studying phenomenology and engineering. There is going to be a set of moments when we start to understand the principles of intelligence. As a citizen, I want this to be a technology that can in an idealistic way better human conditions. It's so profound, it can be very, very bad, it can be very, very good. I would like to see a framework of this technology developed and deployed in the most benevolent way.
Lamb: I like one of the sayings of Turing, we can only see a short distance ahead. As Fei-Fei said, i want Ai, as a scientist, to advance. But since it has as big an impact as what people have been saying here, they are key responsibilities of ourselves. What we are trying to do is to consolidate traditions of AI. We've to look for convergence, as you said, Gary. Making AI fairer, less biased, and something very positive for us as humanity. We need to see our fields in a very humanistic way. We have to be guided by serious ethical principles and laws and norms. We are at the beginning of an AI Cambrian explosion. We need to be very aware of social, ethical and global implications. We have to be concerned about the north/south divide. We can not see it from only a single cultural perspective.
Sutton: You know, AI sounds like something purely technical. I agree with Luís, it is maybe the most humanistic of all endeavors. I look forward to our greater understanding, and a world populated with different kinds of intelligence, augmented people, and new people, and to understanding and novelty and varieties of intelligences.
Pearl: My aspirations are fairly modest. All I want is an intelligence, very friendly and super competent apprentice. I would like to understand myself, how I think, how I am aroused emotionally. There are some difficult scientific questions about myself that I don't yet have a solution for. Consciousness, for instance, and free will. If I can build a robot that has the kind of free will I have, I will take it as the greatest scientific achievement of the 23rd century, and I am going to make a prediction: we will have it!
Ness: So much of the discussion is about automating what humans do. It's interesting to think about how this could augment human intelligence. And enhance human experiences. I've seen posts people have written of poetry, even, with these large language models. Wouldn't it be better to build something to actually help poets create novel poetry? I like pop music, but I would also like to hear some novel endeavors. The first engineers who built Photoshop built those Matrix-y images of VR People. But no designer today doesn't know how to use Photoshop. I hope AI will do for human experience what Photoshop did for designers.
Stanley: It's difficult to articulate, this is such a great endeavor. For me it's a bit along the lines Robert said: we have to be doing this for ourselves. We don't want to get rid of ourselves. We have things we can't do without some help. I know great art when I see it, but I can't produce great art. Somehow these latent abilities we have could be made more explicitly with the rich assistant. So something AI could do is just amplify us, to express ourselves. Which I think is what we want to do anyway.
Choi: Personally, I have two things I'm excited about. We have been talking about curiosity. What's fun right now is curiosity how to read things in the learning paradigms so we might be able to create things that do amazing things we never could have done before. There's that intellectual gratification. I would like to stay optimistic we can go very far. And then I have this other part of me, so equity and diversity is really deep in my heart. I'm amazed by this opportunity I have to make the world better in that way. improving biases people have. We might really be able to help people to wake up by creating AI that helps them understand what sort of biases they have. I'm excited to see what happens next year.
Tversky: I see lots of AIs here. AIs that are trying to emulate people. There, I worry. Are you trying to emulate the mistakes we make? Or are you trying to perfect us? That's one set of AIs. I see others that seem to be acting as tools and worry about implicit bias in those tools. The creativity, the music, the poetry, all of that is helpful. I study human behavior. I'm always interested in what the AI community can create. Those issue have always been eye-opening to me.
Kahneman: I first became interested in the new AI when I talked with Demis Hassabis, and I am really struck by how modest he appears to be now than six or seven years ago. It's a wonderful hope, it seems to have become actually more distant in the last few years. Another observation: about the apprentice, and the notion humans should remain in control. Here there is real room for pessimism. Once AI or any system of rules competes with human judgement, eventually there will be human judgement, and the idea they actually need a human, probably not. When there is a domain that AI masters, humans will have very little to add in that domain, and that could have very complicated and I think painful consequences.
Tsao: My hope is to understand the brain. I want AI and neuroscience to work together for the simplest and most beautiful explanation for how the brain works.
Marblestone: I hope it will amplify human creativity. The most advanced scientists will be able to do more than they could, but also some random kid could design a space station. Maybe it will get us a universal basic income. And maybe it will be our descendents of humanity going out into space.
Koch: As a scientist I look forward to working with AI to understand the brain. To understand these fantastic data sets. But as Fei-Fei emphasized, much of AI is naive. AI has accelerated and tech is part of the reason for inequality, the growing distrust in society, the tribalism, the strife, the social media. I was surprised today when we talk about ethics. I hang out with people who work at State departments. People who work with military laws. There's a big concern with militarization of AI. People are trying to negotiate treaties on should there be a human in the loop when we build killer robots, as is happening. What about the growing threat of militarization ? Can we do anything about it? Is the genie out of the box?
Kidd: I don't think I have anything to add. This isn't exactly the answer to the question, but I think what I want from AI is what I want from humans: a different understanding of what AI is, and what it's capable of. Take the example of vaccines, and who gets them, and the decision making process. They pointed to the algorithm. But people are behind algorithms. What we really need is a shift from AI as something separate you can point to and blame to holding people accountable for what they develop.
Mitchell: I suppose we are near the end, I want to ditto a lot of what people have said. My goal is to find things interesting and fun, and we are very good at post-hoc rationalization as academics. But fundamentally part of what we are doing is entertaining ourselves. There's this self-serving pull that's creating a lot of this. That is worth flagging. It's nerding-out. The thing I hope AI can be good at is correcting human biases. If we had AI capable of understanding language used in performance reviews, it could interject in those discussions. I would love to see AI turned on its head to handle really problematic human biases.
Rossi: I have to follow up on what Margaret said. The practical terms of AI solving difficult problems, and it can help us understand ourselves. But I think what was just said was very important. From my point of view, I never thought so much about my values before working on AI. What AI systems should be used for, and not used for. To me, it's not just my own personal reflection, the whole society has the opportunity to do this. Those issues around AI, a way to reflect on our own values. Our awareness of our own limitations. Being more clear about what are our values.
Calo: I want costs and benefits of AI to be evenly distributed, and I want the public to trust that is what's being done, and I think that's impossible without changes to the law.
Marcus wrapped up the discussion by saying his very high expectations were exceeded. "I especially loved this last question." Marcus brought up the "old African proverb," that "It takes a village."
"We had some of that village here today." Marcus thanked Boucher for lots of work organizing the event, promoting, etc.
Boucher made a closing announcement, calling the discussion "hugely impactful." Boucher said the next debate, AI Debate 3, will be the same date next year, but in Montreal. Face to face, said Boucher, "That is our hope."
And that's a wrap!