Big Data: a matter of opinion
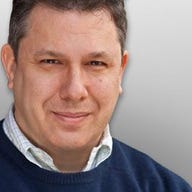

Last night, I moderated a panel discussion, "Enterprise Insights: Go Big (Data) Or Go Home." About 80 people paid $5 each to attend the event at Microsoft’s offices in Manhattan, exceeding my expectations and those of my co-organizers, Yuriy Michael Goldman and Conrad Wadowski who lead the New York Business Intelligence and Enterprise Tech Innovation Meetups in NYC, respectively. The audience consisted of IT and marketing workers as well as a large group of data analysts. The audience was engaged and interested…I might even say concerned; getting a better understanding of Big Data is seemingly an urgent priority for a great many professionals.
Who’s Who
The panel included representatives from the biggest companies in Hadoop, Open Source BI, NoSQL and Data Analytics. Specifically we had Richard Daley, co-founder and CSO of Pentaho, Patrick Angeles Director of Field Architecture at Cloudera, Edouard Servan-Schreiber, Director for Solution Architecture at 10gen (the company behind MongoDB) and Kathleen Rohrecker, Director of Marketing at Revolution Analytics (the principal company behind the R Project).
Questions
As a primary objective, I wanted to investigate the commonalities and conflicts between Big Data and Business Intelligence (BI). Are the two fields essentially the same, or is Big Data replacing/disrupting BI? Why are the tools so different and why are the practitioners different too? Will Big Data technologies ever have the enterprise readiness that BI products do right now? Secondarily, I was especially interested to hear each panelist’s definition of Big Data and where to apply it. I learned a lot form the panel, both about the issues at hand, and differing industry attitudes around them.
Can we all get along?
Panel members seemed to agree that BI and Big Data were complimentary and would coexist. I was surprised that there was such consensus on that key point, so I pushed a bit further. I asked if the current popular model of using Hadoop to process gobs of unstructured data, and then push the results into conventional data warehouse and BI systems for analysis, was a temporary stop-gap or a permanent necessity.
Pentaho’s Daley saw the approach as natural and sensible; Cloudera’s Angeles was far less convinced. Servan-Schreiber of 10gen saw the whole process of moving data to specialized analytical databases as inefficient, at the very least and, for a growing number of customers, simply unacceptable. Certainly, MongoDB’s new Aggregation Framework, which allows for in-situ analysis of data in an operational NoSQL database, is consistent with this point of view.
Defining Big Data
Each panel member had a different definition for Big Data. Cloudera’s Angeles defines it (rightly so, in my opinion) as work with data of a scale where traditional technologies break down, or cease to be effective. Servan-Schreiber explained that 10gen measures the Bigness of Data by its velocity, the scale and performance it demands, and the need for real-time analytics.
Also Read: Big Data: Defining its defnition
Revolution Analytics felt more comfortable talking about getting the best analytical value from data, regardless of data set size (thereby sidestepping the question somewhat). Pentaho puts a sale in the Big Data bucket if it's for a deployment that runs on top of Hadoop, NoSQL or a Data Warehouse appliance like Vertica or Greenplum.
To Hive or not to Hive
Another difference of opinion centered on the way BI tools and Big Data integrate. Daley of Pentaho felt strongly that jamming Hive in the middle to make the two talk to each other is not sufficient. Cloudera's Angeles felt that Hive works very well, going so far as to deny my accusation of it being "Rube Goldberg." 10gen finds it inappropriate to impose the SQL query paradigm on unstructured data, but also felt that writing MapReduce code in Java was impractical. In incontrovertible support of that point, Servan-Schreiber asked audience members if they had MapReduce skills or experience and only 2 hands went up. Revolution’s Rohrecker, meanwhile, didn’t have too much to say on the Hive issue. And given the option to write MapReduce jobs in the R programming language, that makes perfect sense to me.
Guild membership required?
One of my last questions, addressed primarily at Rohrecker, was whether analytics work would remain in the hands of data scientists and other specialists, or if it would become more accessible to downstream business users. Rohrecker felt that such work is genuinely difficult and not readily delegated to non-specialists. Others on the panel seemed to agree. It was striking to me that we ended with strong consensus, just as we had started with one, especially because I happen to think that analytics capabilities will be brought downstream to business users, and probably in the short to medium term.
In general, it’s clear that different data economy “subcultures” have different definitions of Big Data and different opinions on how best to work with it. The different opinions and definitions are in large part self-serving. None of that is surprising, but it is a hallmark of fragmentation for an early-stage technology cycle. I would expect to see companies, opinions, approaches and ecosystems coalesce and, in part, commoditize. But from yesterday’s panel, it’s clear that cohesion is still a ways off.