Can Big Data operations be manageable? Two companies say yes.
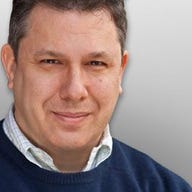
It's all well and good that Hadoop distribution providers include management consoles for their software. Cloudera offers its Manager product; Hortonworks (along with other ODPi distros) uses Apache Ambari and MapR now provides its MapR Control System. These tools aren't bad, but they're designed primarily for cluster-level operations and metrics. They're not really there to help you manage individual jobs or determine performance over time of scheduled, operationalized jobs.
The relational database world does have tools that do that though. What's more, they augment, rather than duplicate, the functionality in the vendors' own management tools. In the Microsoft SQL Server world, for example, Microsoft's own SQL Server Management Studio is a very capable tool, offering a slew of management reports. But that doesn't stop companies like Dell (formerly Quest) Software, Red Gate Software, Idera and SQL Sentry from offering huge suites of their own, all of which add considerable value.
The Big Data world needs tools like this too. And today, it's getting them.
Unravel, in a good way
After raising a Series A funding round of $6.5M in December, a Big Data startup called Unravel Data is emerging from `stealth today. Unravel is dedicated to simplifying data operations in the Big Data arena, helping Big Data and IT teams cooperate and collaborate in a fashion similar to the way developers and IT do with DevOps tools (in fact, the company uses the term "DataOps" to refer to the need it addresses).
The company's products don't merely monitor jobs but, according to Unravel Data's founder and CEO, Kunal Agarwal, offers automated root cause analysis for when things go wrong. Unravel also offers guided remedy -- troubleshooting support that is algorithmic, machine learning-driven, with rule- and cost-based optimization as a foundation.
Agarwal says that while everyone wants to run Big Data technology, people are lost in managing it. Most data folks don't know distributed computing and vice-versa. The result, when something goes wrong, is everyone picking through logs and crudely testing theorized diagnoses until the problem is remediated. That just doesn't scale, and Unravel aims to address that need.
StreamSets thinks SLAs
StreamSets is a one year-old company dedicated to helping companies manage the intake of machine-generated streaming data (especially relevant to IoT) and even batch data intake as well. StreamSets was founded by Girish Pancha (CEO), former chief product officer of Informatica, and Arvind Prabhakar (CTO), a former engineering leader at Cloudera. This combination of Enterprise BI/ETL and Big Data pedigrees logically produced a company that is focused on bringing Enterprise-grade manageability to Big Data.
Rather than just provide a platform for streams and stream processing, StreamSets allows data teams to establish and track operational KPIs (key performance indicators) for data flows, and will alert operations personel when performance degrades. This has been facilitated by the company's Data Collector product, which was often embedded by ISVs in their own products.
DPM Dashboard, with topology Sankey diagram (Source: StreamSets)
Today, StreamSets is introducing its new Dataflow Performance Manager (DPM) which aims to provide this manageability around end-to-end data flows, following data from its source all the way to its destination data lake or other Big Data repository. This facilitates management of data lineage, creation of SLAs (service-level agreements) for these data flows and remediation of SLA violations. StreamSets expects DPM to reach GA (general availability) in time for the Strata and Hadoop World event in New York City the week after next.
Will it play in Peoria?
Let's be honest: the early days of Big Data were dominated by companies and products with an "oohs and ahs" go-to-market strategy. And if that's what customers' palates are set for, then tools to help manage and troubleshoot data flows, and guarantee their SLAs may not be big crowd-pleasers (although Sankey diagrams never hurt).
But Big Data is past its infancy. The technology is deployed, and expectations around ROI and competitive velocity have been set sky-high. Meanwhile, those expectations often go unmet, the technology is hard to use, and even harder to manage. That's causing real pain and stress. And, if we're continuing to be honest, successful products are the ones that address such pain points.
Perhaps neither of these companies can do that, but I think they both will. Their leaders have the right motivation, the right priorities and, most important, the right values to create products that make Enterprise Big Data avoid being a contradiction in terms.