Cloudera Enterprise 6 hits the streets
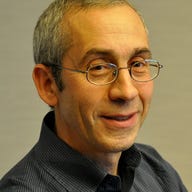
Video: How LA is using AI and maching learning to better understand traffic
Coming in the midst of a transitional year, Cloudera is announcing at the Strata London conference this week general beta release of the 6.0 release of its platform after an extended beta. For the new release, Hadoop 3.0 is the star of the show.
We reviewed Hadoop 3.0 at the beginning of the year. To recap, the 3.0 Apache Hadoop release marks a major watershed for the platform, as it starts to address the information lifecycle with a feature with a very geeky name: Erasure coding.
TechRepublic: Apache Hadoop: A cheat sheet
Erasure coding is a key feature of established RAID technologies. With Hadoop 3.0 embracing this feature, the Apache community is admitting that Hadoop won't be exempt from the laws of gravity of enterprise storage.
The background goes thusly: when Hadoop emerged, the idea was that, even with data replicated 3x, that storage would be so cheap and scale-out compute so linear that you wouldn't have to think about limiting it. Kind of like the outdated maxim that nuclear power would be so cheap that you wouldn't have to meter it.
Well, maybe the universe may seem infinite, but at some point, there's a limit to storage sprawl. Even in the cloud, as we noted a few months back, too much cheap eventually becomes expensive.
Erasure coding means you don't have to replicate data 3x; the actual footprint for Hadoop 3.0's implementation of it decreases by roughly half. Of course, there's always a tradeoff. Erasure coded data is essentially near-line data, meaning you can access it for your computation, but only after you restore it.
Cloudera Enterprise 6 also embraces Hadoop 3.0's new capability to federate YARN so that multiple YARN engines can allocate compute across different, partitioned parts of the cluster. There's a similar enhancement to NameNode - instead of just one standby NameNode, you can have as many as you want. That feature doesn't necessarily scale out compute or storage per se, but it makes failover far more robust.
Other highlights of Cloudera 6 include support of Apache Kafka 1.0, roughly six months after it went GA. That reflects Cloudera's strategy not support bleeding edge, but instead support new open source features once they've stabilized. The same goes for Solr 7 released last summer, which is the crux of Cloudera Search. In this case, Cloudera made up for lost time as it was previously on the 4.x Solr version. The highlights of Solr 7 include a choice of index updating modes. For highly scaled deployments, you can have multi-master like capabilities that allow any local replica to become the master (this speeds up search indexing for scale-out deployments); a Paxos-like capability where the master is chosen by a consensus; or continue with the existing master-slave mode where index updates are pulled from a master.
Cloudera 6 also makes the jump to Spark 2.0. And there will be tighter integration of Spark (and Kafka for that matter) compared to previous versions, which will simplify managing data pipelines. Supporting Hive 2 means that Cloudera 6 leverages the vectorization that improves Hive performance up to 80 percent, while supporting Ozzie 5.0 adds the ability to schedule recurring jobs.
All this comes in a year where the company is doing a major pivot. Like each of the other Hadoop vendors (and conferences), most are taking Prince-like strategies that are, in effect, adding the tagline of the company-formerly-known-for Hadoop. It is due to the realization that, like any enterprise technology investment, big data needs more of a business focus to get buy-in beyond the corporate center of excellence unit or data science team.
Then there is so-called identity issue: are these folks really selling Hadoop, and just what is Hadoop anyway? Hadoop was originally defined as scale compute (MapReduce) and storage (HDFS). Yet today, Cloudera and Hortonworks are each selling cloud-based Platform-as-a-Service (PaaS) offerings that slot in cloud object storage in place of HDFS, and their offerings are increasingly being optimized on Spark compute.
Then there is the fact that Hadoop is no longer the sole path to performing big data computations; there are streaming data analytics pipelines, not to mention dedicated services for Spark, machine learning, and deep learning that are providing alternate paths to big data analytics outside the components of Apache Hadoop.
Capping things off for Cloudera is that the company has had to make some tough decisions to get on the road to profitability. The key to the business for Cloudera and its big data platform rivals like Hortonworks and MapR is that the sales cycle is like that for any enterprise system: it is long and only gets profitable at renewal time when the footprints grow - the so-called land and expand strategy,. As a public company, there's no secret to Cloudera's numbers. Sales were growing nicely, but there was a realization that too many of them were one-shot deals. So Cloudera decided to take the bitter medicine this year. Share prices have recovered modestly after taking a sharp dive after release of final FY18 results back in April as the company strives to up its game managing Wall St. expectations.