Cognitive chatbots in customer service: Beyond the hype and behind the scenes
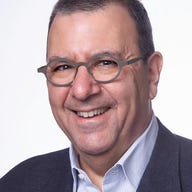
AI-based chatbots are all the rage today, with market growth estimates in the range of 24 percent to 37 percent. As a result, the market is ripe with outlandish claims that chatbots will "revolutionize" enterprise software and similar hype.
For enterprise buyers, the challenge is seeing through this marketing hype to find what's real and works.
Featured
Cutting through this hype is an important part of the mandate of CXOTalk, a series of in-depth conversations with leading thinkers on innovation, artificial intelligence, and digital disruption.
On episode 257 of CXOTalk, Chetan Dube, CEO of AI software company IPsoft, explains important issues to consider when designing and buying products. Chetan has been building cognitive systems for two decades, and I have had lengthy discussions with large IPsoft customers, so I know the company's products are real.
During the conversation, Chetan explains the ideas and challenges associated with developing artificial intelligence to create chatbots for customer service. Among the topics we explore are:
- The Turing test as a design goal
- Role of digital labor
- Measuring the value of cognitive systems in customer service
- Attributes of a cognitive bot
- Techniques for emulating empathy and emotion
- Ethical issues associated with digital labor
- Public policy issues related to artificial intelligence
You should watch the entire conversation in the video embedded above and read the complete transcript. An edited summary of key points is below.
Who uses digital labor?
The new digital workforce provides significant ROI and NPS score benefits to our customers and humans are getting elevated to train and teach this new digital workforce. We have seen rapid adoption, particularly in the finance and insurance verticals.
Banking, for instance, is very aware of how their low asset, high friction, high margin areas are progressively facing digital attackers with few assets but providing equivalent solutions. So, big banks are reacting with an aggressive strategy as opposed to defensive posturing. They are building up their digital portfolio.
The healthcare industry is also rapidly starting to adopt digital labor solutions for improving both the patient and the caregiver experiences. Retail is also following the trend in a big way.
Your AI product is called Amelia. What were the design goals?
The design goals have been continuous over the past 19 years. The question Turing asked when he said, "I propose for you to consider the question, can machines think?" has haunted us. Our design goal has always been [to answer the question], "Can we make thinking machines possible and what it would take to build a real human equivalent thinking machine?" Does it need to emulate all the different aspects of neocortical activities or can we imitate that? That has always been the guiding force behind Amelia's design.
How can buyers evaluate digital agents in customer service?
The industry is asking for good net promoter scores: the number of promoters minus the number of detractors should be in the positive. The number of people who want an experience with an intelligent agent or chatbot, versus the number of people who want to be taken to a human, should be higher.
You almost always find dissatisfaction with chatbots because they're basically not intelligent and you find the number of promoters is less than the number of detractors who want to be taken away from a chatbot and forward onto a human customer service agent.
Our goal principles are positive net promoter scores, people wanting to talk to an intelligent agent. We want the intelligent agent to solve problems for the customers. You have to ask yourself, "What does it take to deliver these intelligent solutions?"
First, you have to understand what a customer is saying semantically. Right now, what we are discussing is good. It's being vectored into the entire audience of this premier talk into their hippocampus, semantic store of all facts. It is also registering into episodic, event-based memory, which is the collection of all other CXOTalks they have seen and all the other supporting documentation they have seen around the topic of cognitive and artificial intelligence.
It's also going into their process and analytic and affective memory. They have an emotional connection to this topic, about the implications of cognitive and digital labor solutions on the social and demographic and the neo-Luddite movement. All of those things.
Only then, can the audience compile a thought like, "What would be required for us to deliver a better solution that meets the demand of the industry?" That's real neocortical emulation as opposed to a typical chatbot, which is bucketing what you say into one of the IVR-esque [interactive voice response] buckets and providing you a canned response.
Colin Crook on Twitter asks about empathy in artificial intelligence?
It's an insightful question. Thank you, Colin.
I think McKinsey had research saying that better net promoter scores are dependent on the emotional connection of the agent with you.
How do we achieve that emotional connection with the customers that are being serviced?
You must have EQ vectors tail the exact EQ vector of the customer. The integration of EQ vectors is the mood vector, which is not as inflective or seasonal, but you take the integration [of all these]. The mood vector of the cognitive agent needs to be tailing that of the customer being serviced. That integration of all mood vectors are the personality vectors.
Three-dimensional PAD / OCC models with emotional, mood and personality vectors give you the ability to make your agent behave in a human-like way: having an affective reaction, an empathetic reaction, to the person it is serving. Sentiment analysis -- in both the textual and inflected nature of their tone and tonalities -- allows us to do that with a high degree of precision.
Today, over a few hundred Global 2000 companies employ digital agents. The largest mobile carrier uses this to examine sentiment during an interaction with the customer. If the customer sentiment score rises to a certain level, for example], where it exceeds the top ceiling, this may eventually be a good opportunity to upsell this customer.
If the customer sentiment and discord registered in our interaction falls beneath a certain floor, a trap automatically is made to send this customer over to another human agent or a supervisor to intervene.
What about the ethical dimensions of cognitive machines interacting with humans?
You struggle with that. Definitely.
There are two schools of thought. The utopian school of thought says this will do everything from cure cancer to eradicate poverty and hunger and water problems. The other is the dystopian school of thought, the Musk and Hawking club that believes that this is going to be the final invention known to man.
I am a subscriber to the utopian school of thought. But, while there's an active debate going on in the community between the utopian, is it going to be a good thing or is it going to be a bad thing, I ask the third question, "Do we have a choice?" Do we have a choice? Will time and tide wait for anyone? I ask you, will time, tide or technology wait for anyone?
I have yet to meet a single CEO who says, "Oh, yes, we can drive 45 percent benefit to our shareholders, and we can get improved customer experience and operational efficiency. Yeah. I'm going to walk away from it."
History has proven that technology moves forward. Some may argue, as you did, Mike, that we have a tiger by the tail, but we are going to move into this thing. It's going to come.
How do we prepare for it so that man can thrive in a world where digital laborers take care of mundane chores that pull us down, and man can elevate himself to higher forms of creative expression? That's the thought to which I subscribe.
Christian Pescatore on Twitter asks, "How do you advise an enterprise buyer who purchased a simple chatbot instead of a cognitive agent?"
The AI community has put lipstick on that pig. Forgive my directness. We have put a thin layer of DNN classification, deep neural network classification with support vector machines.
We take natural language input but still try to bucket it into 4,000 buckets in the back. If you ask atomic, simple questions, it works. It gives you that impression because its horizontal sweep expands to that extent; it gives the impression that you're talking to an intelligent agent:
- Hey, how is the weather?
- Can you book me a flight?
- Can I get a hotel reservation?
- Can I go Chanterelle, a French restaurant?
- What is the score of the preseason game between the Knicks and Nets?
All these work. However, ask questions of your claimed chatbot solution. Ask, can it read? Put it to the test of a ten-year-old. Can it read a standard operating procedure for your company? Can it understand what it has read? Can it solve problems from what it has read? Can it create an empathetic connection to my customer based on what it has read? Can it deliver to me net promoter scores that are positive? "Please switch me to a chatbot," said nobody ever.
If you are in chatbot land, the only advice I can submit to you is, and please forgive my directness: abandon it. It is a dead-end street. You are never going to get customer value creation there. You need an intelligent agent that mimics human behavior.
Thumbnail image by Peyri Herrera, Creative Commons on Flickr. CXOTalk brings together the most world's top business and government leaders for in-depth conversations on AI and innovation. Be sure to watch our many episodes! IPsoft has been a CXOTalk underwriter in the past.