Data Works, Hadoop 3.0 is round the corner, and 'Horton Hatches the Egg'
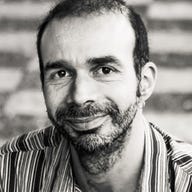
This week Munich was host to DataWorks EMEA, one of the major big data events in the region as well as globally. The event, organized by Hortonworks and Yahoo, featured the latest and greatest from the world of open source innovation in big data, as well as a plethora of use cases from the field and the opportunity to get an in-depth view of Hortonworks' current status and future plans.

Hadoop is moving forward
Hadoop 3.0 is round the corner
To begin with, what could be more exciting to the Hadoop-dominated big data space than a new major version of the fiery beast? Hadoop 3.0 has been in the works for a while now, and although that's no secret, it's not exactly common knowledge either.
In any case having the chance to get a sneak peek on what's cooking, and by one of Hadoop's founding fathers no less, is not to be missed. So when Sanjay Radia spoke, everyone in the room listened.
Radia has co-founded Hortonworks, is part of Hadoop's PMC as well as the architect of HDFS. Unlike what you might expect of a co-founder at a company of Hortonworks' magnitude, but like many of his colleagues there, Radia is still very much actively engaged in engineering. His talk was dense, advanced, and algorithmically focused.
Hadoop 3.0 has been in alpha for the last few months, but despite the fact that there's no publicly disclosable release agenda yet, setting GA for Q4 in 2017 may not be unrealistic judging from what seems to be an advanced state of implementation.
The key advances in Hadoop 3.0 are centered around resource management and container support in YARN (Yet Another Resource Negotiator) and replication in HDFS (Hadoop Distributed File System). Radia glossed over a number of improvements in the works there, each of which would require quite an analysis.
The takeaway and the promise however is that while Hadoop 3.0 may not seem to represent such an obvious and major move forward as Hadoop 2.0 was in comparison to its predecessor, it will be more flexible, powerful, and resource-aware.
One set of improvements have to do with the way HDFS works. While erasure coding probably means nothing to most but a select few engineers, the implications of its introduction in HDFS will make many people happy -- from CXOs to Ops and beyond. Erasure coding is an advanced technique for managing I/O and replication in distributed file systems, and by introducing it HDFS will become more reliable, save in disk space and bandwidth.
Erasure coding parallelizes write/read operations in the file system, resulting in lower latency. The tradeoff is higher network bandwidth utilization and higher reconstruction cost in case of failures, plus the higher cost of implementation. This is why Hadoop 3.0 will begin by supporting relatively simpler flavors of erasure coding and then go from there.
Another set of improvements is related to the way YARN works and what it can support. YARN is Hadoop's resource manager, and its introduction in Hadoop 2.0 was key in making Hadoop clusters run more efficiently. Now YARN is coming of age, adding an array of improvements in areas like scheduling, support for long-running services, elasticity, timeline services and -drum roll- containers.
While all the other features are important, but hard to explain to non-engineers, containers enjoy the kind of hype that is sure to turn some heads. Support for long-running services for example was driven by the need to consolidate infrastructure. Simply put, YARN should now be able to manage resources and services that run even beyond a Hadoop cluster. That also includes Docker containers, but there's more to it than hype and ticking boxes.
It means anything that can run in a container can now run in a Hadoop cluster. Anything from Tensorflow to YARN itself -- and these are actual examples Radia referred to. This feature is used internally at Hortonworks as a replacement for Openstack.
Instead of managing and configuring resources via Openstack, Hortonworks engineers are using this YARN-on-YARN approach to spin a cluster within a cluster, an approach that can be scaled both vertically and horizontally. Radia mentioned that they have found this to be cheaper, more reliable and robust than OpenStack.
Horton Hatches the Egg
"You wanted Docker support, we heard you," was Radia's message. But there's a story here which may shed some light on Hortonworks broader strategy.
Like the hero of the book it is named after, Horton the elephant, Hortonworks perseveres. Imag: Wikipedia
Offering support for container persistence was something that MapR recently did, and looked like an obvious move for any vendor aspiring to win hearts and minds among the growing ranks of container users.
MapR built this on Mesosphere, and according to both MapR and Mesos, it did not require any special integration. So how come Hortonworks for example did not go for it too?
Having just watched that Hadoop 3.0 intro, it was an obvious guess: if containers are coming to Hadoop, there's not much need for Hadoop to go to containers anymore. When discussing this theory with Scott Gnau, Hortonworks CTO, he alluded to it: "Containers... hmm, I was not sure we're actually discussing this at this point."
Apparently, Hortonworks has a lot going on, and not everything comes to light loud and clear. Hortonworks culture, deeply rooted in engineering and open source, may have something to do with it.
Like Horton, the elephant hero it is named after, Hortonworks has chosen to stick to its ways in the face of criticism. But now it looks like it may have hatched that egg and wants the world to know. Hortonworks has some new recruits in place with the goal of continuing to execute on its strategy while ramping up a couple of messages.
One, we're doing great, thank you very much, and we'll do even better in the future. Hortonworks is sporting a few numbers there -- 1000+ customers and 2100+ partners worldwide, the first software company to reach US$ 100 million in annual revenue in 4 years.
When asked if they see the company as being on the way to achieve the $1 billion landmark, Hortonworks executives responded that this is what their new recruits and ramping up in terms of outreach are all about.
Two, the fact that we're focusing on getting the basics right first does not mean we are not paying attention. In analyst panels and 1-on-1 discussions Hortonworks emphasized its focus on data in motion and at rest, security and governance, but also went to some lengths to discuss key industry trends and how they are addressing them.
- IoT: we're there, with Ni-Fi and Mini-fi.
- Cloud: we're there, with AWS and Azure, and more coming.
- Streaming: we're there, with Storm + Spark.
- AI: we'll be there, data is the substrate.
Three, we have our way of doing things and it's working out great. Hortonworks has a new President & COO, Raj Verma, who recently joined from TIBCO. Verma is outspoken about a number of things, and coming to Hortonworks from a 23-year career in proprietary software, his views on what has impressed him the most so far are interesting.
Verma notes that the pace of innovation and level of community participation he sees are staggering, and that people are getting more for their buck.
Data works, and open source has its heroes
Case in point, Data Works featured a number of high quality sessions from contributors and users, ranging from deep dives in advanced technical solutions to overviews of business cases. Among those 1000+ Hortonworks customers, some were featured and awarded as "Data Heroes".
Data Heroes: Raj Verma (Hortonworks), Daljit Rehal (Centrica), Tobias Bürger (BMW Group), Rene Castberg (DNV GL), Joe Morrissey (Hortonworks). Image: Hortonworks
BMW Group is in the automotive business and was awarded for its architecture, featuring Hortonworks Data Platform (HDP) as one of the enabling technologies. BMW leverages this architecture internally to manage structured, sensor and server log data and produce batch, interactive SQL, streaming and AI/Deep Learning analysis that power over 100 use cases for 125K employees worldwide.
Centrica is an energy and services company and was awarded for its vision. Centrica manages structured, clickstream, sensor, geo-location, server logs and social media data to produce batch, interactive SQL, search, streaming and AI/Deep Learning analysis. Centrica leverages data collected from its application to understand customer history, needs and overall satisfaction level and provide more accurate smart energy bills.
DNV GL provides services to the maritime, oil & gas and energy industries and was awarded for its application of data science. DNV GL developed a platform called Veracity for its customers to place their data. Most of that data is sensor-related from large physical assets like vessels, rigs, turbines, pipelines and grids. Veracity provides customers with user friendly data quality metrics, emphasizing on data quality and lineage.
Internet of Things
These are just some of the use cases presented, many of which were impressive in terms of time to production or collaboration and community-driven approach. For example, Danske Bank reported going from little or no analytics to predictive analytics in about a year. Hortonworks engineers reported how their collaboration with Netflix resulted in achieving performance parity with Amazon's own EMR using S3 on AWS, and being on their way to superseding it.
So while MapR may rejoice in getting the lead on say container persistence for a while, Hortonworks knows they will be catching up soon leveraging the open source community. And while Cloudera may be moving up the stack, Hortonworks is very skeptical of this move, going as far as to claim it's a sign of weakness. Of course, not everything is rosy in the Hortonworks world either.
In streaming, Hortonworks' offering and message are somewhat convoluted. Hortonworks is mostly behind Storm, which is not seeing the kind of traction Spark is seeing or championing a different approach like Flink. Hortonworks is shipping both Storm and Spark, and also helped incubate Flink. Confused? Asked to comment on their strategy, Gnau mentioned that they have to keep providing support for their users regardless what their choice may be.
In data science and AI, Hortonworks is much less vocal than the competition. In terms of their strategy there, Gnau was reassuring: it's on their radar, and we should expect to see something from them in this space soon. How soon? When they feel the time is right. This is not exactly definite obviously, and it may be too late for Hortonworks to catch up by the time they decide to make a move.
But the issue of differentiating strategy among vendors merits further analysis, and we will return to this in future posts.
How can you turn big data into business insight?