Strata: Cloudera, MapR and others focus on consolidating the sprawl
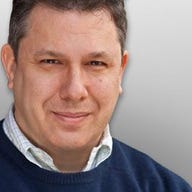
When Strata + Hadoop World comes around in the US, once a year in New York and once a year, as it is now, in San Jose, CA, it's a bit of a news feeding frenzy. I'm not even at the event; I was merely pre-briefed by four vendors, last week and yesterday, with announcements they're making this morning. And still, it's a lot.
Cloudera told me about its new Data Science Workbench and Pentaho told me about what it's doing with data science. MapR briefed me on their edge computing product, MapR Edge. And new player Iguazio told me how it's built a continuous data platform that unites most processes of the data lifecycle, in parallel and in near real-time.
At times like these, I roll the news up into a single post, and I look for a connecting theme. I do that by necessity, to make the post readable. But doing so can also provide a good analysis of the event, or even a point-of-time thumbnail of the industry.
So here's what I found out: after years of the Big Data community belting out numerous open source processing engines, multiple formats and structures for data, umpteen machine learning libraries and numerous streaming data platforms, on premises and in the cloud, "on the metal" and in Docker containers, it is now focused on consolidating the sprawl, and cleaning it up.
Cloudera, data scientist
Let's go in order. Cloudera, which has been building out its value-add on Hadoop, first through the Hue console and later with its Manager, Navigator and Director components (for administration, governance and deployment), is now extending that coverage with its Data Science Workbench. Recognizing that most data scientists and data engineers (assuming, for the sake of argument, that you buy into that taxonomy) do a ton of work with R and Python, often inside notebook environments like Jupyter, Cloudera has taken the technology it on-boarded through last year's acquisition of Sense.io, and brought it into Cloudera Enterprise as the Data Science Workbench, now in Beta.
Much as Hue lets customers examine and manipulate data on their Hadoop clusters, Data Science Workbench allows Cloudera customers to perform data science work in what we might now call Cloudera's IDE (integrated development environment). Data Scientists can collaboratively work on the same code, then define scheduled jobs to run that code and operationalize data science workloads. The open source Feather project, affiliated with Apache Arrow, allows data to be exchanged between Python and R (overcoming their differing data frame formats). And Jupyter notebooks provide an environment for code, documentation and visualizations.
An R session in Data Science Workbench, running on Spark, via Sparklyr
Data Science Workbench runs in a multi-tenant Docker/Kubernetes environment, and it integrates with Cloudera Navigator and Apache Sentry. Its user interface, the code for which is hosted on an edge node, is intentionally GitHub-like in look and feel. And in keeping with Cloudera's "open core" approach, Data Science Workbench is proprietary and exclusive to Cloudera Enterprise, but all of the underlying components are open source.
So, if Cloudera can tie together Python, R, Feather, Sentry, Jupyter and Docker, what can other vendors do to match that? A lot as it turns out. The story continues.
Pentaho does data science
First, let's take a look at Pentaho. The company long ago introduced something it called its Data Science Pack, based on its open source project, Weka, and integrating it with its Pentaho Data Integration (PDI) platform, also based on an an open source project, called Kettle. Subsequently, the company added features like metadata injection and went beyond Weka, adding support for Spark MLLib as well as R, Python and Scala.
The end result of all this incremental work is that Pentaho has a robust data science platform, and one that's integrated into its mainstream data integration tool. That means the more tactical work of data ingestion, preparation and feature engineering, as well as diagnostic visualization, can be done in the very same environment that can train models and score data against them.
So although this doesn't constitute a new, discrete release, Pentaho is rightly formalizing the announcement of this functionality. Full disclosure: my employer, Datameer, competes with Pentaho. But I have to take my hat off here, because Pentaho's taking an approach to data science that I think is key: it's desegregating it from standalone environments and workflows geared to a specific constituency (data scientists) and featuring it as a related capability in its mainstream data platform. Until the industry, as a whole, does this, data science, AI and predictive analytics, despite the hype, will be rarefied and enjoy limited adoption.
MapR, close to the edge
Cloudera isn't the only distribution vendor with cool announcements. And "distribution" is a funny word to use, because MapR is at once morphing into more of a data platform vendor and, as part of that, is addressing distributed architectures for the Internet of Things (IoT).
Here's the skinny: MapR is introducing a new...well...distribution of its Converged Data Platform called MapR Edge, that can run at edge sites, near where IoT data-generating sensors are installed. Much as I wrote about last week with respect to ExtraHop, MapR is deploying the technology to the edge, so more work gets done before the data must travel over a network to a central cluster and be aggregated, analyzed, modeled and more.
But here's the neat thing about MapR's approach: the stuff running at the edge is actually MapR's platform, including Hadoop and Spark. And it's not running on a single CPU or single box, either; it's running on a true cluster, consisting of 3-5 physical Intel NUC Mini PC nodes.
The MapR Edge topology
Each node in these edge clusters has between 64GB and 50TB of storage; supports snapshots, mirroring, replication; and can run Drill and Hive in addition to the core MapR platform. This re-purposing of consumer and small business technology to make IoT computing more intelligent is pretty innovative in my opinion, and parallels the notion of transforming consumer IoT to industrial IoT in the first place.
Iguazio: data flows like waterfalls
The last bit of news to cover is the Beta release of Iguazio's data platform. Based on a "continuous" (streaming) data paradigm, Iguazio also seeks to consolidate a number of technologies into something integrated and rational.
Iguazio has built its own engine that physically runs over Flash memory but virtualizes that layer into RAM to work like a pure in-memory database. This database uses a multi-model store, based on column family structures, indexed for both optimized sequential and random access. And because of its high speed operation (which Iguazio claims supports 2M transactions per second) and parallel architecture, Iguazio says it can handle data ingestion, enrichment, analysis and serving of data simultaneously.
The Iguazio high-level architecture
Essentially, Iguazio says, it has eliminated the notion, and burdens, of more linear data pipelines and has done so while nonetheless supporting multiple standard APIs, including those for Kafka, Amazon Kinesis and DynamoDB, as well for Spark DataFrames.
As with some of the other products I've discussed, Iguazio also ties together technologies such as Docker, Kubernetes and Spark as well as TensorFlow, not to mention those whose APIs it supports. Watching Iguazio will be quite worthwhile. Its product, which is now in Beta and scheduled to hit GA by mid-year, will be pretty disruptive if it can do what it says and cross a meaningful threshold of adoption. That's a tall order, but one this Israeli enterprise seems eager and ready to take on.
Hadoop's creator looks at upcoming tech that will unlock big data
Come together
The Big Data world really feels like it's in harvest mode now. It's planted many technologies over the years. Now it's taking stock of them, and integrating them into various implementations which, dare I say it, are rather turnkey. That's an excellent trend to see, as it makes the technology far more usable by, and relevant to, the Enterprise. And that's what's needed to get Big Data out of a malaise phase and into ROI-producing levels of adoption and success.