DataRobot aims to automate low-hanging fruit of data science
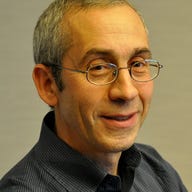
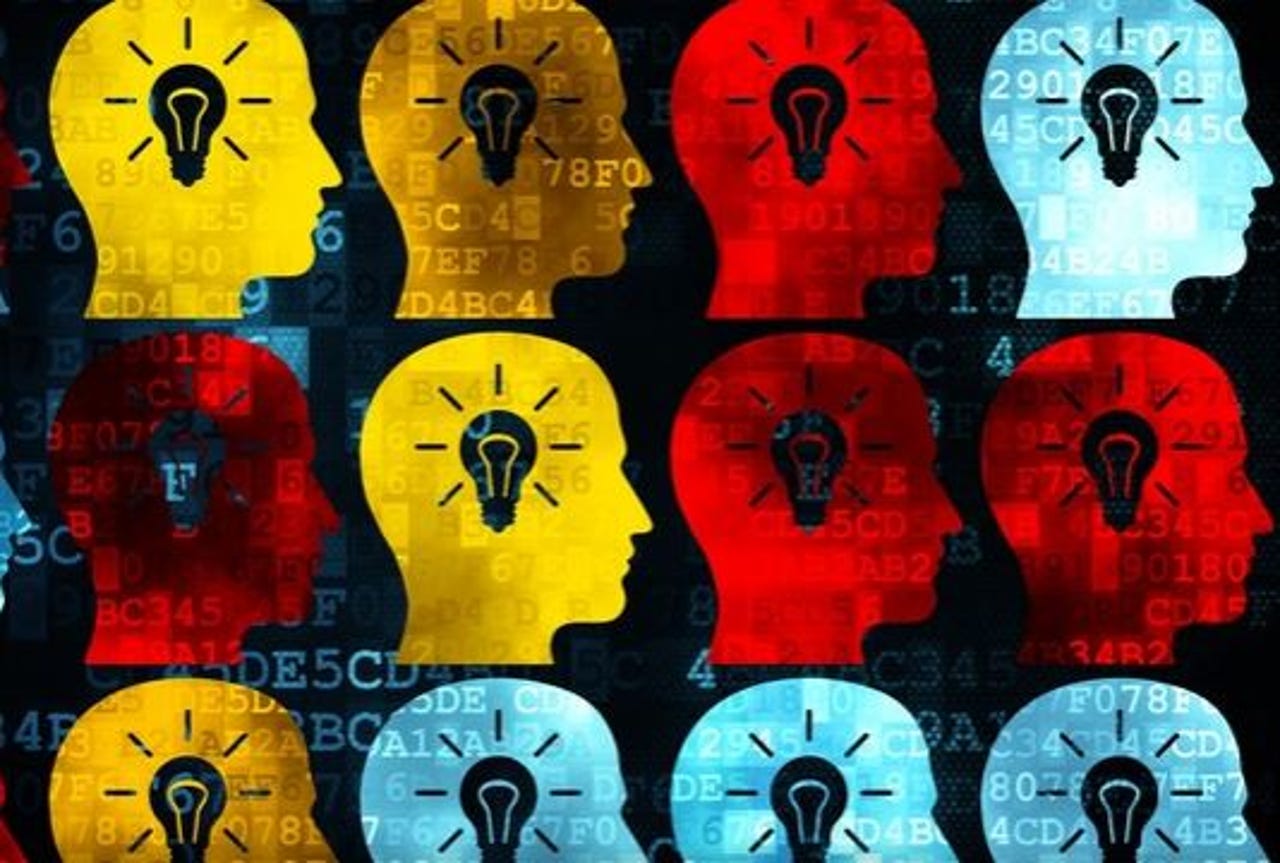
Given a profession that Harvest Business Review has termed the sexiest job of the 21st century, we've often joked that data scientists are so rare and precious, only 35 of them exist in the whole world. As nature abhors a vacuum, it's not surprising that data science programs have become a growth industry in the academic world.
Read this
Answers to the question of "what is a data scientist?" remain elusive; conventional wisdom is that you combine statistical expertise and specialized language programming skills, sprinkle in some domain knowledge along with communications and leadership skills, and voila, you've just defined the human manifestation of unicorn.
But these superhumans have very human problems, as we noted while parachuting into a data science symposium about a year ago. Issues like identifying the right data, the right questions, and then monkeying around with somebody's old spaghetti code and maxxed out compute infrastructure should sound quite familiar to mere mortal colleagues across IT, like the DevOps folks.
Of course, some folks believe that we'll be able to automate our way out of the problem. It's hard not to look at the BI tools of tomorrow, like Watson Analytics, Amazon QuickSight, or ClearStory Data, and believe that artificial intelligence will help us identify those data sets; isolate the signals; suggest the questions to ask (just like you're shopping on Amazon or browsing Netflix); and tell your story for you.
These tools will help make us more productive, but they won't eliminate the need for human data scientists. More than sometimes, you'll just need to adjust course for what the AI algorithm thinks you want to know. And so we'll still need data scientists, hopefully we'll eventually get more of them, and we want to make them more productive.
Not surprisingly, we're witnessing an explosion of tools for making data scientists more productive. They're pretty varied, with just about the only common threads being the tagline, "We're the new competition to SAS." Some help you manage the lifecycle of machine learning projects, while others throw a bunch of machine learning algorithms at you and test which is the best one for the problem, while still others provide development and deployment environments. We'll talk about more of those in coming months, but for now we'll direct our attention at DataRobot.
DataRobot automates the legwork around running machine learning models on your data. With the service, available for private or public cloud, you upload the data and do some "lite" data preparation around it, you indicate what parameter you want to predict, then the tool takes a brute force approach and runs dozens of algorithms on sets, then compares the results on a leader board that is similar to what Kaggle uses to display results of its online competitions (the company employs over a dozen data scientists who've made Kaggle's top 100 ratings).
The audience for DataRobot reflects the ill-defined contours of the data science profession: the company claims that you don't have to be a genius to use it, but the tool is not exactly aimed at business analysts either. We'll later discuss other offerings, such as from Dataiku and H2O, that are aspiring to break through that barrier to promote collaboration between data scientists and business analysts, or Alteryx, which masquerades as a BI tool that lets your R programmers embed advanced predictive analytics and machine learning under the hood to perform some pretty amazing things.
For now DataRobot provides a brute force approach to making data science aspirants more productive. And there's nothing wrong with that.