Do we really need data scientists?
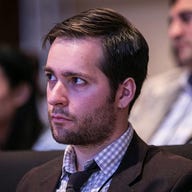
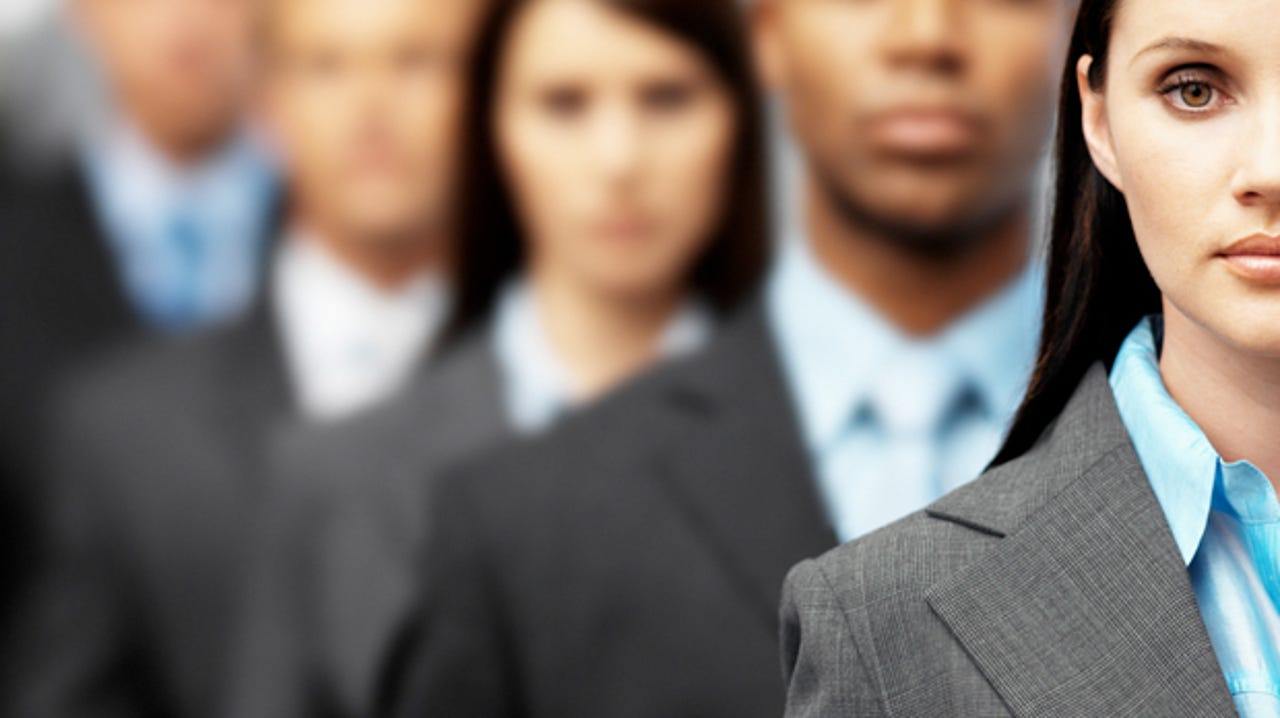
A few weeks ago, I enjoyed a dinner at which the topic of conversation was simple: big data.
At one point, the subject of data scientists came up.
"Is there really a shortage?" one person asked.
"It's even worse than people think," another dining companion responded.
"But really, what is data science? It's not like people went to school to major in it," a third chimed in.
"Exactly," the second replied. "Anyone with 'data scientist' on their resume is suspect."
Later on, that same fellow admitted that he had just hired a "superstar" engineer with this very reason in mind.
What gives?
If you read ZDNet, you know that big data is this year's big buzzword. (OK, it's technically two words, but come on.) Every executive wants to deploy it in their business, even if they're not really sure what it is, how it functions or what it can really do for them. In the enterprise, "big data" is the new social -- meaning it's the word you attach to everything to seem like you're on the cutting edge.
Still, reports of shortages for data scientists -- the wizards to make it all work -- continue. If you thought it was hard to hire away the industry's top software developers, competing for these oracles of insight is exponentially more difficult.
Is it all hype? Are data scientists really necessary (and worth all that trouble)? And for who are they appropriate, really?
I wanted to dig into these questions. So I rung up Stephen Purpura, CEO/CTO of Context Relevant, a Seattle-based company known for its machine learning platform. Purpura recently announced the hiring of three more members of his company's "brain trust," even as he develops a resource to make such people unnecessary for certain kinds of businesses.
In other words, he's a guy who sits on both sides of the hype spectrum. I spoke with him.
ZDNet: Give us a quick introduction to your company before we dive in.
SP: The company was founded in March 2012. We've been working on this for nearly a decade, the business idea that is the company today.
Back in 2001, during the dot-com bust, I was working for Madrona Venture Group that had [intellectual property] in big data. But we shelved it.
I've spent the last decade trying to bring it back. We formed the company after creating the proof-of-concept and we hit the ground running.
What most vendors do is sell an electric set, dump it on the table, and expect you hire a bunch of experts to put it together and solve the problem. These are people who go from project to project, company to company and make a lot of money. This happens in advertising, or Wall Street. It's highly manual work, and a lot of that knowledge is stored in their heads is not encapsulated in tools. Most projects that are successful these days start from scratch.
It turns out that the problems that Wall Street faces are not that different from those that companies in the Fortune 2000 face. The only difference is that on Wall Street, there's a need for speed because speed is money. So they're the front-runner. The needs of high-traffic websites come after that.
Many companies are understanding that a lot of their business depends on understanding the needs of their customers. These types of things are the difference between the haves and the have-nots today. There is growing demand and expectations for results.
ZD: Let's get down to it, then. Data scientists! They're in demand. They're rare. They're expensive. Business leaders think they need them, even if they're not sure what they do. What gives?
SP: The industry believes that a person is a data scientist when they have the ability to visualize projects that make the company money by looking at the data that exists and turn that into a statistical process that predicts whether or not to invest in a customer, or upsell them, or whatever.
These people have statistical skills, business savvy, and elite programming skills. They know how to do things that regular developers don't know how to do. That makes these people very hard to find. How many people do you know have the technical skills of the best developers, but can also visualize projects that make the company money?
That's why our company exists -- we are trying to bridge the gap. Ruby on Rails allowed people who didn't know how to build the structure of a website to build something that basically worked; it wouldn't fall over when 1,000 people visited it. We've done the same thing by providing the same set of guide rails and example applications so customers can get up and running quickly and find value. Contrast that with a world in which companies try to hire a person who can do all this, or a manager who cobbles together a large team across various skill sets.
ZD: Do we really need data scientists? Living, breathing ones, anyway?
SP: Well, when you have the opportunity to buy LeBron James, you buy. (laughs) But not everybody has that.
They hire superstars with track records because the systems haven't been rolled out. You can't hire Accenture to do something like this. Superstars are what get it done. That's what Google does -- they have a substantial bench of people who are really, really smart. Companies that already have established businesses where a company like Google can't go after them, they can hire people who aren't necessarily LeBron James and still stay ahead of their competition.
ZD: Indeed, you just hired some. So where does that leave you? Sir, you're no Google.
SP: It's really hard as a young company to hire them. We don't expect them to be fantastic to write great code. We have a team that's good at that, and they make it easy for our data scientists to do less work. That's step one: reduce the requirements for our data scientists to write production code.
Step two is hiring people that are really creative and can visualize how to solve problems. Their backgrounds aren't necessarily computer science. One guy on our staff is a very well-known computational sociologist. You never see those things together. He's never going to be able to write production code for a Fortune 2000 company; in fact, he'll be too bored by it. So he gets to see lots of customer problems and tweak problems to make them more general.
So we paired these people who were really good at statistics and analysis with people that are experts at building distributed systems.
We struggled through the last 10 years when the tools were so bad that it took days, weeks, months to do a single iteration of a problem -- to look at what was actually happening in the data. It took seven years to become an experienced data scientist. It took that long to do the 10,000-hour estimate. You have to have experience to solve data problems. Now we've sped that up so that you can fail in seconds. So you can iterate rapidly. A better user interface was a major experience change that allows you to create better people faster.
ZD: Given your point about building experience, can someone really call themselves a data scientist today?
SP: If they work at Facebook, yes. If they work at LinkedIn, probably. If they worked at Google, maybe. There are people who are good at this. I've adopted this term because the people who adopted it early were actually the people who were using science and data to actually develop products. When you interview them, it's easy to tell -- we can screen someone and tell within a half hour whether they know what they're doing or not.
ZD: Data science is a relatively new term, but the concept isn't all that new. What changed in the last decade?
SP: I was a software engineer and program engineer at Microsoft before I went back to school. Take my cohort at Microsoft who have moved on to senior management positions -- I talk to them about what I'm doing now, and they're flabbergasted. It's completely foreign to them, because most of these things have been invented in the last five years. And these are rockstars! People I think are among the smartest people in the world. I'm not one of those people, but I'm now armed with methods that use this data better. The faster they realize that, the faster they'll be the people in their company that can actually make a difference.
Companies like mine are going to bring the Fortune 2000 into this world. We are dedicated to helping them in every way possible. That's our job -- to understand how to help them be successful with data without having their whole team retool.
You know, I have every incentive to tell you that data is going to drive everything in this industry. But I take my salary in this. My money is where my mouth is.