Dremio releases Data Lake Engines for AWS and Azure
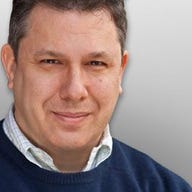

Dremio Data Lake Engine's architecture
Dremio and its eponymous platform have always been focused on high-performance data virtualization. Such platforms are centralized brokers that connect to and query multiple data sources on a user's or application's behalf. A hallmark of data virtualization, and one of its biggest hurdles, is performing federated queries that return a single result set composed of data from more than one of the connected sources. But today, Dremio is turning its attention to a single data source: the cloud data lake.
Also read: Startup Dremio emerges from stealth, launches memory-based BI query engine
Also read: Dremio 2.0 adds Data Reflections improvements, support for Looker and connectivity to Azure Data Lake Store
Also read: Dremio 3.0 adds catalog, containers, enterprise features
Jumping in the lake
Rather than obsessing on the performance of querying multiple sources, Dremio is introducing technology that optimizes access to cloud data lakes. There are good reasons for this. Cloud data lakes are seeing increasing adoption in the Enterprise, acting as the de facto collection point for corporate Big Data. But the very architectural premise that makes the cloud data lake economically efficient -- its basis in cloud object storage -- also makes for an often-unspoken downside: data access is slow.
Rather than leave that flaw an obfuscated detail, Dremio is taking it head-on and working to solve the problem. Today, the company is introducing new Data Lake Engine technology, as part of its Dremio 4.0 release, that significantly increases query performance against data in Amazon Web Services' (AWS') Simple Storage Service (S3) and Azure Data Lake Storage (ADLS) Gen2.
Also read: Microsoft releases preview of its 'Gen2' Azure Data Lake Storage service
Also read: Two new Azure analytics GA releases, one preview and a big push on SQL DW
Think globally, cache locally
In a briefing with ZDNet, Dremio CEO and co-founder Tomer Shiran explained the new architectural approach, which is fairly straightforward (with hindsight). Since Dremio itself is deployed on a cluster of cloud virtual machines (VMs) and since VM instance types that use high-speed Non-Volatile Memory Express (NVMe) solid state drive (SSD) technology are plentiful, Dremio's Data Lake Engine caches data from the cloud storage-based lake into those much faster SSDs for better performance. Dremio's name for this technology is "C3" (the Columnar Cloud Cache).
This is a smart approach based on robust precedent: various cloud data warehouse platforms, including Snowflake and Azure SQL Data Warehouse Gen2, use this very same technique of SSD caching to maximize performance while still leveraging economical cloud object storage as the persistent backing layer. In the cloud data warehouse world, this enables the clusters to be paused and resumed, while keeping bulk storage costs low.
Also read: Cloud data warehouse race heats up
Also read: Azure SQL Data Warehouse "Gen 2": Microsoft's shot across Amazon's bow
Warehouse perf, lake flexibility, cool optimizations
In the data lake world, this approach will allow the same flexibility (with even Dremio's Apache Arrow-based "reflections" able to be persisted to cloud object storage), while still upholding the basic tenet of a data lake: maintaining a single repository of data which can be queried and processed by multiple processing and execution engines.
Also read: Apache Arrow unifies in-memory Big Data systems
Dremio's Data Lake Engine technology goes beyond mere SSD caching, however. The company is also providing something it calls Predictive Pipelining, which will fetch data in advance from columnar data sources like Apache Parquet and ORC files on S3, ADLS or even HDFS (the Hadoop Distributed File System). Shiran says Predictive Pipelining results in 3x-5x faster query response times. Dremio has also brought to general availability (GA) its upgraded execution engine kernel based on the open source Gandiva technology (which it developed). Gandiva generates CPU-native native code, optimized for fast vector operations. The company says this yields performance increases of up to 70x (note that's an "x" and not a "%").
Also read: Open source "Gandiva" project wants to unblock analytics
Connectivity and security
With Dremio and C3 set up, data lakes become Business Intelligence (BI)-friendly, as Dremio provides native connectors for self-service BI platforms including Tableau, Power BI, and Looker, as well as general connectivity via ODBC, JDBC, REST and Arrow Flight. Customers will also get new advanced cloud security, based on technologies like Azure Active Directory (AAD) as well as AWS' Identity and Access Management (IAM), Secrets Manager and Key Management Service (KMS).
The combination of the data lake's agility, cloud security, C3's performance optimization and BI-friendly access means Dremio is facilitating the concept of data-driven culture in an important new way. If everything works as Dremio says, it would take the combination of open source data technology and cloud storage technology and make it performance-optimized and self-service-ready.