Google AI chief Dean sees evolution in MLPerf benchmark for machine learning
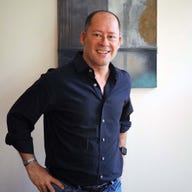
If computer systems are to evolve to handle ever larger machine learning models, a standard way to compare the effectiveness of those systems is essential, according to Google head of AI, Jeff Dean. But that system of measurement itself must evolve over time, he said.
Artificial Intelligence
"I think the MLPerf benchmark suite is actually going to be very effective," said Dean, in an interview with ZDNet, last week, referring to the consortium of commercial and academic organizations known as MLPerf, founded within the last few years. The MLPerf group have formulated test suites that measure how different systems do on various AI tasks such as the number of image "convolutions" per second.
Google, along with Nvidia and others, regularly trumpet the performance of its latest computer systems on the tests, like students comparing grades.
Dean spoke to ZDNet from the sidelines of the International Solid-State Circuits Conference in San Francisco last week, where he was the keynote speaker. Among his topics was the emergence of new kinds of chips for AI. MLPerf, he told ZDNet, can help sort out the proliferation of chips that speed up certain aspects of machine learning.
"It'll be interesting to see which ones hold up, in terms of, are they generally useful for a lot of things, or are they very specialized and accelerate one kind of thing but don't do well on others," Dean said of the various chip efforts.
MLPerf, however, has its critics. Some people in the chip industry have called MLPerf biased in favor of large companies such as Google, claiming the large tech firms engineer machine learning results to look good on the benchmarks. That raises the question of whether benchmarks like MLPerf actually capture metrics that are relevant in the real world.
Also: Google experiments with AI to design its in-house computer chips
Something of that skeptical attitude was implicit in remarks last fall by AI startup Cerebras Systems in an interview with ZDNet. Cerebras, unlike some other AI chip startups, has declined to provide MLPerf results for its "CS-1" system, saying the tests are not relevant to actual workloads.
The benchmark won't be perfect initially and will have to evolve, Dean replied, when presented with the skeptics' argument.
"I mean, any benchmark suite is going to have issues," Dean told ZDNet, "but I think having industry standard benchmark suites is going to be a pretty useful thing going forward."
Google, said Dean, along with Nvidia, and Intel, and other consortium members "are working to make these as representative as possible."
MLPerf can show things such as the speed-up achieved on machine learning tasks as a computer system is improved with new versions of hardware and software.
Some benchmark tests, he said, "are a bit smaller than some of the real world workloads we do internally, but they need to be sized so that they can be run in a reasonable amount of time on a small-scale system, even though you want them to span" very different system sizes.
It's hard, said Dean, to get a benchmark that's representative from on an embedded system operating at one watt of power, all the way up to 1,024-chip "pods."
One reason, he said, is because massively parallel systems may do well improving some operations, such as matrix math, but also increase chip-to-chip communications overhead, so that measures of performance start to become very different.
"But I think over time, you'd like to see larger-scale benchmarks than the ones that are currently in there," said Dean. "I think the MLPerf community is doing a relatively good job of doing that in a relatively fast-moving environment."
The MLPerf group started with seven tasks it deems representative of machine learning work, and says it will add tasks to the benchmark suite over time.
It's important, said Dean, to strike a balance. "You still want some stability in a set of benchmarks over time so you can compare them."
The MLPerf group specifically modeled its benchmark evaluation upon a previous generation of benchmarks for chips, known as "SPEC," for "Standard Performance Evaluation Corporation," developed in the 1980s.
Dean sees a continuation of that tradition for a new era. "I would view that as, kind of, this decade's SPECint and SPECfp," he told ZDNet, "where you can suddenly have a fairly level playing field in how everything gets evaluated, and you can have a suite of representative machine learning workloads that people care about, and that different vendors can focus effort on." The SPEC precedent is itself controversial, as people argued for years over whether the measures were accurate or, again, merely engineering-as-marketing for chip companies such as Intel.
But Dean expressed confidence MLPerf will deliver some useful qualitative insights along with quantitative ratings.
"And it's not going to be not just accelerating those benchmarks," said Dean, "but what they do to make those benchmarks perform well will be generally useful for similar kinds of processors."