IBM AI researchers say ‘what is the question’ is the real question
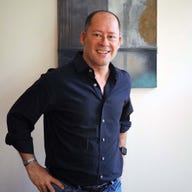
Featured
There is a place somewhere between today's machine learning technology and some future "AI" that is murky and difficult and conflicted.
Into that breach, IBM endeavors to insert itself as a voice of competency and experience.
At the prestigious NeurIPS machine learning conference in Montreal this week, IBM executives John Smith, manager of AI technology at IBM, and Kush Varshney, a principal research scientist with IBM Research, were making the case that the company has a role in how a still very "brittle" machine learning field can be more reliable and "trustworthy," depending on what one means by that phrase.
Also: There is no one role for AI or data science: this is a team effort
"It's about moving from narrow AI, where all of this really powerful technology has been highly accurate, but within a limited area of application, and making it something broader, something less brittle and something explainable," Smith told ZDNet.
Perhaps not "Artificial General Intelligence," says Smith, but something that lies between that Holy Grail of AI and today's actual implementations of neural networks.
Some of that involves particular original technical achievements, which IBM researchers will discuss this week. For example, Lazaros C. Polymenakos, is presenting the paper Knowledge Grounded End-to-End Dialog, which builds on prior work in the field of sentence embeddings in natural language. It tries to give greater understanding to machine learning models by treating individual entities in natural language statements as having their own separate space in memory.
And then there's work that broadens out from the technical approaches to a notion of how one defines the problem in machine learning to begin with. An example is Project Debater, a computer system that engages people in a back-and-forth dialogue.
"We got onto this point of how can a computer puts together arguments," says a Smith. "Debater is about how does the computer come up with these problems in the first place, how does the computer go and do all of its homework?"
"The interesting thing about Debater is, it's not just reading but listening," says Smith. "Listening to how are people saying things, it's listening comprehension."
Although Smith describes Debater as "quite early fundamental work" -- there is a raft of published research behind the effort, posted by IBM in one big collection -- it is not an example where IBM is creating technology from scratch. "We are building on natural-language processing tools here," he explains.
"If you are looking for a single, end-to-end model, it's not that, it's the whole NLP pipeline, and making all of that work in this situation, and establishing a baseline."
To Smith, again and again the matter of AI comes back to defining a problem. "You can come up with a great idea" from a technology standpoint, "but then have no data, or not have anything to do with the data that's interesting."
IBM has selectively picked areas where it believes it can help, such as cases of bias, for example. At IBM's booth at the show, the company displayed monitors describing efforts such as a an exploration of predictions of recidivism among felons in the US.
The research firm ProPublica had done a study, in 2016, of predictions of recidivism that was performed using a private algorithm, "COMPAS," sold to law enforcement by the firm called Northpointe. ProPublica's study had found that African Americans, for example, were predicted by the algorithm to have higher rates of recidivism than other members of the population, which was not actually supported by historical recidivism data -- an instance of race bias.
Varshney gestured to results on the display that showed IBM could revise such data to come up with predictions that were highly accurate, but also didn't have the high bias. Why this is hard, and why it benefits from IBM's technology, he says. "The difficulty is the dependency between the statistical elements" says Varshney. "You have to go through and find all the ways that there are dependencies that operate that cause bias even if you've explicitly removed these variables of race, gender, etc., or explicitly compensated for them." (More on the effort in the original blog post on the matter.)
Beyond such isolated incidences, Varshney says an in increasing issue he and others will tackle is the overall question of what makes statistics reliable. Machine learning is one application of statistics, and whether it is used narrowly or broadly, there is still the fundamental question of how well statistics can be relied on to make inferences about populations and behavior and future occurrences. Another example of putting things into practice is work conducted with Memorial Sloan Kettering Cancer Cancer on the "International Skin Imaging Collaboration melanoma project. (That work is separate from IBM Watson work being done with Memorial Sloan Kettering Cancer Center.)
Must read
- IBM creates AI to destroy humans... in debate (CNET)
- How IBM Watson is revolutionizing 10 industries (TechRepublic)
Although the work is purely academic at this point, Varshney is optimistic, saying that "hopefully we will get it into the clinic soon." The idea of an AI-assisted doctor is a decades-old dream, he is aware, but "first we need to get the accuracy that's required - it comes back to trusting AI."
Such examples of partnership comes back to Smith's larger point of defining the problem.
"It's a conversation we have all the time with subject matter experts, when we talk to one another that's when ideas emerge."
He notes that with IBM having over 400,000 employees "in every industry ... we get a lot of real world problems coming back to us."
36 of the best movies about AI, ranked
Previous and related coverage:
What is AI? Everything you need to know
An executive guide to artificial intelligence, from machine learning and general AI to neural networks.
What is deep learning? Everything you need to know
The lowdown on deep learning: from how it relates to the wider field of machine learning through to how to get started with it.
What is machine learning? Everything you need to know
This guide explains what machine learning is, how it is related to artificial intelligence, how it works and why it matters.
What is cloud computing? Everything you need to know about
An introduction to cloud computing right from the basics up to IaaS and PaaS, hybrid, public, and private cloud.