Integrated vs. specialized: Which shines the brighter light on 'dark data?'
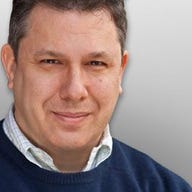
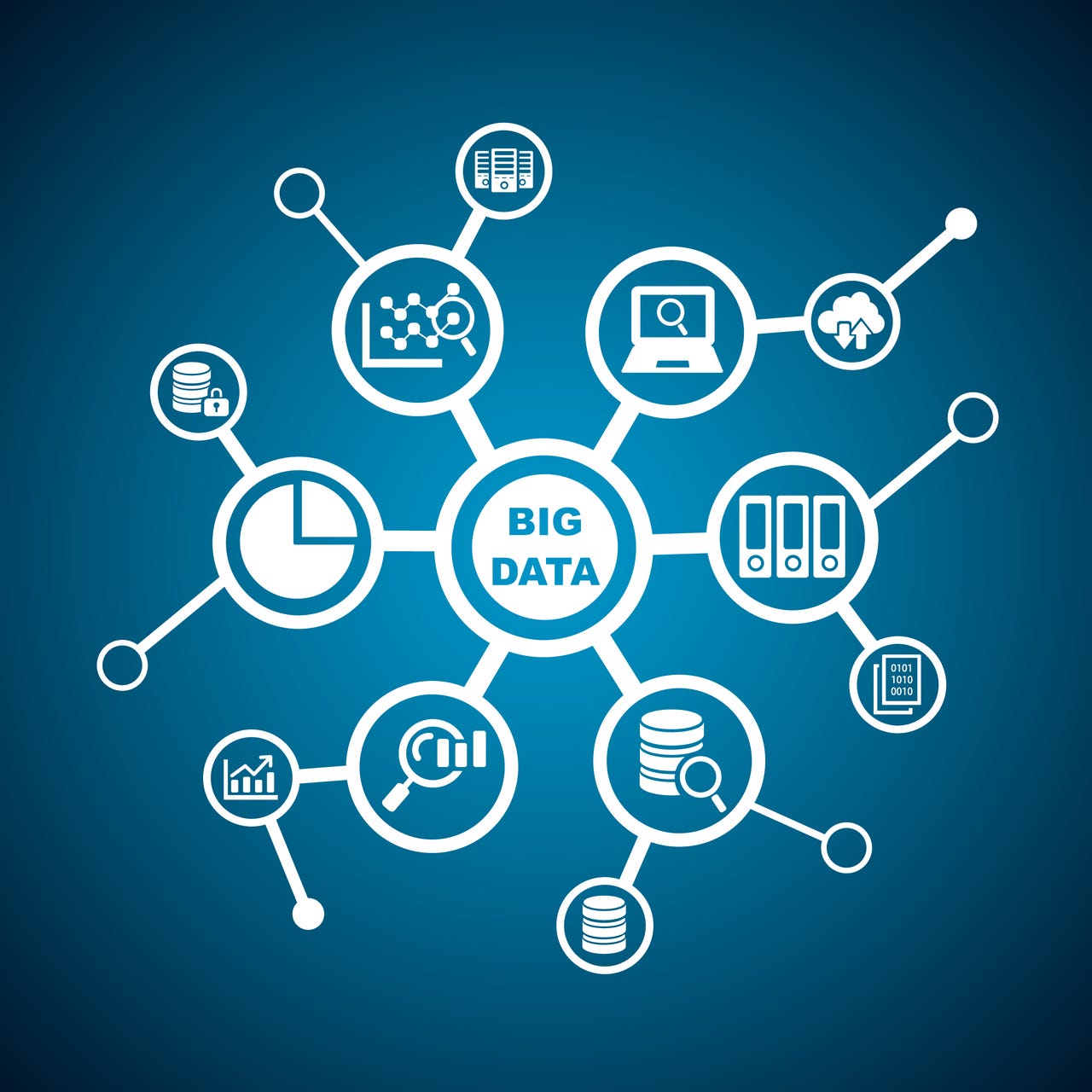
Ingest, data prep, analysis, visualization, and export -- they're all part of the big data analytics lifecycle. The good news? The market sports multiple product categories that handle each of these.
The bad news: there are far fewer products that handle many of these areas together. It's getting more complex too, as other big data lifecycle categories, like data lake management/data cataloging and big data operations/DevOps are emerging.
This may be frustrating, but it's also understandable: if there's an area of functionality that the market is neglecting, then funding a company to develop and provide that functionality makes sense. It helps the market, it helps the company and, hopefully, it helps the company's investors.
Does it help the customer, though? That's harder to say. If you're doing your data prep in one tool and your analysis in another, that's a bit regimented, and the context switch may be jarring. Moreover, if your analysis reveals to you more prep work to be done, you are sent back to the data prep tool for a "do-over," rather than embarking on iterative effort.
Case in point
Featured
Recently, I spoke to Bob Laurent, VP of product marketing at Alteryx on a related subject. The discussion began as an investigation into Laurent's notion of "dark data:" data that you have in your possession but aren't using, or analyzing, for the betterment of the business.
As Alteryx has a focus on data preparation, the discussion began with talk of how to avoid dark data, by grabbing, processing and coalescing data sets, be they buried in log files, point-of-sale feeds or elsewhere, to get at intelligence that would otherwise be latent and obscured.
That's all fair game. The nitty-gritty of digging for valuable data -- then scraping the debris away and making it sparkle and shine for observation and analysis -- is the bread and butter of every company in the analytics field.
It wasn't really enough though, not for an interesting conversation -- until something unexpected happened.
Lifting the darkness
But then, as if sifting for data sets, we stumbled on something really valuable. Laurent described a case study to me -- one where a financial services customer used Alteryx to look at its trading terminal data, to find correlations between certain trading patterns and various abuse incidents (like money laundering, for example). While discussing the use case, Laurent mentioned something that at first seemed incidental, but then revealed itself to be key.
After staff at Alteryx's customer completed their data prep and analysis work, to determine patterns in transactional data that may indicate money laundering activity, the customer decided to take that analyzed data and make a predictive model from it, allowing the customer to detect potentially nefarious activity in advance of any illicit events occurring.
Cluster analysis in Alteryx
Repurposing
The customer acquired Alteryx for the purposes of observational analytics. That work is valuable in itself. It was all the customer intended to carry out and the project was already successful. But then, because Alteryx has integrated the R programming language into its product and has the ability to build predictive models and score data against them, the customer put those features to work too.
The availability of adjacent capabilities, in a single product, led to a customer realizing much greater value. No salesperson had to intervene and pitch the potential to do this; no additional products were required. But the functionality was there, and the customer's work to date meant all of the necessary prerequisites to build a predictive analytics solution were met. So the customer ventured out into predictive analytics of its own accord, at its own pace, connecting the dots on its own.
From capabilities, a result
Obviously, the customer got more value. Arguably, so did society, as this analytics solution effectively fights financial crime. It's a moral victory, and it's a story with a moral: enabling a customer to make connections in the data lifecycle enables her to understand and "own" the analytics process.
Laurent mentioned to me that Alteryx is seeing a shift from customers taking known data sources and determining desired outputs, to exploring potential data sources and discovering if useful, unexpected outputs can be derived. Clearly, the case of this customer provides supporting evidence here.
Products that cover multiple phases of the data lifecycle effect intimacy with data and enable tenacity in non-specialists to dig into their data, to derive levels of insight they never knew were there.
It strikes me that this illuminates the crux of the "dark data" question. Yes, point solutions shine a light on individual pieces. But more integrated solutions help solve the whole puzzle. And that's ultimately how customers get their ROI.