Intel-backed startup Nyansa chases the total problem in the AI of network monitoring
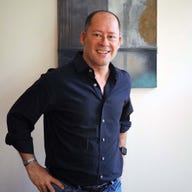
Featured
There are many opinions about what matters most in machine learning. Some would say it's the data, some would say it is the algorithms and equations used to train computers on that data. Still, others would say it is the formulation of the question itself that is most important in machine learning.
The last point of view is representative of a startup called Nyansa, composed of networking veterans and big data specialists who believe they have a better approach to network management than, say, Cisco Systems or Hewlett-Packard Enterprise.
The reason, according to chief technologist and co-founder Anand Srinivas, is because Nyansa figures out all the different parts of a system -- not just the switches and wireless access points, but the applications as well, that can affect what an end user experiences.
"Our innovation is not inventing new machine learning algorithms, it is in terms of bringing machine-learning algorithms to a use case like networking," Srinivas said in an interview with ZDNet on Monday. Srinivas holds a PhD in wireless networks and algorithms from MIT, and has held a number of industry positions, especially for software-defined networking, at firms such as Overture Networks, Plexxi, and Airvana.
Also: Network technologies are changing faster than we can manage them
Based in Palo Alto, four-and-half-year-old Nyansa sells tools to IT to monitor the health of the network, explain degradations of performance when they happen, and then propose solutions. Its tools run on Amazon's AWS, though they can also be installed on-premise, with hooks back to the public cloud. The company has over 100 customers, representing over 10 million devices "under observation," it says, across 200,000 access points from different vendors, on hundreds of production networks. Clients include Uber, Tesla, and Lululemon. It has been bankrolled by chip giant Intel's investment arm, and Formation8, to the tune of $27 million.
Its machine learning tools are very simple, far less sophisticated or adventuresome than today's deep learning neural network approaches. They include things such as logistic regression analysis, random forest searches, nearest-neighbor searches, and "cluster" analysis. "A lot of this is off-the-shelf stuff," confesses Srinivas.
"It is more straightforward than deep learning; deep learning is not the right approach for us, not yet, not until we grab all the low-hanging fruit which the simpler kinds of machine learning algorithms can give us."
Nyansa gathers petabytes of data from those millions of client devices and thousands of access points, and first establishes a baseline. How well does the network perform, in terms of things such as what percentage of users have a wireless connection issue, on average, or what percentage have a Citrix application connectivity issue? Some of these devices have no users, they are Internet-of-Things gadgets, such as a General Electric wireless patient monitor, or the wrenches used by Tesla on the shop floor in its Fremont facility. Telemetry data must be gathered from those devices as a baseline for performance.
"One way you can think of us is as a vertically-integrated Splunk," says Srinivas, referring to the Big Data monitoring system that ingests and mines customer data. "We can take any type of data and tie it into our system, but we go one step further to solve customer use cases."
Also: Cisco launches UCS system for AI, machine learning, deep learning
By having data from multiple customers in the cloud, says Srinivas, a baseline can be set not just for a given customer but across an industry. "What's good performance," he asks rhetorically. For a given industry, "if the baseline is a 30 percent network performance connection failure, then 5 percent for a given customer may be fine for them."
Once a baseline is established, deviations can be detected in order to determine if a problem is, say, a network problem per se, or rather an application problem. And once deviations from the baseline are established, predictions can be made. "Based on what other customers have done, what is our prediction for actions that have been most beneficial," is how he describes it. By then observing how recommendations are carried out, and the effects, the system can move beyond mere correlation, the focus of a lot of machine learning, to a sense of causality.
"By automatically learning a baseline, and learning it everywhere, and doing it in the exact same way, we can give you a recommendation, and it will have an impact."
"At first, perhaps it's 80 percent correlation. But when a customer takes that action, that baseline will tell you the truth of whether the action made a difference; if it [performance] gets better, the recommendation by definition is correct. That feedback loop gets you back into causation."
Also: Why you need to learn about application performance monitoring TechRepublic
As to how they differ from Cisco or Hewlett, Srinivas sees the Nyansa system as more comprehensive in what it looks at than either one. "We don't care who the vendor is for wireless [access points] or RADIUS or DNS or DHCP, we will take whatever data from whatever source, that is a fundamental difference."
"Their data sources are limited to their own stuff."
Srinivas offers the example of GE bedside wireless patient monitors in a hospital run by client Mission Health, a healthcare system serving North Carolina. It is not enough to say that a network is or is not performing at baseline. "The final thing that matters is, are those waveforms [of patient data from the monitors] getting back to the nurse, and is that nurse able to see the oxygen level on the screen. It doesn't matter if the wireless signal is good, you can't just baseline wireless, because the particular data source you care about, the monitor, has nothing to do with switches."
Also: Facebook is using AI to curb exploitative and naked images of kids CNET
The answer, then, to machine learning, in its simpler form and perhaps even its more complex incarnations, is how engaging with the complexity of situations builds understanding. "Even with deep learning, the magic is in tuning the deep learning network, knowing how to turn the knobs," observes Srinivas. "The crux of it is experience, over time knowing exactly how to tune things."
Scary smart tech: 9 real times AI has given us the creeps
Previous and related coverage:
What is AI? Everything you need to know
An executive guide to artificial intelligence, from machine learning and general AI to neural networks.
What is deep learning? Everything you need to know
The lowdown on deep learning: from how it relates to the wider field of machine learning through to how to get started with it.
What is machine learning? Everything you need to know
This guide explains what machine learning is, how it is related to artificial intelligence, how it works and why it matters.
What is cloud computing? Everything you need to know about
An introduction to cloud computing right from the basics up to IaaS and PaaS, hybrid, public, and private cloud.
Related stories:
- There is no one role for AI or data science: this is a team effort
- Startup Kindred brings sliver of hope for AI in robotics
- AI: The view from the Chief Data Science Office
- Salesforce intros Einstein Voice, an AI voice assistant for enterprises
- It's not the jobs AI is destroying that bother me, it's the ones that are growing
- How Facebook scales AI
- Why AI and machine learning are driving data lakes to data hubs
- Nvidia, Scripps Research partner to advance AI for disease prediction
- This is what AI looks like (as sketched by AI)
- AI delivering returns for enterprise early adopters
- Google ponders the shortcomings of machine learning