Machine learning vs payment fraud: Transparency and humans in the loop to minimize customer insults
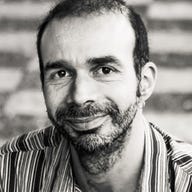
False positives, or customer insults? Which one sounds better? It's a trick question -- it's the same thing, framed differently. In binary classification, a false positive is an error in data reporting in which a test result improperly indicates the presence of a condition (the result is positive), when in reality it is not present.
Even in our data-driven era, however, that definition could sound a bit dry or confusing for some. Hence, "customer insult" was born. In fraud prevention, the term customer insult is used to describe a false positive: A transaction, which is not fraudulent but is nevertheless marked as suspicious.
That happens often and is more of a problem than you may think. A recent survey conducted by Sift on 1,000 consumers in the US found that 36% of respondents have had a transaction falsely declined due to suspected fraud. Another study by PwC found that even one negative customer experience can have a significant impact on retailers.
So, what can be done?
Anti-fraud largely depends on machine learning
Kevin Lee, Trust & Safety Architect at Sift, was leading the Risk Ops team at Square back in 2013. During his time there, they were focused on loss prevention but equally, if not more so, on user growth and adoption. Lee did not definitively say Square invented the term customer insult. But he did mention they needed a way to measure false positives and wanted to describe it more viscerally.
As such, they started using the term "insult." The term stuck and has since become part of the nomenclature for fraud, risk, and trust and safety teams. Today, Sift announced the launch of Insult Monitor, a capability for online businesses looking to increase revenue by reducing false positives. ZDNet connected with Lee to discuss how this works and what it brings to the table.
Sift's Insult Monitor promises to maximize revenue for online businesses by measuring fraud false-positive rates and allowing those businesses to reduce friction for legitimate purchases. Insult Monitor is integrated with Sift's Payment Protection product. Customers can set up Insult Monitor within their Sift Console and set up groups to begin testing for customer insult rates.
Customer experience is important when deciding between buying options. Anti-fraud is part of this, too. Image: PwC
After testing, Fraud or Trust and Safety teams can adjust thresholds to allow more legitimate orders through (reducing insult rates) while still blocking fraud. The big question is how does this work. To answer that, we'll have to revisit the fundamentals of fraud prevention in general, and Sift's solution more specifically. Both largely depend on machine learning.
Lee stated that Sift uses supervised machine learning because it ramps up faster than unsupervised -- it does not take as much data to learn. Sift leverages its global model, as it is used by over 34,000 websites.
Sift, in turn, uses those websites to protect each other, said Lee:
"We have real-time adaptability and anomaly detection, which means that we can stop new forms of bad as soon as it pops up. We don't need to wait for a model refresh or a rule to be generated. This reduces a business' overall company exposure rate to fraud.
We practice dynamic friction, meaning, machine learning enables us/merchants to provide amazing user experiences to known, trusted customers. This enables merchants to complete the bigger / Amazon-esque transactions with more tools under their belt. We level the playing field a bit."
The holy grail for effective anti-fraud: transparency and humans in the loop
As far as false positives go, however, a big part of the problem seems to be lack of visibility. According to CNP's 2018 Fraud Operations Study, 42% of businesses don't even know their false-positive rates. This is a very high percentage, considering the impact false positives can have. Is it that businesses don't know any better, or do they lack the means to check?
To date, said Lee, businesses haven't been able to measure their false positive rates because the only way they would know if a transaction was wrongfully declined was if the customer reached out to them to let them know. Those who say they know their false positive rates are likely basing that on customer complaints or estimations.
This, Lee went on to add, is one of the ways Insult Monitor is different: It provides a concrete, quantitative way to measure false positives. Sift built out a sampling framework that allows merchants to select a subset of orders that would normally be declined. Businesses can tag them appropriately, and let it go or send it to some additional verification to see which are truly fraudulent versus those that could be legitimate.
Leveraging human expertise alongside AI is a way to develop and use AI.
Sift claims businesses can set up Insult Monitor instantly without needing any developer resources. This capability isn't reliant on machine learning. Rather it's an experimentation framework built into Sift's workflows. Sift built Insult Monitor, Lee went on to add, because of a pain point felt by many customers who asked if there was a way to figure out false-positive rates.
This, Lee said, is in many ways the 'holy grail' for measuring the effectiveness of fraud-fighting teams, who until now have only been able to track how much they're mitigating risk rather than how much they're growing the business.
We don't know how well this will play out, but the approach looks like it's building on best practices: Introducing transparency so that users know what is going on in the system, and control, so that they can intervene. Human-in-the-loop approaches for machine learning are gaining ground.
Our takeaway: The more sophisticated organizations become at using machine learning, the more clear limitations and mitigation techniques become.
NOTE: Article updated on March 12, 2020 to reflect the number of web sites using Sift.