MapR 6.0 converges control of data at rest and in motion on the same pane of glass
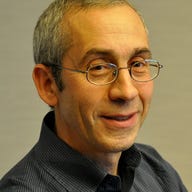
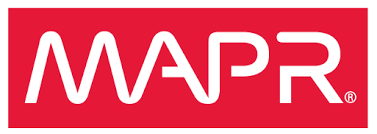
As the processing of data in motion claims a larger proportion of big data workloads, the brass ring has been to make it a first class citizen with data at rest. The new version of the MapR converged platform does just that from a control perspective: data in motion and data at rest can now be managed from the same pane of glass. Call it convergence of control on a data platform that MapR bills as converged.
The new version, MapR Converged Platform 6.0 being announced today, also consolidates several security-related functions and introduces a new change-data-capture (CDC) capability. In conjunction with MapR's recent announcement of its Data Science Refinery, the theme of the 6.0 release is being cast as promoting DataOps.
The backdrop is that MapR positions its platform as more than just Hadoop. While other Hadoop providers will support managing data at rest and data in motion, MapR uniquely handles it within a single cluster -- that is core to its billing as a converged platform. And its announcement earlier this year to host containers and abstract its storage breaks new ground as a big data application platform, while opening up a new front in the file storage market.
The highlight of the 6.0 release is an expanded and more granular MapR Control System. Like its rivals, MapR offers a pane of glass for configuring, monitoring, and managing their clusters. In the new release, the control system becomes more granular, drilling down below file system to volume, table, and stream levels. That's where you get the elusive ability to manage data in motion and at rest on the same screen.
A related feature is streamlining and extending the coverage of some security-related functions, which can be activated with a single click. The new release adds single click activation of encryption on the wire (for data in motion) and enforcement of authentication. But the release stops short when it comes to encrypting data at rest; for that you still need to drill down into some Linux file system utilities. We view this as MapR's next logical step if you are going to converge securing of data and access to it.
A common pain point for managing Hadoop clusters (we'll use the H word even if MapR won't) is that security measures don't always cover all components. In the 6.0 release, MapR is supporting one-click authentication and encryption on the wire to the components that are clearly strategic to it: the core file system (MapR-FS), the NoSQL database (MapR-DB), data warehousing (Hive), data flow (MapR Streams), interactive query (Apache Drill), and compute (Spark). Given MapR's focus on its control system, Drill, and Streams, it's not surprising that the new capabilities won't extend to components that are not strategic to the platform: Sentry, Storm, and Impala.
Other new and recently announced features that are part of the 6.0 release include a new change-data-capture (CDC) capability that provides support for a function that is core to the enterprise database world. In this case, MapR is billing it as a key component of real-time data ingest that can be used for training and refreshing machine learning models that, using MapR's container support, can be executed as microservices. It capitalizes on a recent secondary indexing feature added to MapR-DB that can be utilized for representing unique cases, such as pertaining to specific analytic models or materialized views.
MapR is putting a DataOps spin on the new release because of the affinity of more granular control and CDC features that can be leveraged by its recently announced Data Science Refinery. The refinery is designed to promote self-service data science. This harks back to the guiding notion of data science: to succeed you have to fail fast, and you can only fail fast if you have complete visibility and control over real-time processes. MapR is not the only big data platform provider that is providing tools for enabling data scientists to put their models into production, but their converged architecture provides a one-stop shop for those looking to fail fast.