MIT’s deep learning found an antibiotic for a germ nothing else could kill
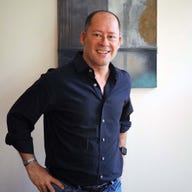
One hundred years ago, the state of the art in finding antibiotics was epitomized by the playful explorations of Alexander Fleming, the Scotsman who discovered penicillin.
"I play with microbes," Fleming is quoted as having said. "It is very pleasant to break the rules and to be able to find something nobody had thought of."
Today's research in antibiotics is conducted somewhat more mechanically, perhaps, but it's still important to break the rules sometimes, to look where one might not otherwise.
Scientists at the Massachusetts Institute of Technology and Harvard last month described in the scholarly journal Cell how they used a deep learning neural network to identify a molecular compound that's different from most antibiotics. They showed that when the compound is injected in mice, it fights bacteria that no existing drug can eliminate.
The discovery even has implications for fighting the coronavirus that is causing the Covid-19 disease.
It's a rule-breaker on many levels: Finding a novel use for an existing compound; using neural nets in place of familiar chemical definitions; and finding an antibiotic that doesn't behave like the usual kind. It's enough to make one believe deep learning forms of AI can change the rules of life sciences.
The single antibiotic molecule they arrived at, christened "halicin," in honor of the Hal 9000 computer in the movie 2001, is a compound known for years to inhibit the activity of protein kinases that can cause liver damage. It wasn't known that it could serve as an antibiotic, until now. In that way, halicin is characteristic of a recent trend in drug discovery: repurposing, where a known compound finds new uses.
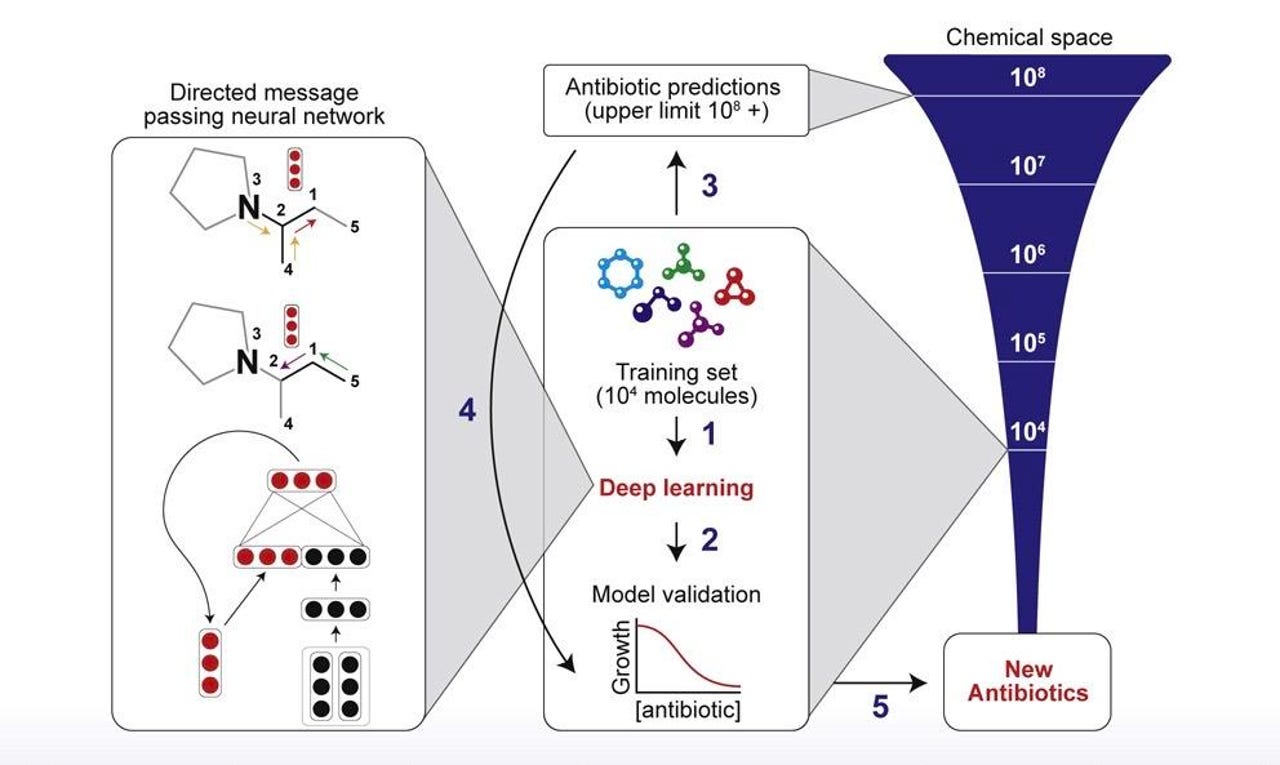
The training procedure used by MIT scientists first exposed a neural network to over two thousand example molecules and "ground truth" about whether or not they fight E. coli bacteria. The trainer network could then be used to find bacteria-fighting molecules in larger data sets including those with millions of molecules.
What halicin fights is a version of the pathogen known as Acinetobacter baumannii, or A. baumannii, one of the increasing number of "multi-drug resistant" bacteria that can't be fought by existing antibiotics. A. baumannii tends to occur in hospital settings, and can accumulate on all sorts of surfaces, including pillows and bed linen but also blood pressure cuffs. It often attacks critically ill patients and it's been a rising public health problem for years. The MIT authors note that the World Health Organization has marked A. baumannii as "one of the highest priority pathogens against which new antibiotics are urgently required."
The big question, as with most AI-in-medicine efforts, is whether the scientists simply got lucky or if there's a logic to the discovery that points the way to further breakthroughs.
There's much here to suggest the latter, logic rather than luck. The scientists trained a model to form a representation of the chemical structure of molecules, and it was that model that picked out the compound they found, a compound that would ordinarily seem unlikely.
Also: Google DeepMind's effort on COVID-19 coronavirus rests on the shoulders of giants
To understand the logic at work, you have to consider the problem the scientists faced, a problem a lot of AI grapples with: exploration versus exploitation, how to expand the search for possible answers, but also build upon what's already known.
Much of antibiotics research at the moment is experiencing a kind of crisis of exploitation and exploration, according to lead author Jonathan Stokes, who is a Banting Fellow in the Collins Lab at the Broad Institute of MIT & Harvard. Antibiotic research has in recent years either turned up duplicative molecules that don't go beyond existing antibiotics, or else gotten stymied trying to find possibilities in the vast searchable chemical space created by high-throughput screening.
The solution is to leverage AI. Stokes and the team — it's a big team, as Stokes is joined in the paper by nineteen colleagues from multiple MIT and Harvard labs — trained a neural net on known molecules that do and don't fight the bacteria Escherichia coli. Once the network was trained to classify whether a molecule could fight E. coli, they used that trained network to search a database of over 6,000 molecules that are in various stages of clinical development, to pick out one that would match what the neural net concluded is a structure that can fight E. coli, and there they found halicin.
Lo and behold, that same E. coli antibiotic, halicin, is also good at fighting other bacteria, in particular A. baumannii, the presence of which it drastically reduced in mice who'd been infected with the bacteria. "The isolate that we used in our skin infection model (A. baumannii CDC 288) was resistant to all antibiotics commonly used to treat this bacterium," Stokes wrote in an email to ZDNet.
(One of the stand-out virtues of this work is that it's not just searching in silico, but also testing in vivo; that kind of thorough "wet lab" work is rare in deep learning research, as Science Magazine's Derek Lowe has pointed out.).
Not only was halicin an unexpected antibiotic, it seems, as far as they can tell, to operate via an "unconventional mechanism" that they had to study to try and understand.
What's key here, as far as the neural net, is that the deep network didn't rely on preset information about the chemical structure of molecules, it built new representations, as they're called.
For years, science has assembled "fingerprints" of molecules, including antibiotics. The fingerprints are things such as the sequence of bonds between atoms in a molecule. Those fingerprints can be used to predict the activity of the molecules, such as whether it might be likely to fight bacteria. The process of using computers to search such fingerprints is a well-established discipline called "Quantitative Structure-Activity/Property Relationship," or QSAR. Deep learning has already made progress using fingerprints to predict molecular properties.
But relying on known fingerprints wouldn't work if the problem is to explore more broadly. And so the deep network used in the MIT study, called "Chemprop," instead built those fingerprints from scratch.
The Chemprop network takes the visible chemical bonds in the molecule, left, and uses them to infer missing bonds, constructed as a vector in the layers of the neural net, right, a process of inference known as "message passing."
Chemprop, developed by colleagues including Kevin Yang at MIT, was explained last year in a paper by Yang and team members. Chemprop is what's known as a "directed-message passing neural network," or D-MPNN. The message-passing phenomenon is now well-established in the deep learning literature as a way to handle structured data, including the graph of a molecule as atoms and bonds between them. Chemprop learns to predict the structure of molecules by having to fill in those chemical bonds in the training data. It's a process familiar to anyone who's looked at work on graph convolutional neural networks and the like.
As Stokes and the team describe it, the D-MPNN "builds a molecular representation by iteratively aggregating the features of individual atoms and bonds." As successive layers of the deep network transform this representation, what is built up at the "highest-level" of the network is a "single continuous vector representing the entire molecule." That single vector is the learned alternative to what has been the engineered fingerprint of QSAR.
Of course, the deep network's results are helped by a subtle balancing of considerations by Stokes and the team. The molecular database in which halicin was found, called the Drug Repurposing Hub, which is housed at Broad, was chosen because it "consists entirely of molecules under clinical or preclinical investigation," Dr. Stokes told ZDNet in email. That's important for the purpose of exploration mentioned above, because the team was "looking for new molecules" rather than drugs that are already approved and on the market.
However, they also did a separate experiment where they fed Chemprop with the contents of ZINC15, a well-known in silico chemical library "that contains both clinical and non-clinical molecules," most of them non-clinical. In that search, starting from 1.5 billion molecules, they narrowed the field to just over 107 million molecules that had "physicochemical properties that are observed in antibiotic-like compounds." From that still-vast subset, Chemprop found eight "antibacterial compounds that are structurally distant from known antibiotics," including one they recommend for further research because of the fact it completely sterilized E. coli when tested in vitro.
One in a billion: the halicin molecule MIT found, sounded by molecules of the training set and from the larger search space of the Drug Repurposing Hub, visualized as a similarity relationship known as "t-Distributed stochastic neighbor embedding," or t-SNE.
The team is working on increasing the size and diversity of its chemical libraries for training, Stokes told ZDNet.
"Within the next 18-24 months, we are hoping to assemble a purpose-built training library that can be applied to a wide array of bacterial pathogens in the search for new antibiotics," he wrote in email.
It's possible Chemprop could be applied to coronavirus. "Indeed, the platform could be extended to other applications, including the development of antiviral molecules, provided the appropriate training data are available," Stokes told ZDNet.
And so, the MIT work is proceeding through a careful set of choices about exploring and exploiting knowledge, based on design of the network and knowledge of the data sets. It's not mere statistical good fortune.
In fact, the authors compared Chemprop to older machine learning methods such as random forest and support vector machines using traditional engineered QSAR fingerprints instead of learned representations. Those statistical approaches found things, but they didn't give the same prominence to halicin, perhaps because the statistical models are not inferring broad structure, they are simply pattern-matching to known fingerprints.
Also: The subtle art of really big data: Recursion Pharma maps the body
That last point raises the single most tantalizing element in all this: representation. Chemprop found something meaningful, and given that halicin is structurally different from other antibiotics, it seems that Chemprop is generalizing away from narrow structure to broader patterns, which is what deep learning is supposed to do.
But the high-level vector that Chemprop creates is a black box. We don't know exactly what's in that learned representation. In an email to ZDNet, Dr. Regina Barzilay, one of the authors, who has multiple appointments, including with MIT's Computer Science and Artificial Intelligence Lab, confirmed that the learned vector is not interpretable, unlike the traditional engineered fingerprints. The vector is a compressed image of all the patterns of molecular structure the machine encounters, and that compression renders the vector both effective and, in a sense, opaque.
One interesting future challenge for this kind of work may be to excavate the contents of the high-level vectors that Chemprop creates. If one looks at the comments in response to the original Cell paper, mostly from biologists and chemists, there is skepticism because the learned representation doesn't seem to correspond to the vast store of biological scholarship about how antibiotics work. Addressing such skepticism by opening the black box could be a worthwhile project for further research. The learned representation may ultimately point to higher biological or chemical truths that humans haven't yet acquired that could be profound.
In the meantime, Chemprop, and the discovery of halicin, both reflect the intelligent priorities of the scientists, namely to build upon acquired biological knowledge and at the same time vastly expand the boundaries of exploration — as Fleming did, to look where one might not otherwise.