Watch these autonomous drones zip through the woods
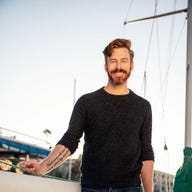

Expert human drone pilots have proven incredibly adept at piloting UAV through complex courses at high speeds which are still unmatched by autonomous systems. But researchers at the University of Zurich and Intel Labs are collaborating to change that, and their work, recently presented in the journal Science Robotics, could have far-reaching implications for the future of commercial drones.
"Autonomous navigation in environments where conditions are constantly changing is restricted to very low speeds," explains Matthias Müller, Lead of Embodied AI Lab at Intel Labs. "This makes drones unable to operate efficiently in real-world situations where something unexpected may block their path and time matters."
That's obviously a big impediment to safely rolling out drones for commercial use. The solution seems to be harnessing the decision-making abilities of expert pilots to train drones to function autonomously.
"In partnership with the University of Zurich, we were able to show how a drone trained exclusively in simulation by imitating an expert pilot is able to perform in challenging real-world scenarios and environments that weren't used during the training of the convolutional network," says Müller. "The trained autonomous drone was able to fly through previously unseen environments, such as forests, buildings and trains, keeping speeds up to 40 km/h, without crashing into trees, walls or any other obstacles – all while relying only on its onboard cameras and computation."
The results were achieved by having the drone's neural network learn from a simulated expert pilot that flew a virtual drone through a simulated environment full of complex obstacles. The expert had access to the full 3D environment while the drone's neural network only had access to the camera observations with realistic sensor noise and imperfect state estimation.
That input imbalance (what researchers call a "privileged expert") forced the drone to learn to act with exceptional dexterity in less than ideal conditions. The quadrotor demonstrated a decrease in the latency between perception and action while simultaneously demonstrating resiliency in the face of perception artifacts, such as motion blur, missing data, and sensor noise.
"Existing systems use sensor data to create a map of the environment and then plan trajectories within the map – these steps require time and errors compound, making it impossible for the drones to fly at high speeds," says Müller. "Unlike current systems, future drones could learn navigation end-to-end in a simulated environment before going out into the real-world. This research shows significant promise in deploying these new systems in a wide array of scenarios including disaster relief, construction sites, search and rescue, agriculture and logistics, and more."
One of the benefits of this system is its applicability to a wide variety of real-world environments. The approach demonstrated in the research involved experiments that tested in a set of human-made environments (e.g. simulated disaster zone and urban city streets) and also diverse natural environments (forests of different types and densities and steep snowy mountain terrains). Future application areas for the technology could be disaster relief scenarios, construction sites, search and rescue, agriculture and logistics, and delivery.