AI must tackle the sparsity challenge, says Landing AI’s Gopi Prashanth
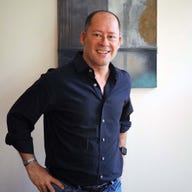
Featured
"Sparsity, that's the direction where deep learning should expand," says Gopi Prashanth, who is vice president of engineering at AI-startup Landing AI, run by former Google AI luminary Andrew Ng.
In an interview with ZDNet, Prashanth reflected on the challenge of taking something built for really big data, the machine learning approach called deep learning, and re-engineering it for very little data, perhaps just one single sample at a time.
It is not an academic concern. The mandate of Ng and his team is to put AI to work for business. That requires using techniques such as machine learning in some settings where there my be very few good examples of a problem to use to train the machine.
Consider a manufacturing line for autos or another finished good. They are systems built for reliability, and so there are not numerous examples of failure from which to learn.
Also: Andrew Ng sees an eternal springtime for AI
Gopi Prashanth, VP of engineering with LandingAI.
"Say you are a visual quality inspector, and a part comes to you" on the manufacturing line, offers Prashanth. "You make a decision by rolling that part in your hand and looking at it to determine whether it is acceptable or not."
"Maybe one in 1,000 products are faulty, two at most; humans can take the two examples and generalize from them very well. But to teach a machine to use a few samples of data is a very hard technical problem to solve -- it's one of the key challenges we have to work on."
Prashanth knows something about systems that interact in the real world. At Amazon, he worked on the "Amazon Go" project that built stores where people could just walk in and grab stuff and go, and have the total billed to them later, using a novel combination of sensors and machine vision engineering.
When Ng reached out to him, while he was at Amazon, "I wasn't looking to leave, but it was a very personal approach," he says. "He [Ng] talked less about the opportunity, and more about me; he had spent time looking at my career and my resume to understand my strengths and weaknesses; he made it very personal, and that really struck a cord with me."
"We talked and and I found we shared a very similar vision."
Also: IBM, Apple, and Facebook represent new tilt toward business for venerable AI conference
The vision is one of solving "many problems for business, thinking ten to fifteen years ahead," says Prashanth. Applied science is the focus, how to transform a whole company or a whole field, such as manufacturing or healthcare.
"We are going into areas that are not traditionally tech-heavy, like manufacturing," he says. "Our assumption is we will uncover problems we can solve that even the customer isn't aware of, things about optimization and cost savings and all that -- that is our assumption going forward." (Read the ZDNet interview with Ng in December.)
The problem of sparsity goes to the heart of where deep learning and other AI approaches break down, Prashanth suggests.
"Deep learning is very nascent," he says. "It is very good at taking in large volumes of data, basically fitting that to a multidimensional surface in hyperspace -- a manifold," he says, referring to the concept of a non-Euclidean geometry that represents connections between data points.
Also: Can IBM possibly tame AI for enterprises?
"Deep learning is going through more and more of this pile of junk, as they call it. The deep learning network puts stuff in, and the model comes out," is how he summarizes the basic operations of deep learning. "If the model is wrong, you collect more data, you stir the pile again, and you train the network out of that error."
"But, not many people are looking into the problem of generalizing from sparse data."
Must read
- 'AI is very, very stupid,' says Google's AI leader (CNET)
- How to get all of Google Assistant's new voices right now (CNET)
- Unified Google AI division a clear signal of AI's future (TechRepublic)
- Top 5: Things to know about AI (TechRepublic)
In manufacturing, the assumption has always been to remove "anomalies" from the system, hence the very few examples in quality control of something that is wrong.
Landing AI doesn't necessarily need to invent new deep learning frameworks or tools. Things such as TensorFlow, which are available to Prashanth and the team, "are already pretty elaborate."
"Right now, we haven't hit a point where we need to reinvent the tools."
What's required, he says, "is to teach a network to generalize" based on examining "what features do humans perceive, and how do you train a model to do the same thing."
That journey is just beginning. How will Landing AI solve the problem?
"If I'd already solved it, it would be easier to answer," says Prashanth with a laugh. "That is going to be the secret sauce."
Scary smart tech: 9 real times AI has given us the creeps
Previous and related coverage:
What is AI? Everything you need to know
An executive guide to artificial intelligence, from machine learning and general AI to neural networks.
What is deep learning? Everything you need to know
The lowdown on deep learning: from how it relates to the wider field of machine learning through to how to get started with it.
What is machine learning? Everything you need to know
This guide explains what machine learning is, how it is related to artificial intelligence, how it works and why it matters.
What is cloud computing? Everything you need to know about
An introduction to cloud computing right from the basics up to IaaS and PaaS, hybrid, public, and private cloud.