Amazon AWS analytics director sees analysis spreading much more widely throughout organizations
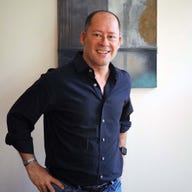
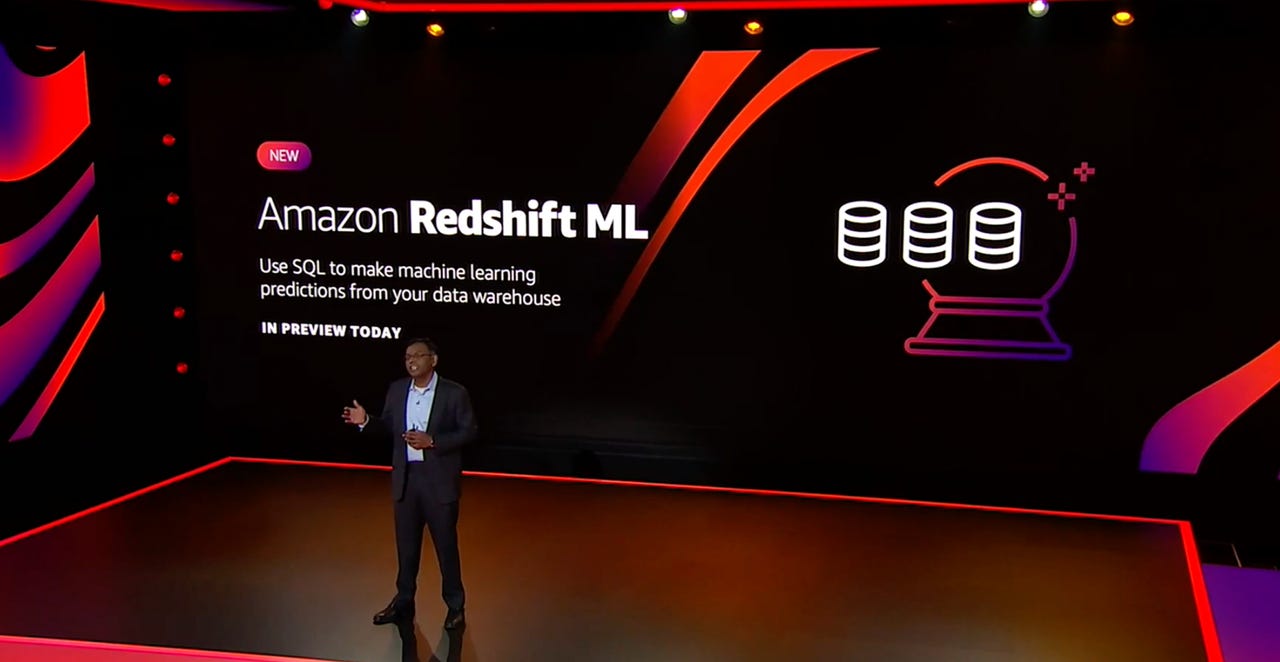
Amazon's vice president of machine learning, Swami Sivasubramanian, Tuesday hosted a keynote to unveil a number of machine learning efforts, during week two of Amazon's re:Invent conference for Amazon Web Services. Among them is RedShift ML, which brings machine learning inference to Amazon's data warehouse service via simple SQL queries that any data analyst can run.
It's time for companies to perhaps have a new "mental model," if you will, of analytics, available much more widely throughout an organization, according to the executive heading up analytics for Amazon's Amazon Web Services.
Companies' parsimonious approach to data, where they had to prune and throw away some information may be a thing of the past, Rahul Pathak, the vice president of analytics for AWS analytics told ZDNet in a video interview Tuesday.
Instead of picking and choose information to cultivate a data warehouse, companies may start to work on much larger data sets that can fit in an Amazon S3 instance, for example.
"One of the big differences we see between what people have done on-premises is that, typically, on-premises have been constrained by cost and scale," said Pathak, "so customers made decisions about what to keep and what not to keep when it came to analytics."
Also: Amazon AWS unveils RedShift ML to 'bring machine learning to more builders'
"What we've tried to do with services in the cloud is to make it cost-effective for customers to really analyze all of their data so that they're not constrained by cost and scale ahead of time."
In addition to resources, permissions were always a limiting factor, noted Pathak, to giving average users access to analytics. That may be less the case once users can tap into the cloud.
"If you think about on-premises appliances, they were typically large, precious things that you controlled access to," said Pathak, "whereas in the cloud, you have the opportunity to really distribute access to powerful analytics to a lot more people and they can do it in a way that's independent without affecting the core analytics operations of the organization."
"In the cloud, you have the opportunity to really distribute access to powerful analytics to a lot more people and they can do it in a way that's independent without affecting the core analytics operations of the organization," says Amazon AWS's head of analytics, Rahul Pathak.
Pathak spoke to ZDNet during week two of AWS's re:Invent annual conference, which is being held virtually this year because of the pandemic.
Earlier in the day, Amazon's vice president of machine learning, Swami Sivasubramanian, outlined several innovations to using analytics on AWS, including QuickSight Q, an extension to AWS's existing QuickSight program. QuickSight Q allows analytics to be integrated into a dashboard so that a business executive can pose analytics-style questions using natural language phrases.
Amazon also said it would integrate machine learning models into its RedShift data warehouse platform, so that developers can run machine learning inference operations without any knowledge of machine learning models. Instead, they need only formulate traditional SQL queries for a database.
Pathak's focus this week is on something called a Lake House architecture, which is a way to merge different data sources such as data lakes and data warehouses.
Customers in the real world have data in purpose-built data stores and analytics services, observed Pathak. As an example, a relational database operation might tie together with an analytics application and go into RedShift, and then be re-directed to a data lake. That involves combining data many times over.
"Real-world customers will be moving data back and forth between data stores," said Pathak.
Also: Amazon unveils Amazon HealthLake, big data store for life sciences
At the heart of the Lake House are tools to automate the integration and transformation of data between these data stores, as well as to abstract away some of the complexity of the different data stores.
A data lake can be built-in S3, and then data can be moved back and forth by Glue, Amazon's ETL service to move and transform data. A new offering announced last week by AWS head Andy Jassy, is Glue Elastic Views. Views lets one move data between repositories in near-real-time. "You can use SQL to define views over multiple sources, and then pick targets to have that view kept up to date in real-time, and we monitor the sources for changes, and propagate that -- think of that as a multi-service, distributed, materialized view service," explained Pathak.
AWS has "invested heavily in governance" to define access control policies for the multiple data stores, he added.
Pathak described the approach of Glue and Glue Elastic Views, and the whole Lake House architecture, as a way to get "the best of both worlds," by which he meant the simplicity and ease of generic tools combined with the specificity of purpose-built applications.
Amazon has an interest in bringing lots of repositories together not just to sell more compute and more storage. During Sivasubramanian's keynote, AWS's head of AI, Matt Wood, described new vertical-market integrations of data. One of them is for life sciences and healthcare, called HealthLake. The idea is to pool all the data that doctors and drug developers need, structured and unstructured, to overcome the time lost by having to constantly consult different repositories.
Ecosystem partners such as Snowflake are still key in all of this, said Pathak. Vendors of ETL tools and data warehouse tools are not outmoded by the AWS tools, he said.
"It's about customer choice, and we offer Glue as a managed service for integration and ETL, but we have deep partnerships with folks like Informatica, SnapLogic that are all in the ETL and integration space," said Pathak.
"Customers will come in with pre-existing relationships," he said, "and so for us interoperability is important and customer choice is also important."
"These are huge segments," added Pathak, referring to things such as data integration tools. "We think there's a lot of opportunity, and a lot of the workloads are still on-premises."
Indeed, Jassy pointed out last week, and Pathak reiterated, that 95% of all workloads in the world are on-premise.
"Customers have investments in existing workloads and logic that's been built in and we want all of that to really just work."
"The opportunity to move all that to the cloud and interoperate with what people have is really the name of the game," said Pathak.