Are Big Data approaches the answer to K12 educational pain points?
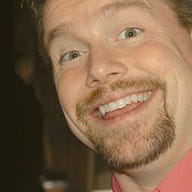
Last month, I attended one of Dell's Social Think Tanks. The topic was Innovation in Education and we had a great discussion on the role of technology in improving the education in America. The Think Tank was big on issues, but turning those issues into actions is overwhelming at best, given the institutionalized challenges that face schools today. As a follow up, Dell held a Google Hangout yesterday with some of the Think Tank participants so we could begin really thinking about concrete solutions. The Hangout recording is embedded below:
Not surprisingly, we had another great talk, but over and over it came up that our current system of high-stakes assessments is broken at best. They do little but penalize students, schools, and teachers and interfere with actual learning in extraordinary ways.
What bothers me most about this, though, is that the technology already exists to assess students' progress on an ongoing basis in a variety of ways, aggregate the data, and drive education in truly personalized and amazing ways. Look at the business intelligence and analytics that businesses apply every day to make decisions and course corrections:
- Walmart knows the items a store needs before its employees do and ships stock automatically
- Credit card companies examine credit histories and card usage patterns to identify high-risk customers or those for whom risk is increasing
- Data from online advertising (click-throughs, conversions, repeated visits, etc.) drive changes to marketing campaigns
- Retailers target consumers with advertisements for products they are likely to buy based on past purchases
- Manufacturers analyze supply chain and production data to improve processes and increase efficiencies
- Insurance companies set rates based on shifting risk profiles built from a variety of data sources
This list could go on for pages. Mid-sized and large companies analyze gigabytes (and even terabytes) of data every week, making both automatic adjustments and providing information to leaders and stakeholders that enables informed strategic planning. Even small businesses regularly use analytic tools to adjust inventory, modify websites, target advertising dollars, and plan for new products.
We're beginning to see the emergence of BI in education - Three years ago, I wrote about an IBM system being used in Mobile, Alabamma, to identify, among other things, students at risk of dropout based on everything from grades to markers of socioeconomic status. But this hasn't managed to scale across states and districts. Too many schools remain focused on high-stakes tests as their sole means of evaluating student achievement. The introduction of student growth models (essentially comparing students to themselves, looking at how they, and the cohorts of which they are members, improve over time) has been an improvement over earlier cross-sectional analyses of NCLB testing data, but in most cases, it still hinges on those same summative assessments that students take every year.
In the same way, adaptive learning software and "Response to Intervention" approaches like those used by Lexia Learning's literacy products represent major steps forward in this area, giving teachers data in real time about individual students and providing scaffolding automatically based on student capabilities. Unfortunately, really useful data like those collected by Lexia, ST Math, and other similar platforms are rarely combined with other sources of student data in ways that would allow us to actually leverage all of the sophisticated BI technology we have at our disposal.
With robust student information systems, learning management systems, instructional software, and powerful data mining tools at our fingertips, "item analysis days" need to become a thing of the past. For those of you unfamiliar with item analysis days (or whatever term your schools or districts use to describe them), teachers in many schools wipe out entire days of professional development examining where last years' students performed poorly on state exams. Then they can shift their curricula to teach to the test better next year...I mean, errr, remap their curricula to better match state standards.
Click here to find out what our information systems should (and could) really be doing for us.
Here's what should really be happening with our vast information systems that we keep building to manage student data:
- Analyze student performance in specific software applications, in written work, and on a variety of assessments to accurately assess student learning styles. This information, along with identified strengths and weaknesses and relevant supporting materials should be provided early and often to teachers so they can stand a chance of meaningfully differentiating instruction.
- Use adaptive learning systems for practice, homework, and ongoing assessment. These systems help students continue to build skills with which they struggle, advance quickly where they excel, and provide useful data to teachers who can again better differentiate instruction among students (no small task with 30 kids in a class, a third of whom may also have special needs).
- Continuously analyze incoming data from these tools and traditional assessments aligned with thoughtful standards to identify deficiencies or potential disabilities as early as possible (and then assess the success of whatever interventions a school puts into place).
- Aggregate the data above with demographic and socioeconomic data to identify students at risk or those who are not growing as expected; subtle shifts in academic progress can be easily detected with the right algorithms, but as class sizes increase, it's quite difficult for teachers to observe and act upon these changes, many of which can be signs of larger issues in a student's life.
- Provide big-picture data to schools and teachers, e.g., subsets of students who are failing to meet particular standards. This sort of information is vital to strategic planning, but, to date, has too often been based on limited snapshots of data (like yearly state exams) and has been used to punish teachers and districts instead of engineer strategic shifts in curriculum and instruction.
- Once all of these elements are in place, BI and analytics tools will be able to meaningfully and objectively identify the professional development needs of specific teachers. If kinesthetic learners in Mrs. Smith's class repeatedly achieve at lower levels than students in her class or other kinesthetic learners in the same grade, then it won't be hard to outline opportunities for her own professional growth and continuing education. Want to implement merit pay without a mutiny? Then let a broad, transparent, robust data set objectively identify the teachers who so clearly stand out from the pack that it brooks no argument.
There are obviously major obstacles in terms of equity, access to resources, and the breadth and depth of available software to make this happen. But until we can take an holistic approach to analyzing and acting on a large body of student data, we'll be spinning our wheels with high-stakes tests that do far more harm than good.
Teachers need to be able to focus on aspects of learning that a computer can't quantify or analyze. How well do students collaborate? How effectively do they communicate? Where are specific learning disabilties or areas of academic and social challenge interfere with their learning? And how can they adjust instruction to best address those challenges? How can gifted students remain motivated and challenged? How can average students find niches in which they shine? And how can struggling learners overcome countless obstacles? Computers will never replace great teachers, but they should free teachers to do their jobs more effectively and ensure that students are more than just data points.