Big Data: Cutting through the bulls**t
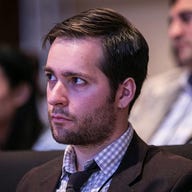
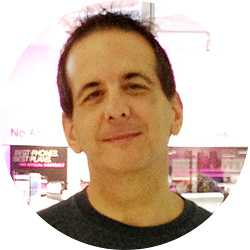
The global market for big data, analytics and business intelligence solutions was worth $22 billion in 2012—and it will keep growing, at a compound annual growth rate of 12.7 percent, through 2017.
That $40 billion estimate, by the way, comes courtesy of the market research firm Frost & Sullivan.
If you've been following coverage here on ZDNet, you'll know that we can be somewhat wary of the term "big data." There's a lot of ambiguity (and hype) wrapped up in the word, and it can be hard to find the nugget of reality trapped inside.
To do that, I run up Frost & Sullivan's Jeff Cotrupe, and brought along Lars Hard, founder and CTO of Expertmaker, a San Francisco-based company that makes artificial intelligence and analytics software.
In a rambling conversation, here's what they had to say on the topic.
Big Data Special Report
Jeff Cotrupe: There's a lot of hype out there. "Big data" is often about "many data." Yes, there's a lot of data, but it's really about different types of data, different structures—39 different areas to consider if you're an enterprise, and another 14 if you're a telecom. They're not only coming at you, but your organization is creating it yourselves.
There are data types, and then there's structured—rows, columns—and unstructured data. We have a nine-part functional model that gets away from this and focuses on what functions and processes you need to take care of. One key area is enterprise search and enterprise knowledge management, because if you're not able to provide great search to find that data at a moment's notice. The magic of enterprise search is that -- we all expect results in sub-seconds, but making that available to everyone with all the sources, not just the web.
Everyone thinks they're sitting on a gold mine. The reality is that there is so much meaningless data. —Lars Hard, CTO, Expertmaker
Lars Hard: There are so many ideas that people carry about the value in their data. Everyone thinks they're sitting on a gold mine. The reality is that there is so much meaningless data. Big data is one thing, big meaningless data is worse. It takes a lot of time to unravel and find what carries meaning.
For that, we need a new generation of tools. Many analytic tools are really not up to the task; many are not so interesting and doing the same things that people have been doing for 10 to 15 years. Disparate sources need to be integrated and meaning found in them. It can be difficult and expensive.
Cotrupe: Enterprises grasp at concepts they've heard. They hear, "Hadoop." Hadoop is wonderful, but it has components to bring the unstructured data into it and allow you to use it as a data warehouse. On its own, it's a distributed file management computing system. You need a good NoSQL database. So they're grabbing at buzzwords.
One of the basic concepts they do get is when we talk about what it really means. One of the precepts here is, with traditional database management systems, there was filtering and summarizing data, because there was only so much storage to go around. A lot of what we're storing might be temporary transactional data. What to keep? What to pitch? What to keep available for real-time analysis? Rather than summarize the data, unlocking the value of it requires going through all of it and not throwing the whole tidal wave at you. So that you're getting the data you really need.
Hard: There's more buzz with this term than anywhere else in the IT industry. I don't really know why.
"Big data" or "predictive analytics" or "machine learning" or "AI"—they are relatively complicated things that we do with computers. A lot of discussion comes down to Hadoop, or whether you're running MapReduce, or...that gets away from the point. The science is easy. It's really an art.
We are being relatively sloppy with the terminology.
I have to tell people that if you configure a certain way, you destroy other interesting things. What kind of provisioning do we have on data? How do we get it into the system? How do we understand what's important? These are relatively structured processes. In the end, I don't want a chart to put up on my wall, because most graphs can never be interpreted by humans anyway, if it's really big and complicated. That makes it abstract. You can't plot on a histogram or monthly development chart like you used to.
We probably need a lot of knowledge transfer. There is an enormous business knowledge if you master data science. Any enterprise that can understand more about their data, there will be huge value.
If you want to dig a huge hole in the ground, you're not using a shovel, right? People are looking at this problem in a way that they have an over-belief in certain technologies that will free them. From a technology perspective, it's an information science. Not every solution is Hadoop and MapReduce. That's not even the question.
So many companies are coming into this space. If you're a large business, and you look at all these companies that will do analytics for you, as a web service, large companies are afraid, because they know that analytics and predictions are what their best people do in their organization. If that's automated, do they want to run on the same algorithms of their competitors? Really? The answer is no.
It's not, "Oh, let's go use the big data now." —Jeff Cotrupe, Frost & Sullivan
We're supposed to get smarter with big data, but it's also about competition. Their intuition is saying that their specific value as a business should be customized to keep the competitive advantage. That complicates the whole discussion. On one side, general services that are low cost and easy to navigate; on the other, deep customization and an R&D department with guys in white coats. That's not viable for most businesses. There has to be something in the middle.
Cotrupe: There's a paradigm here that is interesting. These IT glass walls, where you have to get there and beg them to run a report. When you say "analytics," people think it's that one report you ask them to run for you.
You're empowering business users not to have to beg for information that they need to do their jobs every day. You're putting at people's fingertips—and they don't have to think at all—what they need. It's not, "Oh, let's go use the big data now." If you get it right, it really translates to the business users. We're looking for people who are putting tools at the hands of business users. You don't need it for every application, but real time is very important for functionality.
Featured
We're seeing people that are making tremendous gains. Operators are sitting on a goldmine of data. Instead of just carrying all the traffic and let brands and agencies go after their mobile subscribers, you can equip yourself to sell that access to those relevant subscribers and users you have to match up the right advertising and mobile marketing programs. If you're an operator today, and you're comfortable being the central of your universe all the time, when you get into mobile marketing, you're just one of many. They don't want Operator X's subscribers. They want users ages 16 to 34 who bought electronics in the last 12 months. So capturing your own data and using it lets you become an important part of the chain. It's empowering.
[Telecommunications companies] had to worry for decades about customer proprietary information. So they're very attuned to privacy, yet using information could help tremendously.
In a retail setting, they are aware when a smartphone comes into the store, and can correlate when a sale occurs in the store. The retailer can monetize its Wi-Fi that everybody wants. Take the Gap, for example: they can get a granular level of detail and understand where else the phone went. It revolutionizes the game for retailers. And how do you tie that in with online data? Retailers actually have a "bounce rate" if you leave [the store] quickly. So you can adjust staffing and profitability based on offering the right things at the right time at the right location.
Hard: We are helping e-commerce companies do barcode scanning, which creates new interesting challenges for retailers. Customers go in, scan the barcode using their cellphones and check if they can get deals from anyone else or related products. These forces are coming together. We are driving business away from the store, because I'm in the store, but looking elsewhere. There are so many interesting business models.
Cotrupe: Traditional advertising is still needed. What's more traditional and old-world than billboards? Slap a QR code or something on there and tie it in a mobile marketing campaign or retail program at the point of sale, and old becomes new again. At Whole Foods, people are scanning barcodes to figure out which of the six peanut butters put out like the best. And that may drive how they stock things the next week, and reach the right people with the right offer.
Hard: By integrating those systems, and continuing further into that problem, seeing business as an optimization problem. And then it becomes a task of prediction—can we predict one month ahead? Three months ahead? And that becomes a business advantage in the end, and revenue.