Big-data treasure depends on where you dig for it: Brobst
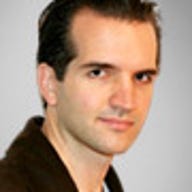
Organisations will find great new value in combining non-transactional data sets with transactional analysis – but must build scalable big-data storage systems rather than assuming that fast but expensive system memory will keep up with exploding data volumes, Teradata chief technology officer Stephen Brobst has warned.
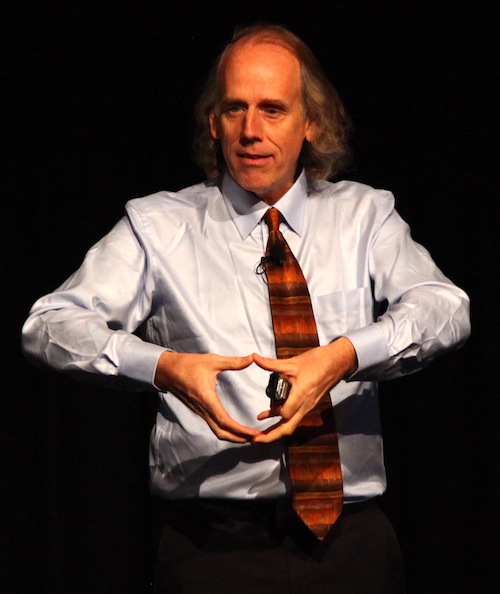
Speaking to attendees at a Teradata analytics event in Melbourne today, Brobst said that while the speed improvements of in-memory processing were obvious, “anybody who talks about putting all the data in memory and big data in the same sentence, has no idea what they’re talking about.”
“From an architectural point of view, of course accessing data from memory is faster than accessing it from a disk drive, but the cost of putting data in memory versus traditional storage is an order of magnitude larger. One of the things you need to look at is how to strike the right balance of performance vs cost.”
The problem is exacerbated because today’s analytics strategies had significantly expanded the scope of the data they’re collecting: whereas companies used to run analytics against transactional history data, companies were now collecting interactional data – records of every click an online shopper makes, for example – as well as detailed geospatial information that is now widely available due to the explosion of ‘spimes’ – objects that are aware of space and time.
“There are all kinds of applications where knowing location is important for how analytics and for how you develop the customer experience,” Brobst said.
“We used to do location analysis based on post codes. But now that geospatial analytics are being done using latitude, longitude and more advanced capabilities, we can start to understand location at a very precise level.”
We used to do location analysis based on post codes. But now that geospatial analytics are being done using latitude, longitude and more advanced capabilities, we can start to understand location at a very precise level."
Brobst described work that Teradata recently undertook with US mobile carrier T-Mobile, which was looking to improve its ability to predict when a customer might churn away from it.
Although certain indicators were available based on analysis of a customer’s interactions with the carrier’s contact centre, T-Mobile found that a far richer source of information was available if the right tools could be applied to analyse mobile-network performance information and correlate it with the other things it knew about its customers.
A high rate of dropped calls from a service, for example, might be a warning sign that a customer was going to switch to a more reliable network; if those dropped calls were all in the same location, that information might not only presage a lost customer but could direct the mobile telco to the best place to put new towers.
“In a lot of telecoms companies, these two different sources of data live in different places,” Brobst explained. “Network engineering has a pile of network data, and the commercial people have a pile of billing data. If we don’t put them together, neither side gets the full value.”
“I might accept a higher level of dropped calls per 1000 if you could guarantee that dropped calls never happen to my high-value subscribers. The idea is to get below the level of the interactions, then combine that with the transactions to get the complete picture.”
Managing that data was far from easy, however: with 3 billion call records alone, T-Mobile had to develop an analysis platform that could churn through massive volumes of information.
Working with Teradata, it used conventional analytics against its structured transactional database, backed with the SQL-MapReduce toolkit and Aster Data data-discovery platform to analyse call-centre interactions that were stored as free-text fields filled with standardised interaction codes.
By combining call-handling records for a particular call, then correlating it with a particular network event, the carrier has been able to get a much better picture of what might tip a customer over the edge – for example, being repeatedly disconnected from calls and then transferred between operators during a customer-support call that keeps them on the line for 20 agonising minutes.
“During a call to the call centre there were many things that happened, and they wanted to build those together,” Brobst explained. “Memo records are built automatically, and with Aster Data we could sessionise them and get a better understanding of what was happening. This kind of analysis actually turns out to be very hard using pure SQL, which is a set-processing language, since sets have no ordering.”
As early analytics trials started delivering new insights, T-Mobile faced the challenge of structuring its expanding data platform. It deployed the Hadoop open-source file system, which is optimised for managing masses of big-data information for analysis.
The Hadoop cluster may be slower than front-line disk or SSD, Brobst said, but it “allows you to capture that data effectively. Once you find value in that data, you can promote it into your warehouse using ETL techniques. You have to do what makes sense based on the size of the data you’re working on.”