Can computers have hunches?
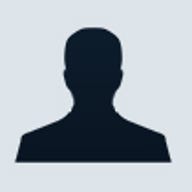

YORKTOWN HEIGHTS, NY—At yesterday's IBM colloquium on cognitive computing, Nobel laureate and Princeton professor Daniel Kahneman addressed the audience as a minority figure—a psychologist among a gathering of computer scientists. But in their effort to build thinking machines technologists have realized a need to better understand thinking humans. And in that endeavor they're deferring to Kahneman.
Here are his words, edited and condensed for clarity.
***
The people here today are interested in how to use computing power for human cognition. And so the task to come is to identify some operations that the human brain performs poorly, and those it does beautifully but which can be improved.
[A distinction] appeared a couple of years ago and it describes two forms of thinking, fast and slow. System 1 is what happens automatically and System 2 is what happens in a controlled fashion, it's slow and effortful. The two systems correspond to two strands in artificial intelligence. There is the problem solving strand, which does things in a logical fashion – the reasoning system. And then there is the strand that goes with scene interpretation.
IBM Colloquium
Let's me begin with slow thinking and System 2. It's characterized with a limited resource we call attention or effort. Attention is needed to perform some tasks and not others. Tasks which involve the switching of attention require a lot of attention. Resistance to distraction requires attention. Only System 2 as far as we know can make decisions and choices. Some examples of what System 2 does are reading a map or making a choice.
The key feature of System 2 is it's limitations. You cannot perform any kind of computation in your head while making a left turn into traffic. We're forced to prioritize and choose.
When we think of ourselves thinking, we tend to think of ourselves as System 2, as in control of our thinking. But the real hero of our thinking I think is System 1, it’s what happens effortlessly.
The list of automatic functions is quite long. It begins with understanding the world, understanding speech, and even uttering speech happens automatically. Associative memory happens automatically. And the idea comes to mind effortlessly. The comprehension of language is effortless, skilled behavior to some extent is effortless, like when a master chess player sees a situation two or three moves would automatically occur to him or her. Emotions and attitudes are automatic. System 2 products we feel ownership of, whereas all of the things that we classify as System 1 are automatic, they happen to us.
Now System 1, the rules of it depart from normal logic. I’ll start with an example. You know about a student, her name is Julie. She is going to graduate this year from college. The one fact I’ll give you is she read fluently at age four. What is her GPA?
Now just about everyone here had a GPA in their head. And likely those GPA's are quite close. How does that happen? How it happens is when I mention that Julie read fluently at age four you had a general impression. She's not at the top but she's certainly near the top. Now I asked her what is her GPA? You also have the scale for GPA. And the GPA that gets chosen has a very simple characteristic. It is as extreme as how precocious she is.
We have a general intensity scale. I could ask you what is the height of a building that is as extreme as Julie's abilities. In that case it leads to completely absurd statistical results. The information that you get from the fact that she read at age four is not very helpful information. But what you find because of the rule of matching is you find completely non-regressive predictions. That seems to be the way we make decisions, non-regressively and by matching.
It's statistically flawed and it's not completely random. If I told you something else about Julie like her fly fishing ability you would not make that inference. But what we also have built into System 1 is we can relate characteristics. GPA and precocious abilities are associatively related. This has many implications, this causes over-confidence but it also allows us to act very quickly, you have the information you're given and you do something with it.
Another instance of very much the same type is shown in this example. Steven is described as meek and tidy with a passion for detail, and people are asked, Is he more likely to be a farmer or a librarian? And most people say librarian. There are about 20 times more male farmers than librarians, but the idea of using the base rate doesn't come up, it's a matching operation.
Obviously System 1 is not a Bayesian System. It is very weak in statistical thinking, but it specializes in causal thinking.
For example there are two cab companies in town, the green company and the blue company. The green company has 85% of the cabs and the blue company the remaining 15%. There was an accident at night, and a witness said he though the cab involved was blue. It was determined that the witness has 80% reliability. People are asked what is the probability that the cab was blue? People say 80%. They go with the witness, even though they know the base rate.
Let me give you a variant on this, same cab companies, same story. However you know 85% of accidents are caused by the green company. As Bayesian problems the problems are completely identical. But the base rate is not ignored in the second problem, people say about fifty-fifty. I have characterized the green cabs, they are reckless madmen. That is a causal property that has something to do with the case at hand. We are following the story of a particular cab and there is no causal correlation, but if the cab driver is likely to be reckless, if there is a stereotype, that information will be used. The system is specialized for causal thinking and very weak for statistical thinking.
Let me give you another example. There were two versions of a questionnaire. One asked, What is the probability that in the next ten years there will be a flood in the US where at least 1000 people will drown? The next was, What is the probability that in the next ten years there will be an earthquake in California that will cause a flood and at least 1000 people will drown? The answers for the second one were that it was much more likely to happen. Because it is a much better story.
Probability is linked to plausibility which is how good is the story. This is about causal connections, matching, and to an extent by social proof. Most of what I think I know I think I know it because I've been told by people I trust and love. That's how people get their political opinions, and that’s why it's so hard to change people's minds. Our beliefs are anchored in social information. If we have beliefs, we believe in the arguments that support those beliefs.
In general what characterizes System 1 is insensitivity to the quality of evidence. It seems to be designed to generate the best story possible based on the available evidence. Wheat you see is all there is. System 1 appears to act as if it were under time pressure, and it wouldn't know what to do if it were given extra time.
So you can think of the automatic system as providing a coherent explanation. We think of a network of ideas and some of the ideas in this network are activated and activity in some inhibits others and this system eventually settles into some kind of equilibrium. And the interpretation is linked to the past, it looks for explanations in the past. It also includes a preparedness for the most likely future – System 1 makes a choice, and we’re not aware that a choice has been made.
The next example is slightly embarrassing. It's something that happened to me and to my wife. We were having dinner with a couple of people and we come back home, and my wife says, “The man in the couple, he is sexy.” Alright. Then the next thing that she says is she says something absolutely bizarre. She says, “He doesn't undress the maid himself.” And I say, “What do you mean?” It turns out she said, “He doesn't underestimate himself.”
It took one word, and possibly a dirty mind, to set a context and to change the interpretation of this. What's interesting is that what I heard had a probability of zero, but I accepted that she had said that, I was completely unaware of any ambiguity.
The interpretation of an event involves a look-back for causes. You hear the sentence, “Jane spent the day in the crowded streets of New York looking at the sights, and when she came back to the hotel she noticed that her wallet was missing.” Now a couple hours later people are more likely to remember that the word “pickpocket” was there than the word “sights.” Because we generate a hypothesis.
What is very interesting about the generalizations that System 1 generates is they tend to be coherent. Associatively coherent and emotionally coherent. Take the sentence that, “Hitler was very nice to children and loved flowers.” People don't like this sentience, this is emotional coherence being violated.
Now one consequence of this is we tend to be overconfident because we suppress ambiguity. Because confidence depends on the quality of the story rather than the quality of the evidence that supports the story.
The main limitation of System 1 is that we live in an oversimplified world. There is a list of mistakes that we associate in general with System 1: over-confidence, anchoring, insensitivity to quality of evidence or base-rate, and my side bias – anything that favors my position I’m more sensitive to.
Let me bring this to a conclusion and switch focus. I have spoken here mainly of the limitations of System 1, but I've neglected the marvels of System 1. The marvel of System 1 is we can develop expertise, and when we develop expertise a lot of these biases and shortcomings can be avoided. System 1 is much faster, and mostly we’re experts at what we're doing. The mistakes happen when we operate outside of our domains of expertise.
Featured
Now this discussion of System 1 and System 2 leads me to the question, What is intelligence? And what would be a intelligent computer look like? Now usually an intelligence test is a test of System 2. But I'd like to ask, how would you test and what is the sense of intelligence you'd test for System 1? If I had to chose something, it's the maintenance and updating of a model of the world. It's from our model of the world that we take in what is happening at the moment. And there seems to be vast difference in people in the quality of that model.
A good test would be to use this model of the world to provide expectations of the world. We can set up target scenarios where we say, If this is going to happen how could it happen? This is something we're capable of doing working off the model that we have.
What would it take to build a computational model that would predict the outcome of this current political crisis? It wouldn't be easy. Predicting the outcome doesn't have a single answer, but some answers are better than others. And what answers are better than others is very important question.
Philip Tetlock became very well know in 2005 when he published a book on political prediction. He's currently working on short term forecasting, it turns out here you can do it much better than anybody thought was possible. He has identified people he calls super forecasters. They absolutely are better than CIA analysts. They combine statistical and causal information in their thinking in novel ways.
So the implications are there are several ways we can think of intelligence agents. We can think of them as an aide, or as a critic. In effect augmenting System 2, it recognizes an anchor, it reminds the individual of ambiguities. And we can think of an intelligence system as an ultimate System 1. And our biggest challenge will be modeling System 1.