Executive's guide to prescriptive analytics
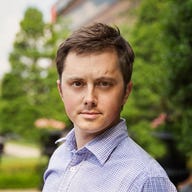
The modern world is inundated with data. It comes to businesses in the form of location data, personal information, cookies, online behavior, buying habits … almost everything internet users do on the web and with their mobile devices ends up generating data of some kind.
One of the biggest ways that businesses can use data is to predict the future through a process called business analytics, in which companies build models of the past, present, and future based on the data they've collected. But knowing what is potentially to come isn't worth a whole lot unless you can figure out what to do with that knowledge, which is where the final stage of business analytics, known as prescriptive analytics, comes in.
Far from being just a forecasting tool, prescriptive analytics aims to go beyond simply describing one potential future: It tugs on the various threads that make up data models to prescribe the best possible course of action.
If your organization gathers data and has yet to commit to a prescriptive analytics program, it's time to consider it. Proper prescriptive programs can save time and money, maximize profit, and ensure that your business is running as smoothly as possible.
SEE: Data analytics: A guide for business leaders (free TechRepublic PDF)
Executive summary (TL;DR)
- What is prescriptive analytics? Prescriptive analytics is the final phase of business analytics. It tweaks predictive analytical models, which predict what will happen if the current course is maintained, in order to achieve the best possible results.
- What kinds of variables does prescriptive analytics track? It's tough to pin this one down to absolutes. Almost any variables that affect business operations can be considered in a prescriptive model. What is important varies across industries, but there are some consistent elements.
- How does prescriptive analytics work? Starting with the predictive model, data scientists make adjustments to various aspects of the model and use multiple types of tech to see how their changes affect the model. Alternatively, they can tweak the model with a specific goal in mind in an attempt to meet a desired conclusion in the most efficient way possible.
- How can prescriptive analytics help businesses succeed? With prescriptive analytics, the game is always the same: optimal performance for minimal cost. Think of a way your organization could improve, then think about what it would take to do that. Prescriptive analytics is the same thing, but with a higher degree of certainty that's more likely to lead to success.
- How does a business get started with prescriptive analytics? Businesses that are already performing data analytics have a leg up on getting to prescriptive analysis. Those that aren't are in for a long, but important, journey that includes hiring new analysts, finding the right prescriptive analytics platform, and building up the descriptive and predictive models that are essential to a good prescription.
What is prescriptive analytics?
Prescriptive analysis is the final stage of a three-part model of business analytics that starts with the sorting of data an organization has collected. Once data has been organized in a way that makes it useful, the first stage of business analytics begins.
Called descriptive analytics, this first stage involves analyzing data to determine the current state of an organization.
Any data that creates a picture of the present can be used to create a descriptive model. Common types of data are customer feedback, budget reports, sales numbers, and other information that allows an analyst to paint a picture of the present using data about the past.
A thorough and complete descriptive model can then be used in predictive analysis to build a model of what's likely to happen in the future if the organization's current course is maintained without any change.
Predictive models are built using machine learning and artificial intelligence, and take into account any potential variables used in a descriptive model. Like a descriptive analysis, a predictive model can be as broadly or as narrowly focused as a business needs it to be.
Predictive models are useful, but they aren't designed to do anything outside of predicting current trends into the future. That's where prescriptive analytics comes in.
A good prescriptive model will account for all potential data points that can alter the course of business, make changes to those variables, and build a model of what's likely to happen if those changes are made.
Prescriptions, like those made by doctors, try to solve a problem in the most efficient way possible. Exercise and a good diet may be enough to resolve a medical problem, but for businesses the best way forward may be adjustments to their supply chain, a streamlined decision-making process, more aggressive sales tactics, or anything else that, in combination with other data points, creates a model of success.
It's worth noting that prescriptive analytics isn't a new concept -- at least according to the IBM team that coined the term in a 2010 article. Prescriptive models were used long before the modern era, but they were limited to the ability of humans to crunch numbers and account for variables.
"Many problems simply involve too many choices or alternatives for a human decision-maker to effectively consider, weigh and trade off," the article said. With the advent of modern machine learning and supercomputing, it's far more practical to build models that are highly accurate and account for practically everything.
SEE: Business analytics: The essentials of data-driven decision-making (ZDNet)
What kinds of variables does prescriptive analytics track?
Business leaders can probably think of a few variables that would go into a prescriptive model of their organization off the top of their heads, but that list is may be only a fraction of all the data points that must be accounted for to build a good prescriptive model.
As mentioned above, prescriptive analysis is all about optimization, so data points that affect optimal business practices are what need to be included. Data used can be either structured or unstructured, so let's take a look at potential variables broken into those two categories.
Structured data
- Customer data
- Sales records
- Shipping records
- Inventory databases
- Billing records
- Timecard records
Structured data is essentially anything that is already in a relational database where there are clearly defined fields into which the data is sorted.
Unstructured data
- Customer feedback
- Web traffic
- Emails
- Invoices
- Paper records
- Documents, both digital and physical
- Photographs
- Audio recordings
- Videos
- Raw data from IoT devices
Unstructured data can essentially be anything that doesn't fall under the structured data category. Chances are your organization has a ton of unstructured data, and lots of it may be important for building a prescriptive model.
How does prescriptive analytics work?
One of the best looks under the hood of prescriptive analytics comes from the 2010 article written by the IBM team referenced above.
In the article, the IBM team defines prescriptive analytics as, "A set of mathematical techniques that computationally determine a set of high-value alternative actions or decisions given a complex set of objectives, requirements, and constraints, with the goal of improving business performance."
If that all sounds like a lot, it's because prescriptive analytics, while simple in concept, requires a lot of work to pull off.
Some of the tools that go into prescriptive analysis include graph analysis, simulations, complex event processing that accounts for additional data, neural networks that combine multiple forms of machine learning, and recommendation engines to model user/customer response.
Additionally, prescriptive analytics has to account for uncertainty in data through the use of heuristics, which is practically a whole other science in and of itself.
Heuristic problem solving involves finding the best course to a practical solution that, while not necessarily the most efficient or ideal, is the best one when uncertainty makes finding the ideal solution impossible.
Given the size and scope of prescriptive models, it makes sense that heuristics are a fundamental part of a good prescription. In many cases, it's simply not possible to find the mathematically optimal solution, so the most practical one that's still an improvement becomes ideal.
Once all variables are accounted for, a model is built and a problem to solve for is determined, a prescriptive analysis can be undertaken. Modern prescriptive analytics tools are often capable of returning results in near real time, making them ideal for changing course on the fly to account for the rapid pace of the modern business world.
How can prescriptive analytics help businesses succeed?
The goal of a prescriptive analysis is always the same: Find the optimal course of action to meet a particular goal.
If you can think of a business need, have found something in your other forms of data analysis that raises questions, or are simply faced with a high degree of uncertainty about the future, there are ways prescriptive analysis can help.
Here are a few examples of prescriptive analysis in action:
DirectBuy, a membership-based consumer buying club (similar to Sam's Club or Costco) used regression techniques and decision tree models to help its member services team develop more effective member retention models and even eliminate several retention programs that were found to be ineffective.
BondIT, a digital investment platform, used IBM's prescriptive platform to build portfolios for fixed-income individuals. Machine learning models have enabled BondIT to custom tailor portfolios to each individual in minutes rather than days and have reduced risk by 30 percent while retaining similar earnings for clients.
FleetPride, which delivers spare parts for commercial vehicles, used prescriptive analytics to streamline its supply chain. Prescriptive models improved inventory management, reduced labor costs, increased profits, and eliminated nearly all errors in shipment packing.
Other potential use cases for prescriptive analytics could be:
- Maximizing airline profits by eliminating over-fueling and creating more efficient flight scheduling
- Improving the cost/benefit ratio of healthcare procedures by finding the best course of action for particular client cases
- Finding the best pricing scheme for items in a retail store
- Optimizing product layout on shelves in a store
- Determining the most efficient way to schedule shifts in a 24-hour factory
- Finding the optimum source for raw materials
The ways in which prescriptive analysis can benefit an organization are incredibly varied, and it's hard to say precisely how a prescriptive analytics program could help a particular business succeed.
From a general standpoint, as mentioned above, prescriptive analytics aims to find the optimal course of action to meet a specific business goal. Whether that goal is something general, like improving profits, or something specific, like determining the optimal spot to drill a well, a good prescriptive analytics program has the potential to transform a business and make the future easier to see.
SEE: Zebra Technologies buys prescriptive analytics software firm Profitect (ZDNet)
How does a business get started with prescriptive analytics?
If your organization has already developed an analytics program of some sort, congratulations: You're one step closer to prescriptive analytics. Having the groundwork in place for descriptive and predictive analysis can save you a lot of time and money.
Organizations that are new to business data analytics, on the other hand, have a lot of work cut out for them.
Step 0: Data
If your organization doesn't have a good handle on its data, or if you haven't been collecting it in any meaningful way, you'll need to start here.
All the data you think may be relevant should be gathered and organized. This includes both structured and unstructured data.
Step 1: State a goal
Like any business transformation initiative, it's nearly impossible to start working toward prescriptive analytics without sitting down and stating a goal. As mentioned before, this can be a particular goal or something broader with a larger impact on the entire organization.
Regardless of how you approach a prescriptive program, it's essential to set a goal so you know what you're working with.
Step 2: Outline
Once you know where you want to get to with a prescriptive analytics program, it's time to figure out what you need to do to get there. This step is going to be the one you spend the most time in, at least from a planning perspective, so make sure you don't skip anything essential.
Things you need to determine during this step include:
- Defining the particular problem you think you have
- Identifying what kinds of data you'll need to focus on
- Determining the kinds of skills your analytics team will need
- Deciding who is responsible for the overall project
- Developing a timeline for the project
- Choosing the software you'll need to perform your analytics
Analytics software comes in a lot of flavors, and the one you choose can have a huge impact on what your team is going to need to do. Different types of platforms are available, and they largely fall into three categories: prepackaged software, modeling platforms, and solvers.
Prepackaged software is typically built to support a particular kind of problem and industry and includes products like:
- River Logic, which is a general use, low-code analytics suite
- Profitect, which focuses on retail applications of prescriptive analytics
- Angoss, which has a focus on marketing
- Rockwell Automation, which is geared toward optimizing maintenance needs
The other two forms of software, modeling platforms and solvers, tend to be used in tandem. Modeling platforms, according to River Logic, are used to build mathematical models that define problems. Solvers, on the other hand, are used to develop solutions based on the problem defined in a modeling platform.
There are dozens of both types of platforms. Some of the leaders in these types of software include IBM, NGDATA, River Logic, FICO, and SAS.
Modeling and solving software tends to be more adaptable, but it requires a lot more analytics proficiency to use. If you're going to go this route be prepared to spend more money—but you should end up with a solution more tailored to your organization's unique needs.
Step 3: Test
Once you have a team in place, have selected a platform, and know what you want to accomplish, it's time to start the real analytics work.
The first thing your analytics team should do is build a proof-of-concept that will help you understand if your project is feasible.
Once the proof has been worked out, you can use the same basic model to build a small-scale implementation with real data. Perform small-scale tests to see what kind of results you get, make adjustments, and then test at larger and larger scales.
At this point, it's advisable to scale up to full strength, but work on building a solid predictive model you can use as your baseline for performing prescriptive experiments.
Once all applicable data has been added, prescriptive formulae and algorithms have been built, and everything is running reliably, it's time for the final step: actual prescriptive analysis.
Feedback from a working prescriptive system can be quick, so be sure your organization is ready to start implementing what your models suggest immediately. Prescriptive models that aren't acted upon are just as useless as ignoring a doctor's orders, so don't let your plans languish by not enacting them immediately.
This whole process can take time. Months or even years can be spent getting things right before receiving actionable prescriptive analysis. Don't let that stop you from investing, though. Prescriptive analytics is fast becoming a major contributor to success in the modern business world.
Why guess, make mistakes, and start again at square one when you can use the advanced machine learning and big data crunching software available on the market today? There may be big startup costs involved, but the well-known adage "You have to spend money to make money" applies perfectly to prescriptive analytics.
Also see
- Prescriptive analytics: A cheat sheet (TechRepublic)
- Prescriptive analytics: An insider's guide (free PDF) (TechRepublic)
- Feature comparison: Data analytics software and services (Tech Pro Research)